Exploiting The Spam Correlations In Scalable Online Social Spam Detection
CLOUD COMPUTING - CLOUD 2019(2019)
摘要
The huge amount of social spam from large-scale social networks has been a common phenomenon in the contemporary world. The majority of former research focused on improving the efficiency of identifying social spam from a limited size of data in the algorithm side, however, few of them target on the data correlations among large-scale distributed social spam and utilize the benefits from the system side. In this paper, we propose a new scalable system, named SpamHunter, which can utilize the spam correlations from distributed data sources to enhance the performance of large-scale social spam detection. It identifies the correlated social spam from various distributed servers/sources through DHT-based hierarchical functional trees. These functional trees act as bridges among data servers/sources to aggregate, exchange, and communicate the updated and newly emerging social spam with each other. Furthermore, by processing the online social logs instantly, it allows online streaming data to be processed in a distributed manner, which reduces the online detection latency and avoids the inefficiency of outdated spam posts. Our experimental results with real-world social logs demonstrate that SpamHunter reaches 95% F1 score in the spam detection, achieves high efficiency in scaling to a large amount of data servers with low latency.
更多查看译文
关键词
Social spam detection, DHT-based overlay
AI 理解论文
溯源树
样例
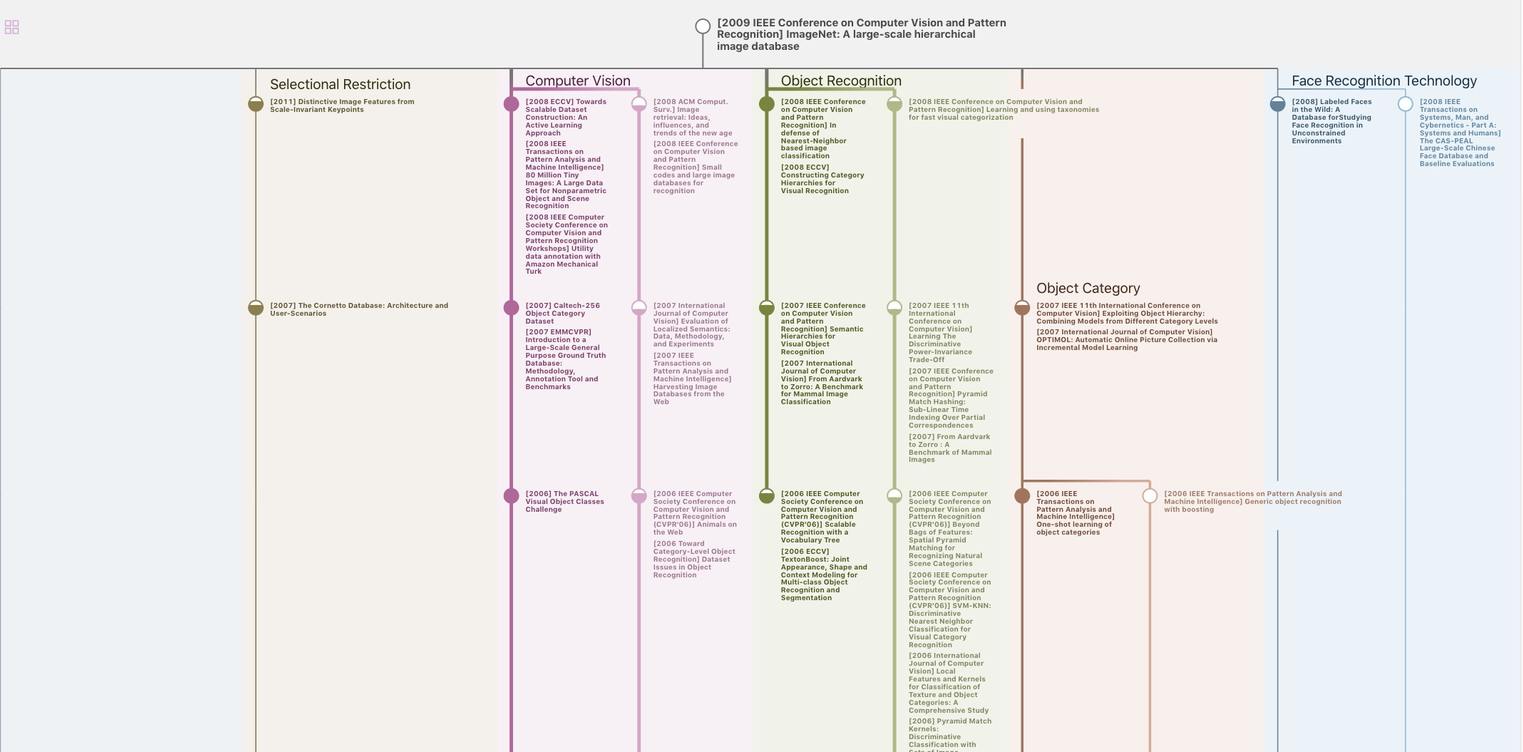
生成溯源树,研究论文发展脉络
Chat Paper
正在生成论文摘要