Impact of baseline covariate imbalance on bias in treatment effect estimation in cluster randomized trials: Race as an example
Contemporary Clinical Trials(2020)
摘要
Individual-level baseline covariate imbalance could happen more frequently in cluster randomized trials, and may influence the observed treatment effect. Using computer and real-data simulations, this paper quantifies the extent and impact of covariate imbalance on the estimated treatment effect for both continuous and binary outcomes, and relates it to the degree of imbalance for different numbers of clusters, cluster sizes, and covariate intraclass correlation coefficients. We focused on the impact of race as a covariate, given the emphasis of regulatory and funding bodies on understanding the influence of demographic characteristics on treatment effectiveness. We found that bias in the treatment effect is proportional to both the degree of baseline covariate imbalance and the covariate effect size. Larger numbers of clusters result in lower covariate imbalance, and increasing cluster size is less effective in reducing imbalance compared to increasing the number of clusters. Models adjusted for important baseline confounders are superior to unadjusted models for minimizing bias in both model-based simulations and an innovative simulation based on real clinical trial data. Higher outcome intraclass correlation coefficients did not affect bias but resulted in greater variance in treatment estimates.
更多查看译文
关键词
(MeSH terms): Bias,Cluster analysis,Multivariable analysis,Randomized controlled trials as topic,Research design
AI 理解论文
溯源树
样例
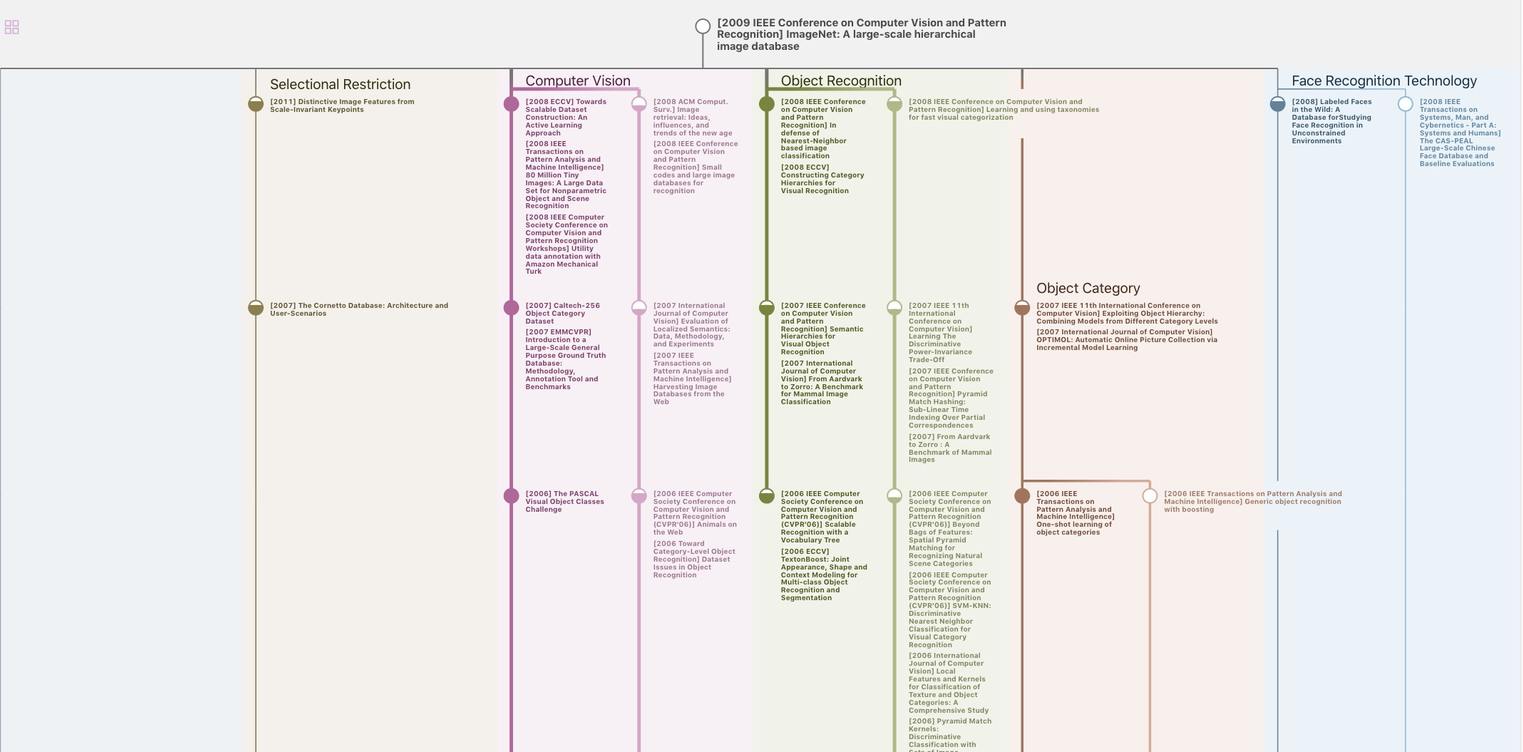
生成溯源树,研究论文发展脉络
Chat Paper
正在生成论文摘要