Robust Non-Bayesian Social Learning
Proceedings of the 2019 ACM Conference on Economics and Computation(2019)
摘要
We study non-Bayesian social learning in large networks and binary state space. Agents who are located in a network receive conditionally i.i.d. signals over the state. We refer to the initial distribution of signals as the information structure. In each step, all agents aggregate their belief with the beliefs of their neighbors according to some non-Bayesian rule. We refer to the aggregation rule as the learning dynamic. We say that a dynamic leads to learning if the beliefs of all agents converge to the correct state with a probability that approaches one in an increasing sequence of large networks. We say that a class of information structures p is learnable if there exists a learning dynamic that leads to learning for all information structures in p. Namely, there exists a single learning dynamic that robustly leads to learning for all possible information structures. We provide a necessary and sufficient characterization of learnable classes of information structures. Whenever learning is possible in a class p it is also possible via a virtually additive learning dynamic, where players map beliefs to virtual values and in each period they simply sum up all neighbors' virtual values to deduce their new belief. In addition, we relax the common prior assumption and provide a sufficient condition for learning in the absence of a common prior.
更多查看译文
关键词
information aggregation, learning in networks, non-bayesian learning, social networks
AI 理解论文
溯源树
样例
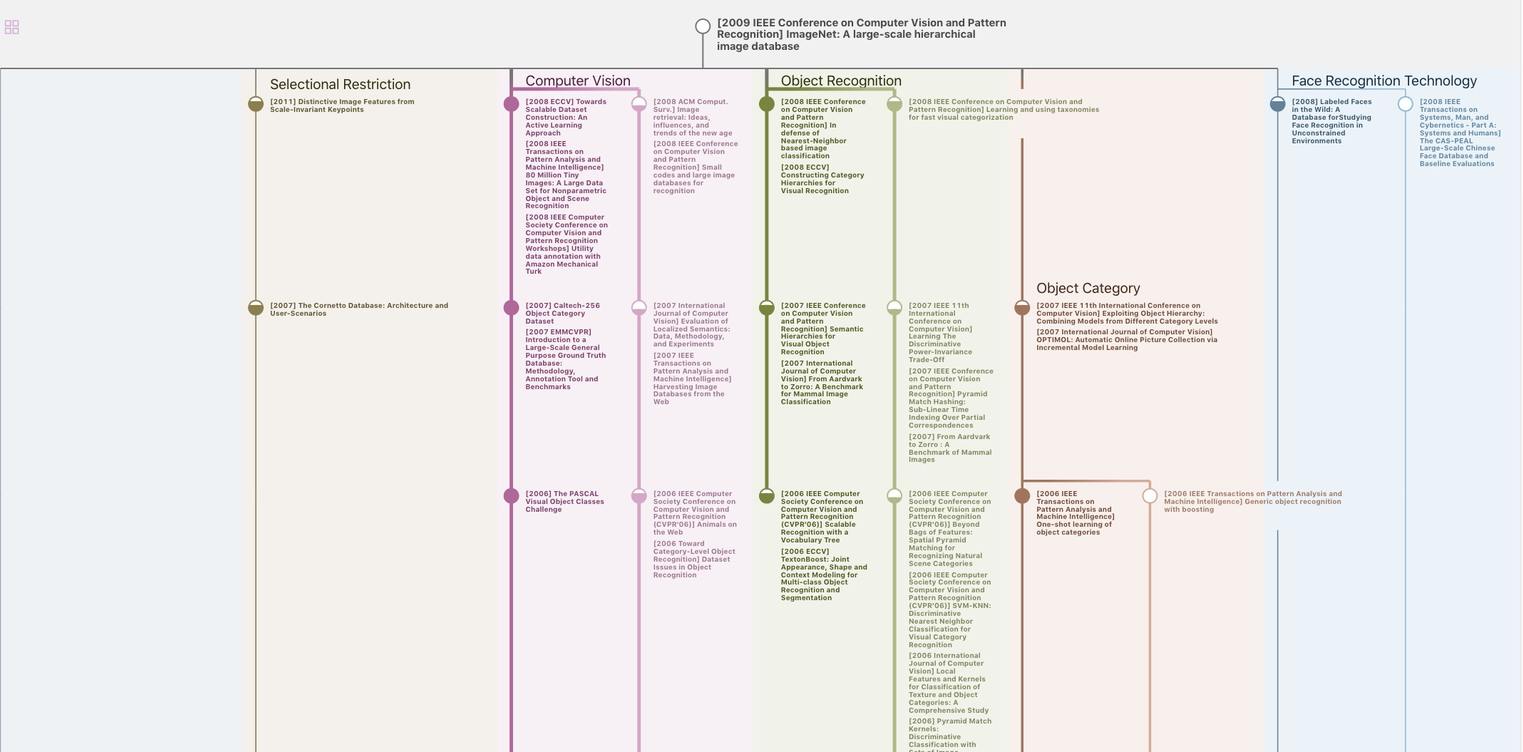
生成溯源树,研究论文发展脉络
Chat Paper
正在生成论文摘要