Demonstration-Guided Deep Reinforcement Learning of Control Policies for Dexterous Human-Robot Interaction
2019 INTERNATIONAL CONFERENCE ON ROBOTICS AND AUTOMATION (ICRA)(2019)
摘要
In this paper, we propose a method for training control policies for human-robot interactions such as handshakes or hand claps via Deep Reinforcement Learning. The policy controls a humanoid Shadow Dexterous Hand, attached to a robot arm. We propose a parameterizable multi-objective reward function that allows learning of a variety of interactions without changing the reward structure. The parameters of the reward function are estimated directly from motion capture data of human-human interactions in order to produce policies that are perceived as being natural and human-like by observers. We evaluate our method on three significantly different hand interactions: handshake, hand clap and finger touch. We provide detailed analysis of the proposed reward function and the resulting policies and conduct a large-scale user study, indicating that our policy produces natural looking motions.
更多查看译文
关键词
handshake,hand clap,finger touch,training control policies,human-robot interactions,hand claps,humanoid Shadow Dexterous Hand,robot arm,multiobjective reward function,reward structure,motion capture data,human-human interactions,hand interactions,dexterous human-robot interaction,demonstration-guided deep reinforcement learning
AI 理解论文
溯源树
样例
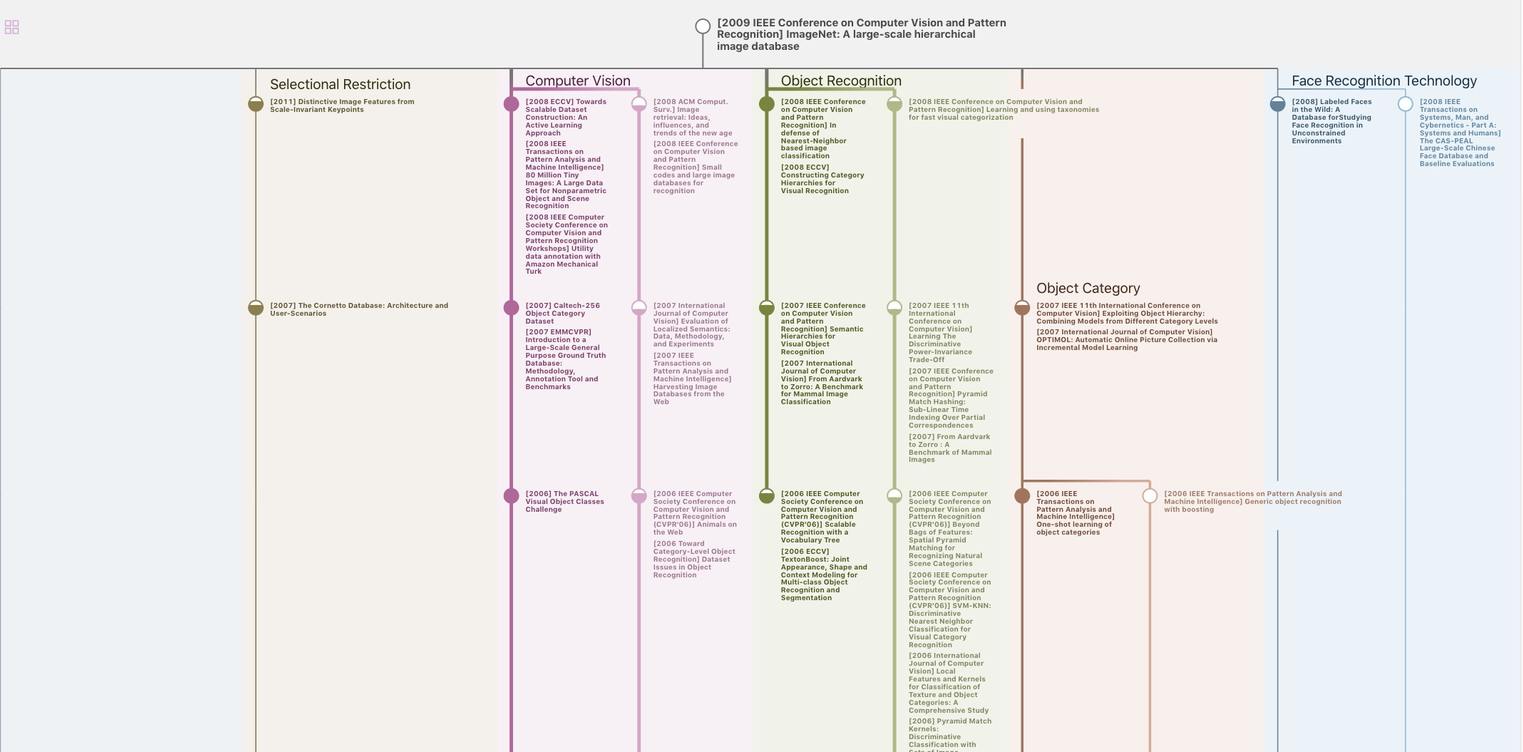
生成溯源树,研究论文发展脉络
Chat Paper
正在生成论文摘要