Learning Markov Models Via Low-Rank Optimization
Operations research(2022)
摘要
Taming high-dimensional Markov models In “Learning Markov models via low-rank optimization”, Z. Zhu, X. Li, M. Wang, and A. Zhang focus on learning a high-dimensional Markov model with low-dimensional latent structure from a single trajectory of states. To overcome the curse of high dimensions, the authors propose to equip the standard MLE (maximum-likelihood estimation) with either nuclear norm regularization or rank constraint. They show that both approaches can estimate the full transition matrix accurately using a trajectory of length that is merely proportional to the number of states. To solve the rank-constrained MLE, which is a nonconvex problem, the authors develop a new DC (difference) programming algorithm. Finally, they apply the proposed methods to analyze taxi trips on the Manhattan island and partition the island based on the destination preference of customers; this partition can help balance supply and demand of taxi service and optimize the allocation of traffic resources.
更多查看译文
关键词
Markov model,DC programming,non-convex optimization,rank constrained likelihood
AI 理解论文
溯源树
样例
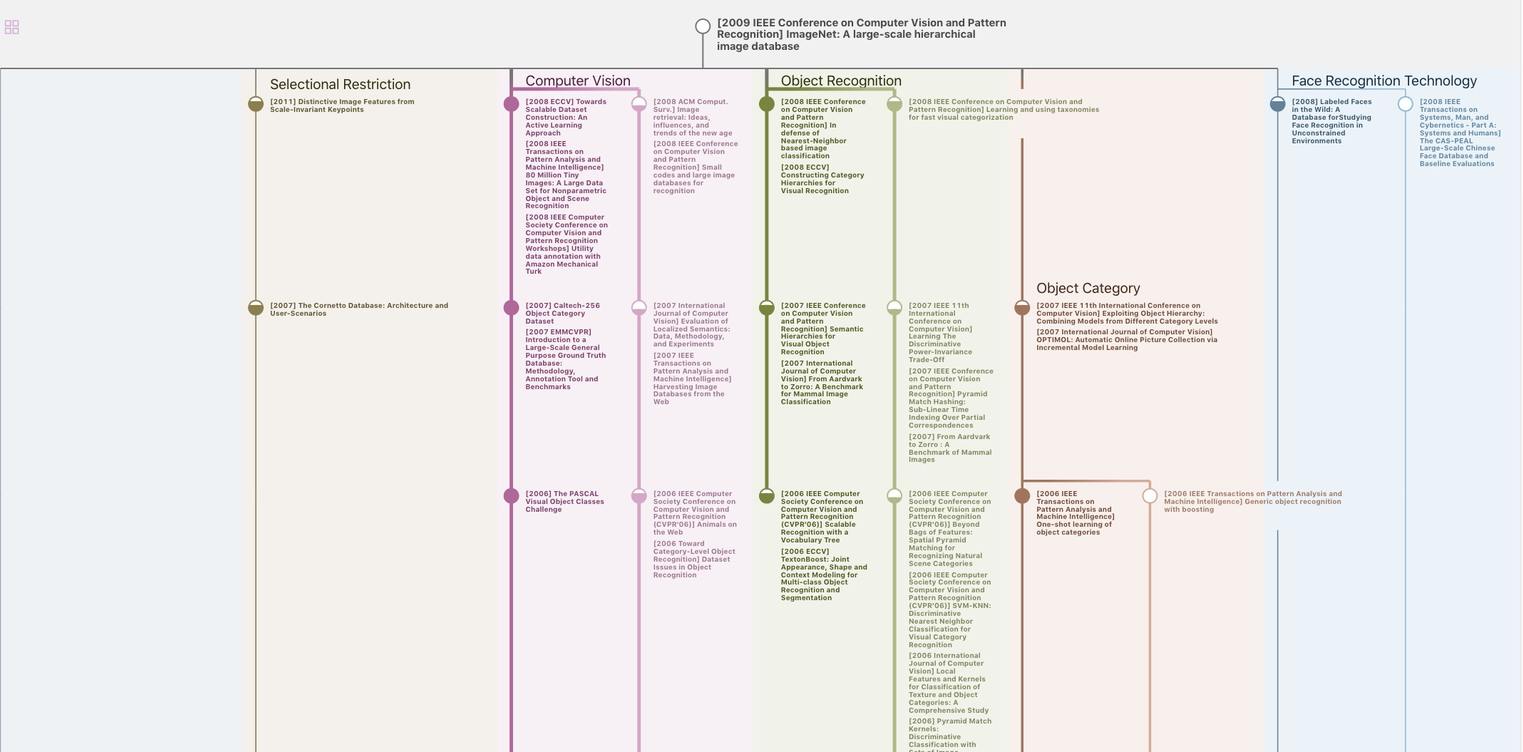
生成溯源树,研究论文发展脉络
Chat Paper
正在生成论文摘要