Greedy Algorithms for Sparse Sensor Placement via Deep Learning
arxiv(2018)
摘要
We consider the exploration problem: an agent equipped with a depth sensor must map out a previously unknown environment using as few sensor measurements as possible. We propose an approach based on supervised learning of a greedy algorithm. We provide a bound on the optimality of the greedy algorithm using submodularity theory. Using a level set representation, we train a convolutional neural network to determine vantage points that maximize visibility. We show that this method drastically reduces the on-line computational cost and determines a small set of vantage points that solve the problem. This enables us to efficiently produce highly-resolved and topologically accurate maps of complex 3D environments. Unlike traditional next-best-view and frontier-based strategies, the proposed method accounts for geometric priors while evaluating potential vantage points. While existing deep learning approaches focus on obstacle avoidance and local navigation, our method aims at finding near-optimal solutions to the more global exploration problem. We present realistic simulations on 2D and 3D urban environments.
更多查看译文
AI 理解论文
溯源树
样例
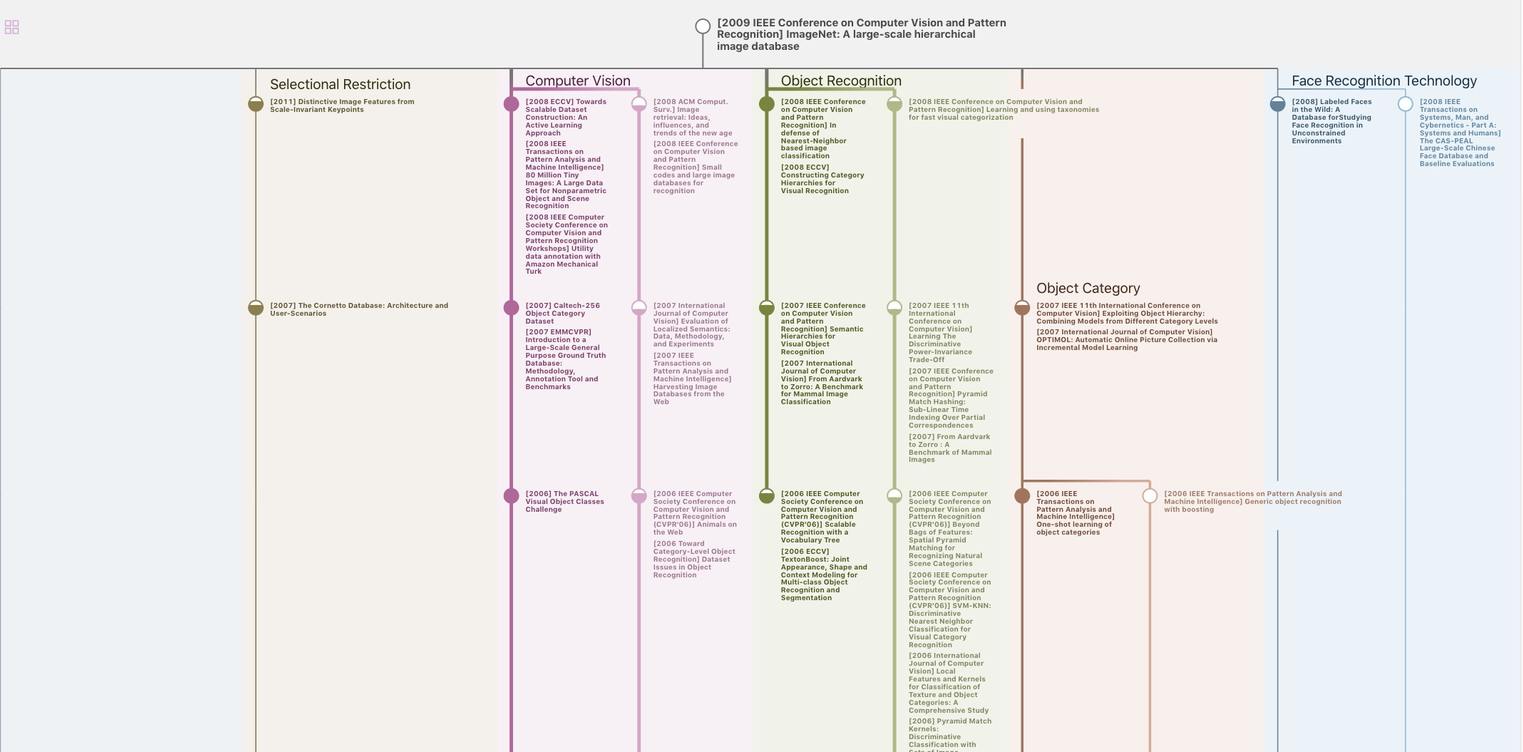
生成溯源树,研究论文发展脉络
Chat Paper
正在生成论文摘要