Use Of Machine Learning To Identify Relevant Research Publications In Clinical Oncology.
JOURNAL OF CLINICAL ONCOLOGY(2019)
摘要
6558 Background: Finding high-quality science to support decisions for individual patients is challenging. Common approaches to assess clinical literature quality and relevance rely on bibliometrics or expert knowledge. We describe a method to automatically identify clinically relevant, high-quality scientific citations using abstract content. Methods: We used machine learning trained on text from PubMed papers cited in 3 expert resources: NCCN, NCI-PDQ, and Hemonc.org. Balanced training data included text cited in at least two sources to form an “on topic” set (i.e., relevant and high quality), and an “off-topic” set, not cited in any of the above 3 sources. The off-topic set was published in lower ranked journals, using a citation-based score. Articles were part of an Oncology Clinical Trial corpus generated using a standard PubMed query. We used a gradient boosted-tree approach with a binary logistic supervised learning classification. Briefly, 988 texts were processed to produce a term frequency-inverse document frequency (tf-idf) n-gram representation of both the training and the test set (70/30 split). Ideal parameters were determined using 1000-fold cross validation. Results: Our model classified papers in the test set with 0.93 accuracy (95% CI (0.09:0.96) p ≤ 0.0001), with sensitivity 0.95 and specificity 0.91. Some false positives contained language considered clinically relevant that may have been missed or not yet included in expert resources. False negatives revealed a potential bias towards chemotherapy-focused research over radiation therapy or surgical approaches. Conclusions: Machine learning can be used to automatically identify relevant clinical publications from biographic databases, without relying on expert curation or bibliometric methods. The use of machine learning to identify relevant publications may reduce the time clinicians spend finding pertinent evidence for a patient. This approach is generalizable to cases where a corpus of high-quality publications that can serve as a training set exists or cases where document metadata is unreliable, as is the case of “grey” literature within oncology and beyond to other diseases. Future work will extend this approach and may integrate it into oncology clinical decision-support tools.
更多查看译文
AI 理解论文
溯源树
样例
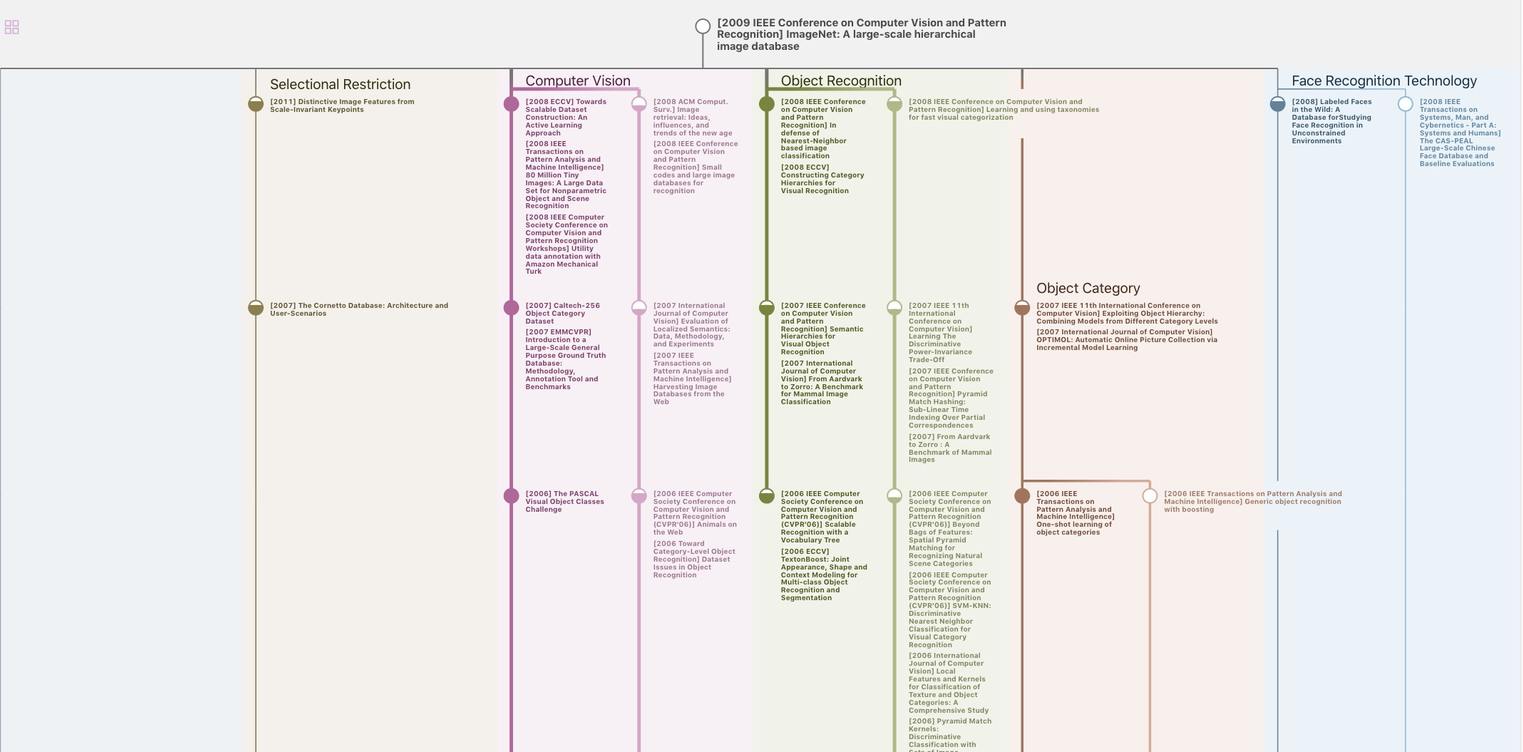
生成溯源树,研究论文发展脉络
Chat Paper
正在生成论文摘要