A Hybrid Normalization Method for Medical Concepts in Clinical Narrative using Semantic Matching.
AMIA Joint Summits on Translational Science proceedings. AMIA Joint Summits on Translational Science(2019)
摘要
Normalization maps clinical terms in medical notes to standardized medical vocabularies. In order to capture semantic similarity between different surface expressions of the same clinical concept, we develop a hybrid normalization system that incorporates a deep learning model to complement the traditional dictionary lookup ap- proach. We evaluate our system against the ShARe/CLEF 2013 challenge data in which 30% of the mentions have no concept mapping. When evaluating against the mentions which may be normalized to existing concepts, our hybrid system achieves 90.6% accuracy, obtaining a statistically significant improvement of 2.6% over a strong edit-distance and dictionary lookup combined baseline. Our analysis of semantic similarity between concepts and mentions reveals existing inconsistencies in ShARe/CLEF data, as well as problematic ambiguities in the UMLS. Our results suggest the potential of the proposed deep learning approach to further improve the performance of normalization by utilizing semantic similarity.
更多查看译文
AI 理解论文
溯源树
样例
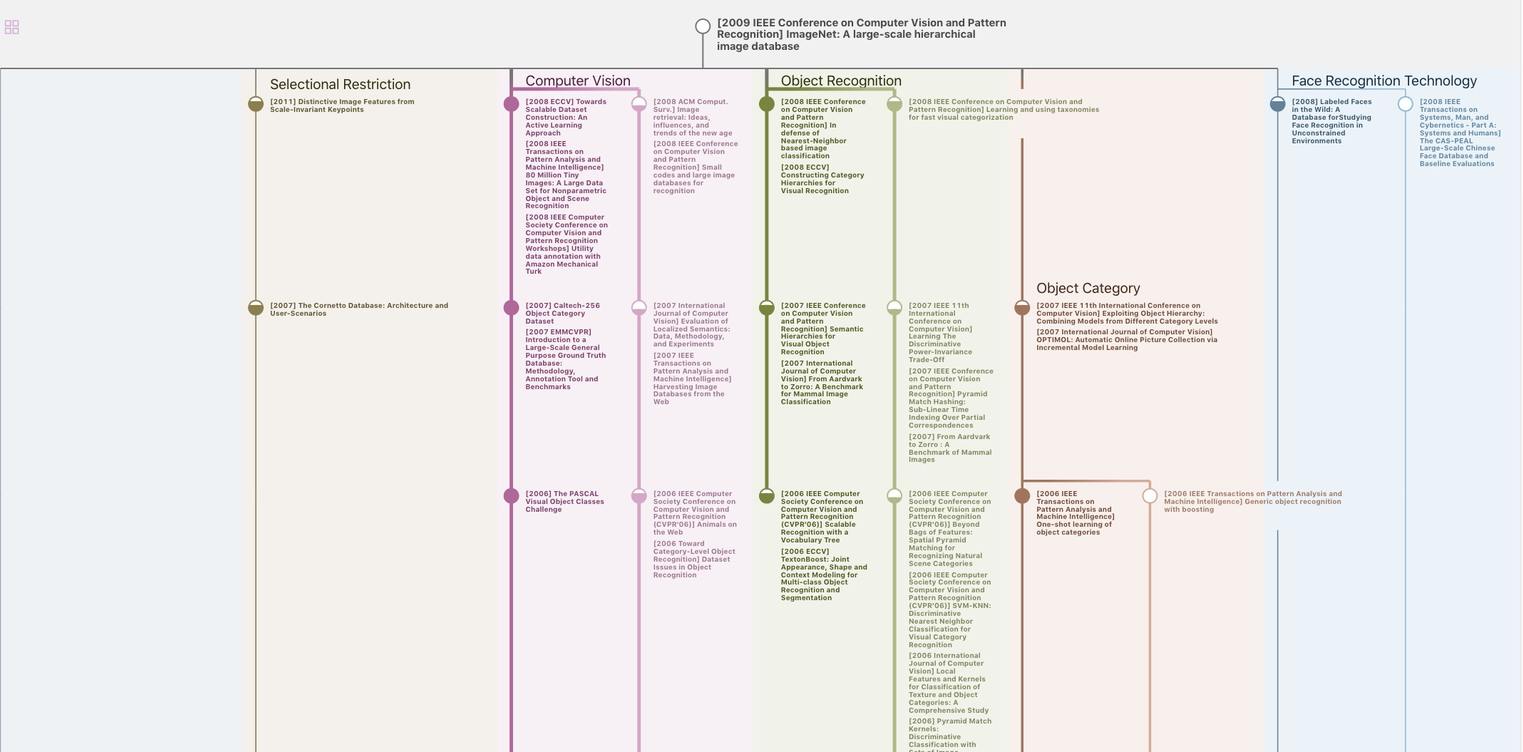
生成溯源树,研究论文发展脉络
Chat Paper
正在生成论文摘要