Phase Harmonic Correlations And Convolutional Neural Networks
INFORMATION AND INFERENCE-A JOURNAL OF THE IMA(2020)
摘要
A major issue in harmonic analysis is to capture the phase dependence of frequency representations, which carries important signal properties. It seems that convolutional neural networks have found a way. Over time-series and images, convolutional networks often learn a first layer of filters that are well localized in the frequency domain, with different phases. We show that a rectifier then acts as a filter on the phase of the resulting coefficients. It computes signal descriptors that are local in space, frequency and phase. The nonlinear phase filter becomes a multiplicative operator over phase harmonics computed with a Fourier transform along the phase. We prove that it defines a bi-Lipschitz and invertible representation. The correlations of phase harmonics coefficients characterize coherent structures from their phase dependence across frequencies. For wavelet filters, we show numerically that signals having sparse wavelet coefficients can be recovered from few phase harmonic correlations, which provide a compressive representation.
更多查看译文
关键词
neural networks, harmonics, phase, wavelets
AI 理解论文
溯源树
样例
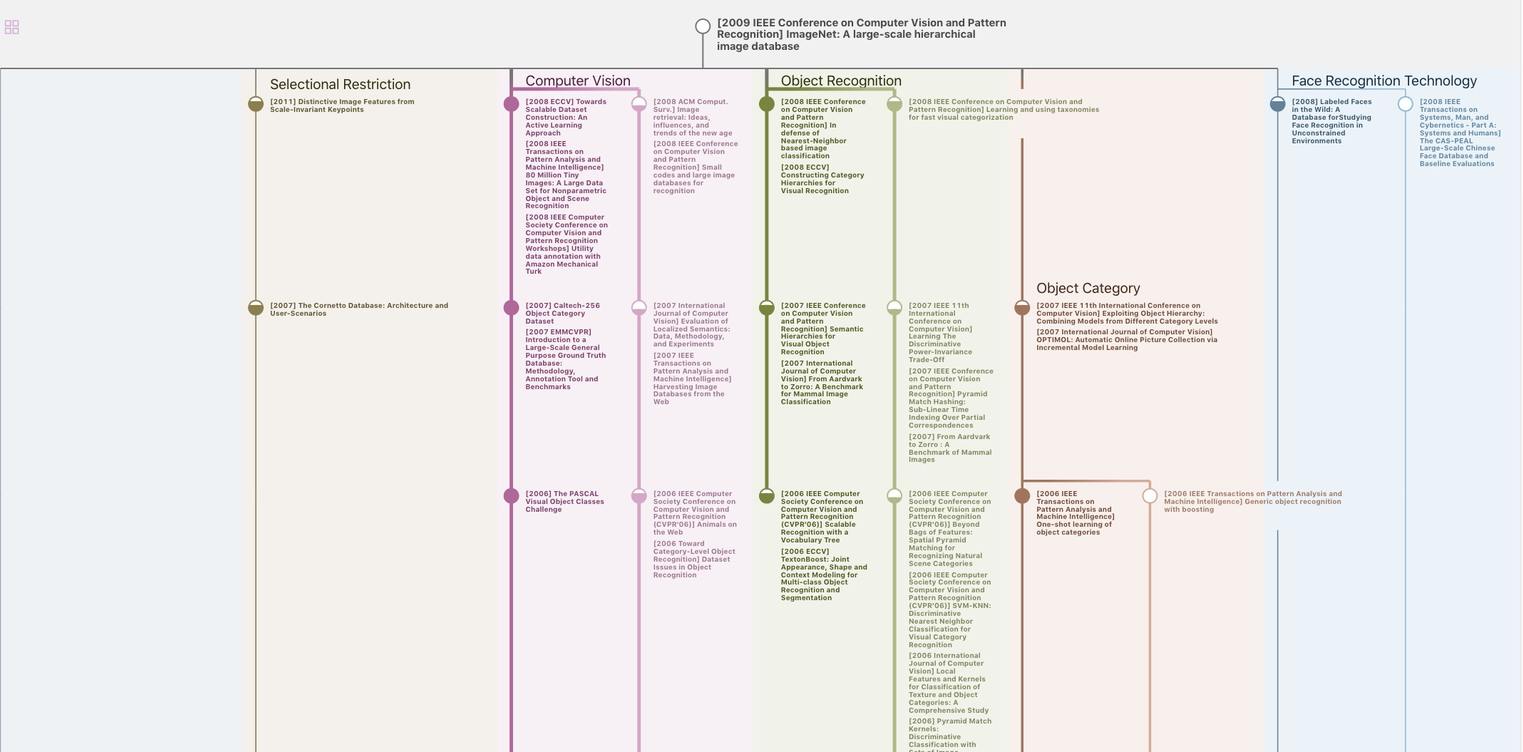
生成溯源树,研究论文发展脉络
Chat Paper
正在生成论文摘要