Hierarchical Document Encoder for Parallel Corpus Mining.
FOURTH CONFERENCE ON MACHINE TRANSLATION (WMT 2019), VOL 1: RESEARCH PAPERS(2019)
摘要
We explore using multilingual document embeddings for nearest neighbor mining of parallel data. Three document-level representations are investigated: (i) document embeddings generated by simply averaging multilingual sentence embeddings; (ii) a neural bagof-words (BoW) document encoding model; (iii) a hierarchical multilingual document encoder (HiDE) that builds on our sentence-level model. The results show document embeddings derived from sentence-level averaging are surprisingly effective for clean datasets, but suggest models trained hierarchically at the document-level are more effective on noisy data. Analysis experiments demonstrate our hierarchical models are very robust to variations in the underlying sentence embedding quality. Using document embeddings trained with HiDE achieves state-of-the-art performance on United Nations (UN) parallel document mining, 94.9% P@1(1) for en-fr and 97.3% P@1 for en-es.
更多查看译文
AI 理解论文
溯源树
样例
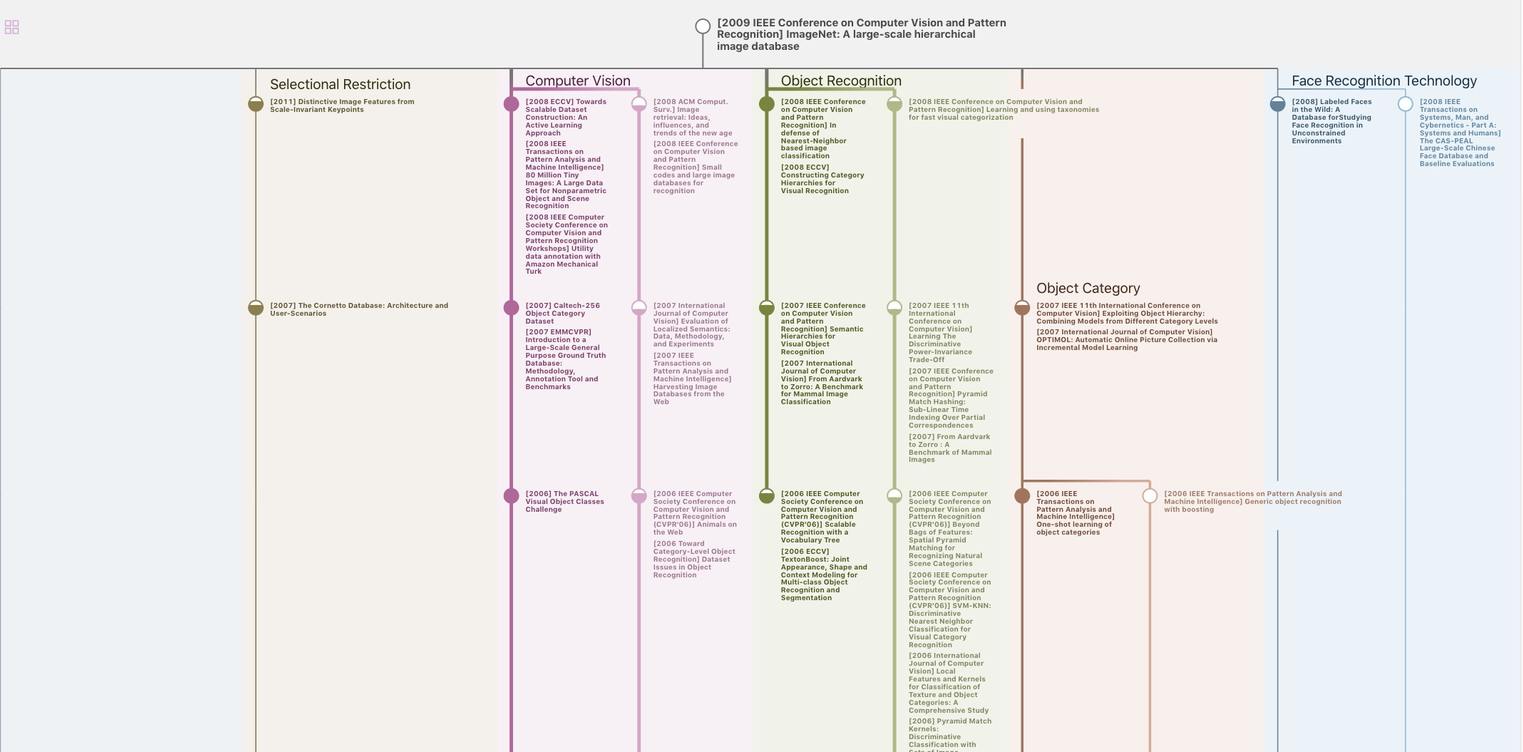
生成溯源树,研究论文发展脉络
Chat Paper
正在生成论文摘要