Kernel quadrature with DPPs.
ADVANCES IN NEURAL INFORMATION PROCESSING SYSTEMS 32 (NIPS 2019)(2019)
摘要
We study quadrature rules for functions from an RKHS, using nodes sampled from a determinantal point process (DPP). DPPs are parametrized by a kernel, and we use a truncated and saturated version of the RKHS kernel. This link between the two kernels, along with DPP machinery, leads to relatively tight bounds on the quadrature error, that depends on the spectrum of the RKHS kernel. Finally, we experimentally compare DPPs to existing kernel-based quadratures such as herding, Bayesian quadrature, or leverage score sampling. Numerical results confirm the interest of DPPs, and even suggest faster rates than our bounds in particular cases.
更多查看译文
关键词
quadrature rules
AI 理解论文
溯源树
样例
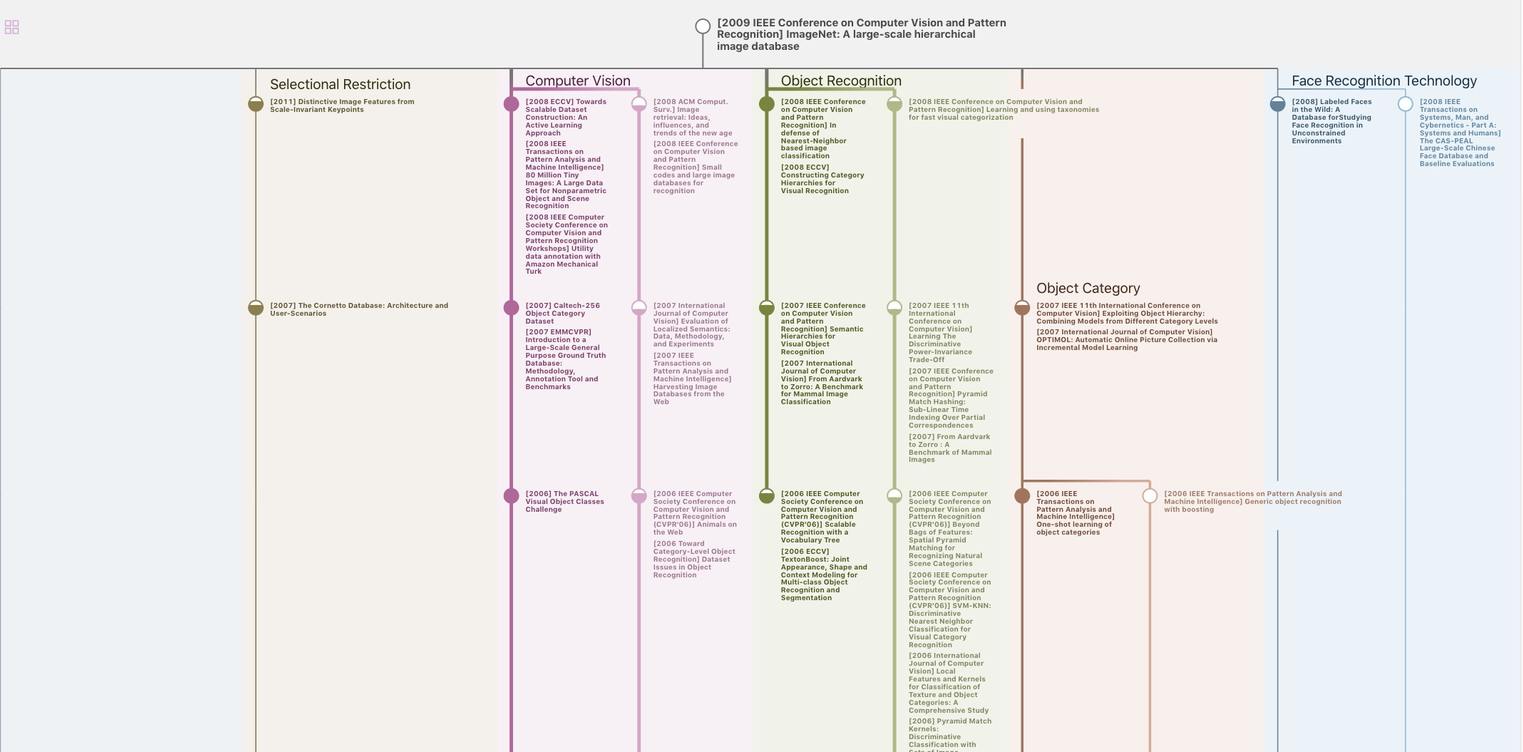
生成溯源树,研究论文发展脉络
Chat Paper
正在生成论文摘要