Natural and Realistic Single Image Super-Resolution with Explicit Natural Manifold Discrimination
2019 IEEE/CVF CONFERENCE ON COMPUTER VISION AND PATTERN RECOGNITION (CVPR 2019)(2019)
摘要
Recently, many convolutional neural networks for single image super-resolution (SISR) have been proposed, which focus on reconstructing the high-resolution images in terms of objective distortion measures. However, the networks trained with objective loss functions generally fail to reconstruct the realistic fine textures and details that are essential for better perceptual quality. Recovering the realistic details remains a challenging problem, and only a few works have been proposed which aim at increasing the perceptual quality by generating enhanced textures. However, the generated fake details often make undesirable artifacts and the overall image looks somewhat unnatural. Therefore, in this paper, we present a new approach to reconstructing realistic super-resolved images with high perceptual quality, while maintaining the naturalness of the result. In particular, we focus on the domain prior properties of SISR problem. Specifically, we define the naturalness prior in the low-level domain and constrain the output image in the natural manifold, which eventually generates more natural and realistic images. Our results show better naturalness compared to the recent super-resolution algorithms including perception-oriented ones.
更多查看译文
关键词
Low-level Vision,Deep Learning
AI 理解论文
溯源树
样例
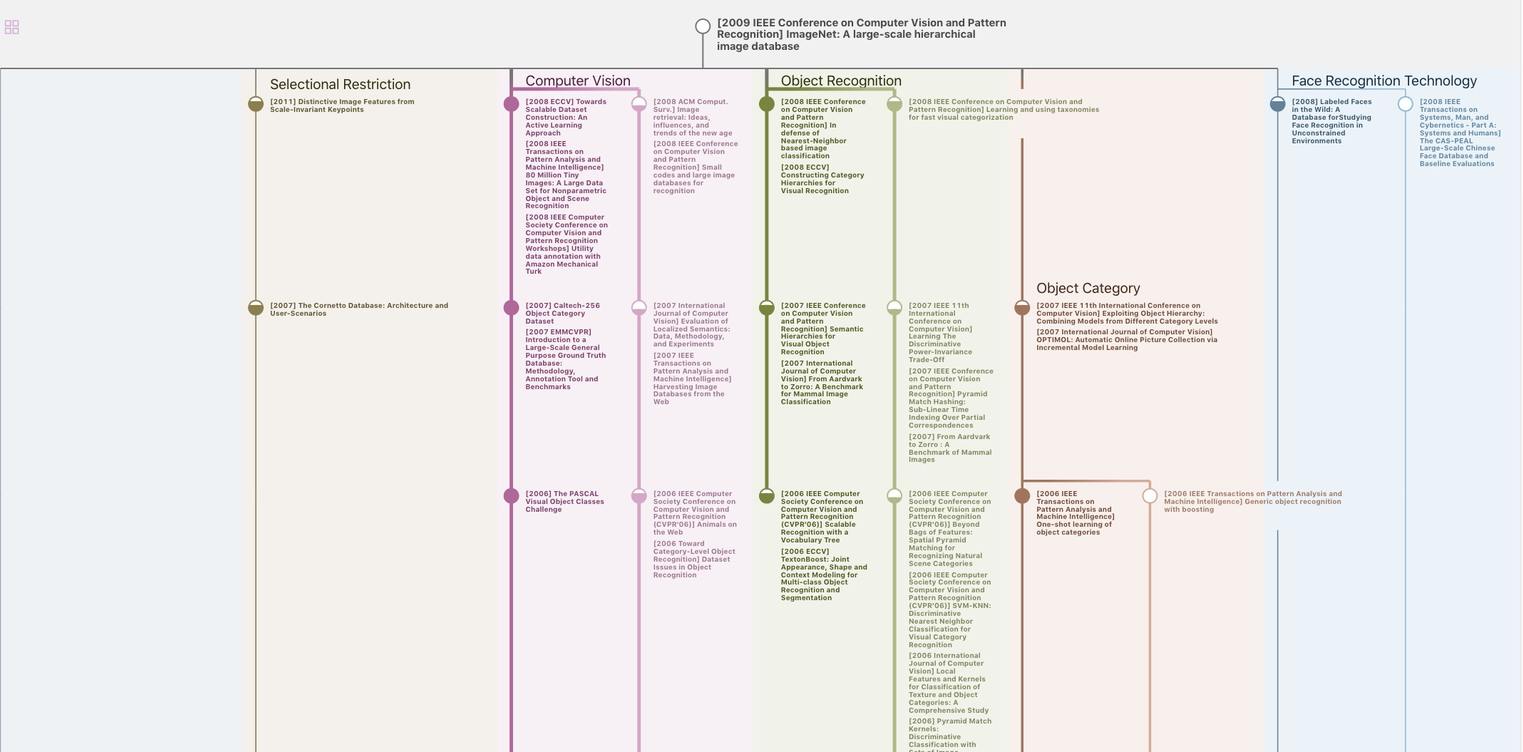
生成溯源树,研究论文发展脉络
Chat Paper
正在生成论文摘要