Purposeful Random Attacks on Networks --- Forecasting Node Ranking Not Based on Network Structure
Acta Physica Polonica B(2019)
摘要
We propose the conception of purposeful random attacks, which can greatly save attacking costs but achieve comparable effects to targeted attacks, based on the hidden degree centrality (HDC) defined according to node features in the one-dimensional circle model of network hidden metric spaces. The macro-matching degree is proposed to research attacking effects. Results show that when the optional node set for attacks is selected as nodes ranked in the top beta% according to HDC, the macro-matching degree will be > 80% with beta = 0.5. The smaller the value of beta, the higher the value of the matching degree, showing that random attacks on the optional node set would be performed at most of those really important nodes. Further, the effect of the purposeful random attack becomes better with the growth of parameter alpha in the circle model. However, after alpha grows to a fixed value, it would have no influence on the attack effectiveness. Jump phenomena presented by changing curves of the macro-matching degree with parameter gamma, another parameter in the circle model, show that networks should be divided into two groups for gamma > 2.5 or gamma < 2.5, and in any group, the effect of the purposeful random attack becomes worse with the growth of gamma.
更多查看译文
AI 理解论文
溯源树
样例
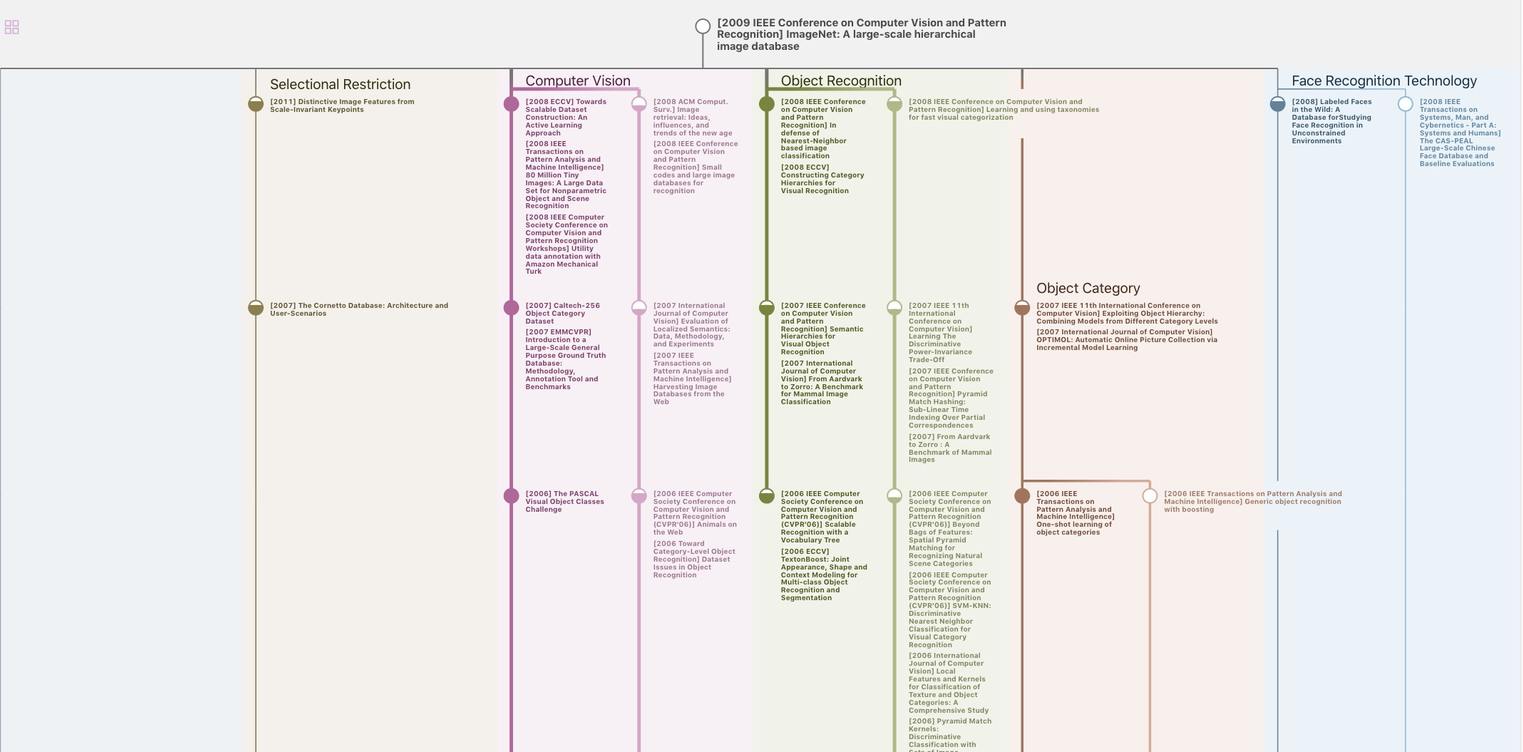
生成溯源树,研究论文发展脉络
Chat Paper
正在生成论文摘要