Privacy-Preserving Paralinguistic Tasks
2019 IEEE INTERNATIONAL CONFERENCE ON ACOUSTICS, SPEECH AND SIGNAL PROCESSING (ICASSP)(2019)
摘要
Speech is one of the primary means of communication for humans. It can be viewed as a carrier for information on several levels as it conveys not only the meaning and intention predetermined by a speaker, but also paralinguistic and extra-linguistic information about the speaker's age, gender, personality, emotional state, health state and affect. This makes it a particularly sensitive biometric, that should be protected. In this work we intent to explore how Leveled Homomorphic Encryption can be combined with a Neural Network to create a privacy-preserving machine learning framework for speech-based health-related tasks. In particular, we will apply this framework to the detection and assessment of a Cold, Depression and Parkinson's Disease. Moreover, we will show how using a Quantized Neural Network, with discretized weights, allows us to apply a Leveled Homomorphic Encryption technique called batching that can be utilized to reduce the effective computational cost of this framework.
更多查看译文
关键词
Privacy, Machine Learning, Homomorphic Encryption, Speech, Health
AI 理解论文
溯源树
样例
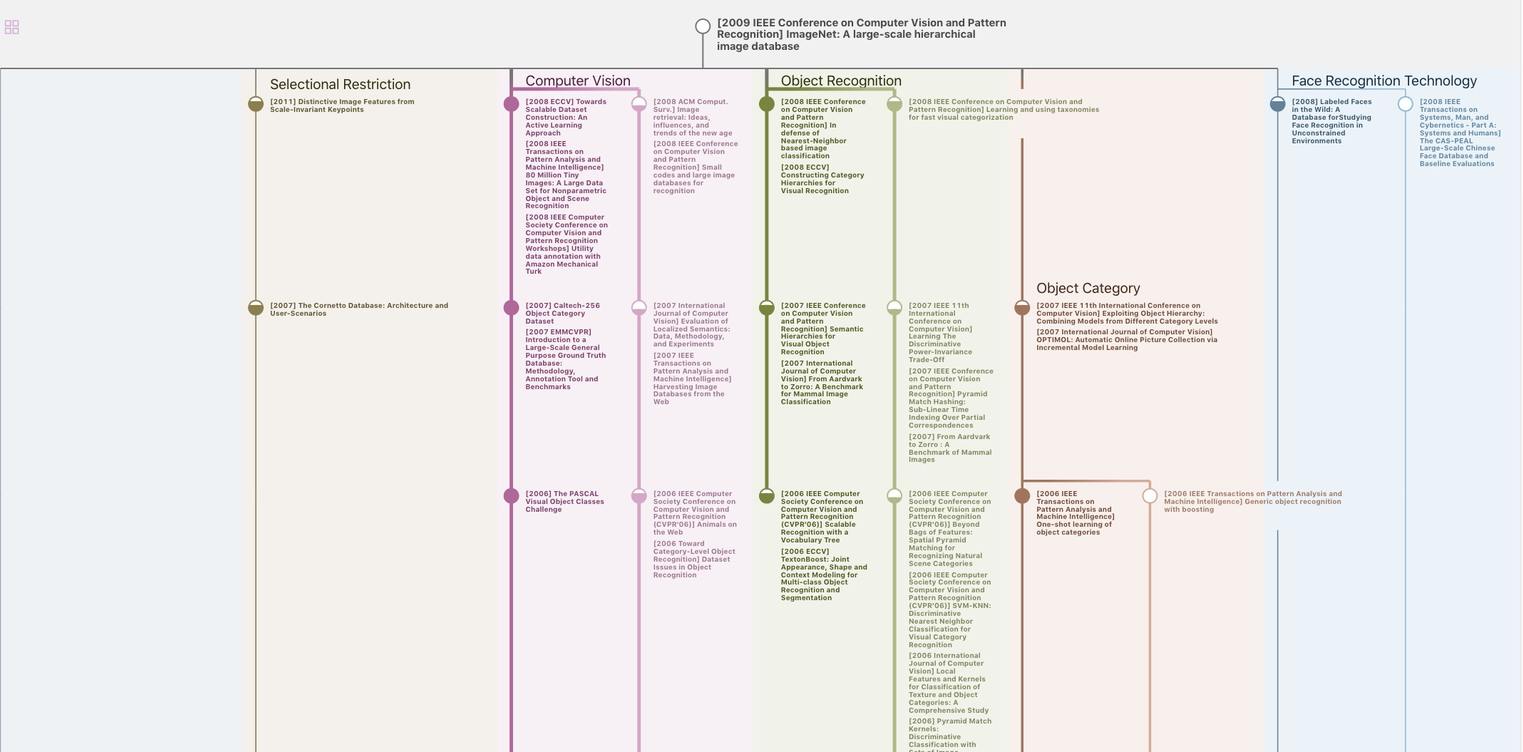
生成溯源树,研究论文发展脉络
Chat Paper
正在生成论文摘要