Designing Deep Reinforcement Learning for Human Parameter Exploration
ACM Transactions on Computer-Human Interaction(2021)
摘要
AbstractSoftware tools for generating digital sound often present users with high-dimensional, parametric interfaces, that may not facilitate exploration of diverse sound designs. In this article, we propose to investigate artificial agents using deep reinforcement learning to explore parameter spaces in partnership with users for sound design. We describe a series of user-centred studies to probe the creative benefits of these agents and adapting their design to exploration. Preliminary studies observing users’ exploration strategies with parametric interfaces and testing different agent exploration behaviours led to the design of a fully-functioning prototype, called Co-Explorer, that we evaluated in a workshop with professional sound designers. We found that the Co-Explorer enables a novel creative workflow centred on human–machine partnership, which has been positively received by practitioners. We also highlight varied user exploration behaviours throughout partnering with our system. Finally, we frame design guidelines for enabling such co-exploration workflow in creative digital applications.
更多查看译文
关键词
Interaction design, machine learning, audio/video
AI 理解论文
溯源树
样例
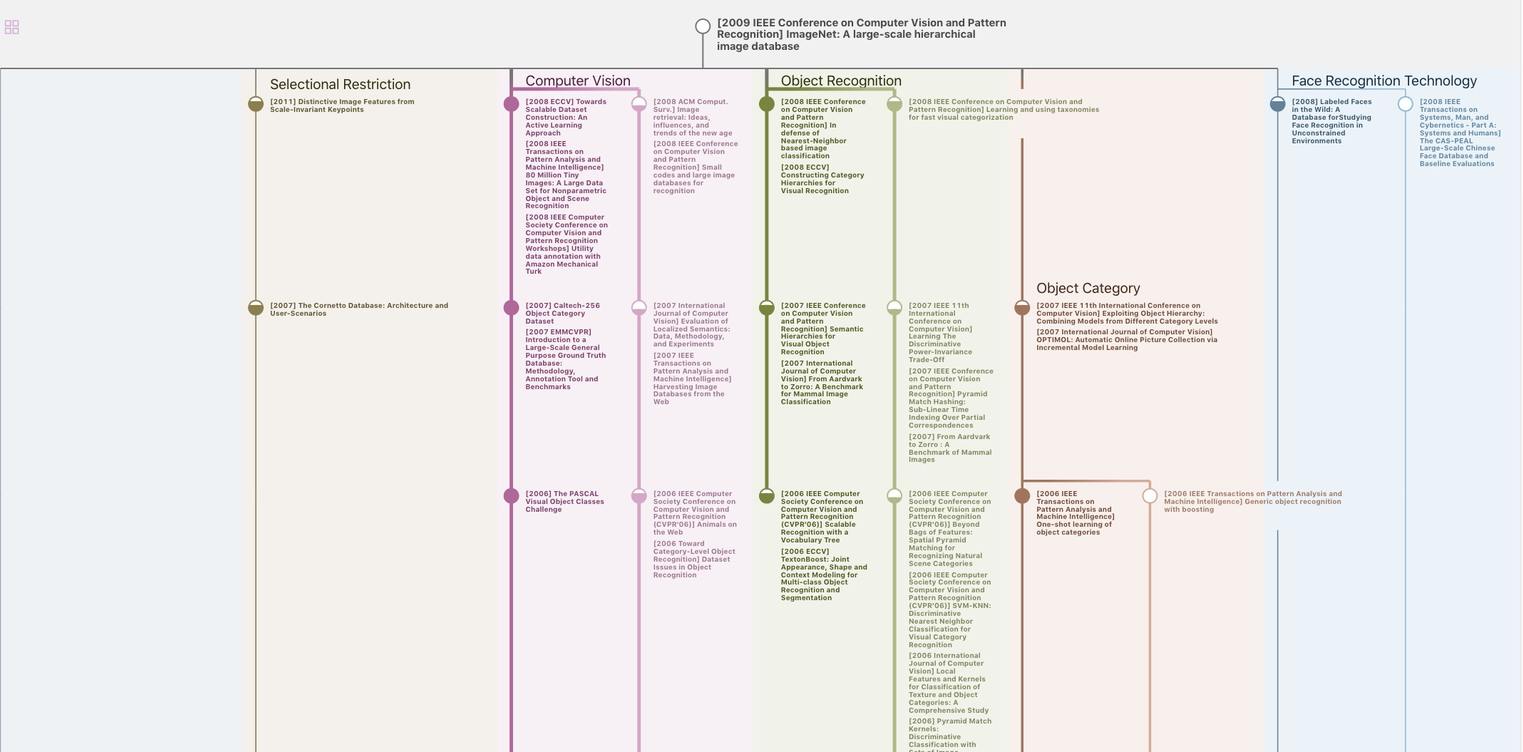
生成溯源树,研究论文发展脉络
Chat Paper
正在生成论文摘要