A Data-Driven Approach for Process Optimization of Metallic Additive Manufacturing under Uncertainty
Journal of manufacturing science and engineering(2019)
摘要
The presence of various uncertainty sources in metal-based additive manufacturing (AM) process prevents producing AM products with consistently high quality. Using electron beam melting (EBM) of Ti-6Al-4V as an example, this paper presents a data-driven framework for process parameters optimization using physics-informed computer simulation models. The goal is to identify a robust manufacturing condition that allows us to constantly obtain equiaxed materials microstructures under uncertainty. To overcome the computational challenge in the robust design optimization under uncertainty, a two-level data-driven surrogate model is constructed based on the simulation data of a validated high-fidelity multiphysics AM simulation model. The robust design result, indicating a combination of low preheating temperature, low beam power, and intermediate scanning speed, was acquired enabling the repetitive production of equiaxed structure products as demonstrated by physics-based simulations. Global sensitivity analysis at the optimal design point indicates that among the studied six noise factors, specific heat capacity and grain growth activation energy have the largest impact on the microstructure variation. Through this exemplar process optimization, the current study also demonstrates the promising potential of the presented approach in facilitating other complicate AM process optimizations, such as robust designs in terms of porosity control or direct mechanical property control.
更多查看译文
关键词
additive manufacturing,uncertainty,data-driven,robust design
AI 理解论文
溯源树
样例
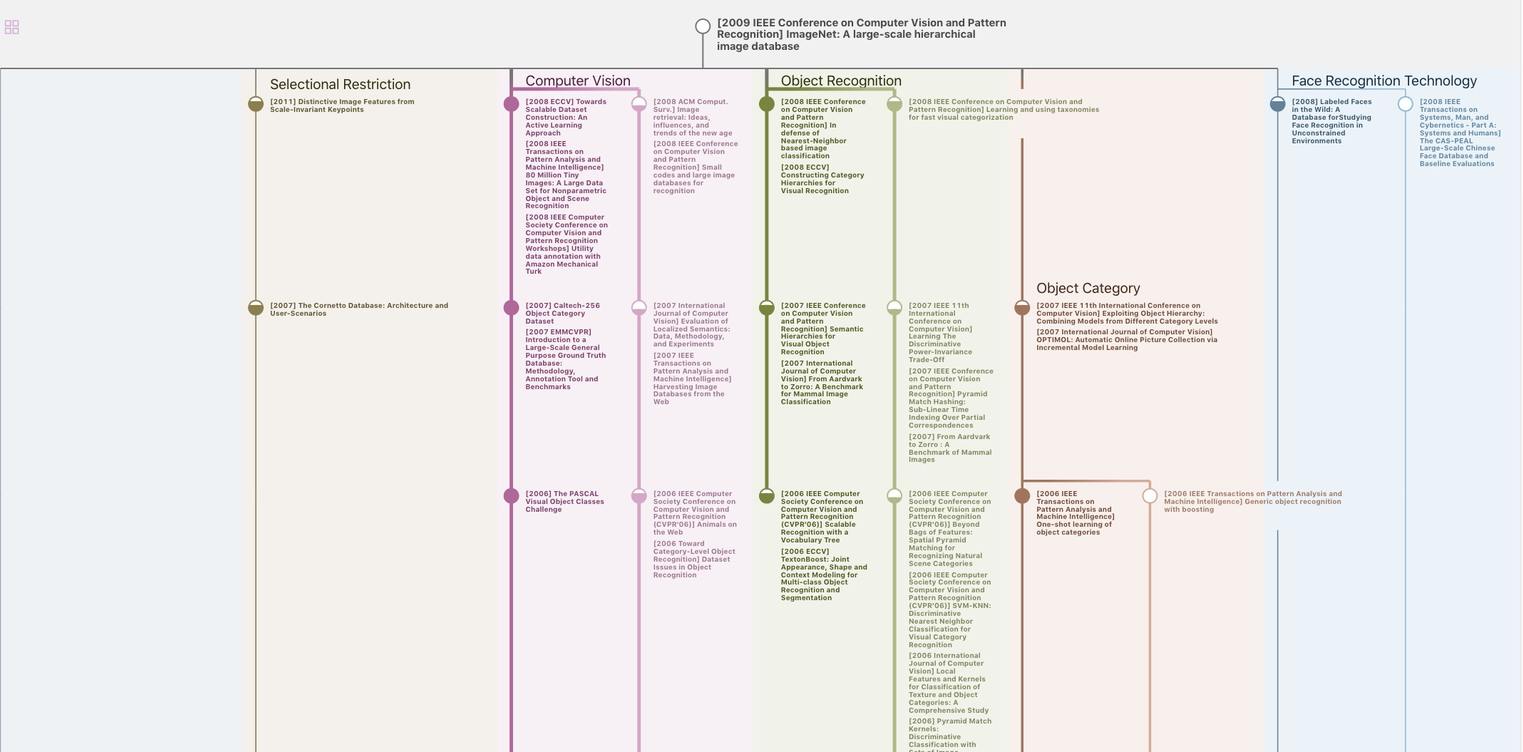
生成溯源树,研究论文发展脉络
Chat Paper
正在生成论文摘要