Bayesian Analysis (2022) 17, 2, pp. On Global-Local Shrinkage Priors for Count Data
BAYESIAN ANALYSIS(2022)
摘要
Global-local shrinkage priors have been recognized as a useful class of priors that can strongly shrink small signals toward prior means while keeping large signals unshrunk. Although such priors have been extensively discussed under Gaussian responses, in practice, we often encounter count responses. Previous contributions on global-local shrinkage priors cannot be readily applied to count data. In this paper, we discuss global-local shrinkage priors for analyzing a sequence of counts. We provide sufficient conditions under which the posterior mean is unshrunk for very large signals, known as the tail robustness property. Then, we propose tractable priors to satisfy those conditions approximately or exactly and develop a custom posterior computation algorithm for Bayesian inference without tuning parameters. We demonstrate the proposed methods through simulation studies and an application to a real dataset.
更多查看译文
关键词
heavy tailed distribution, Markov Chain Monte Carlo, Poisson distribution, tail robustness
AI 理解论文
溯源树
样例
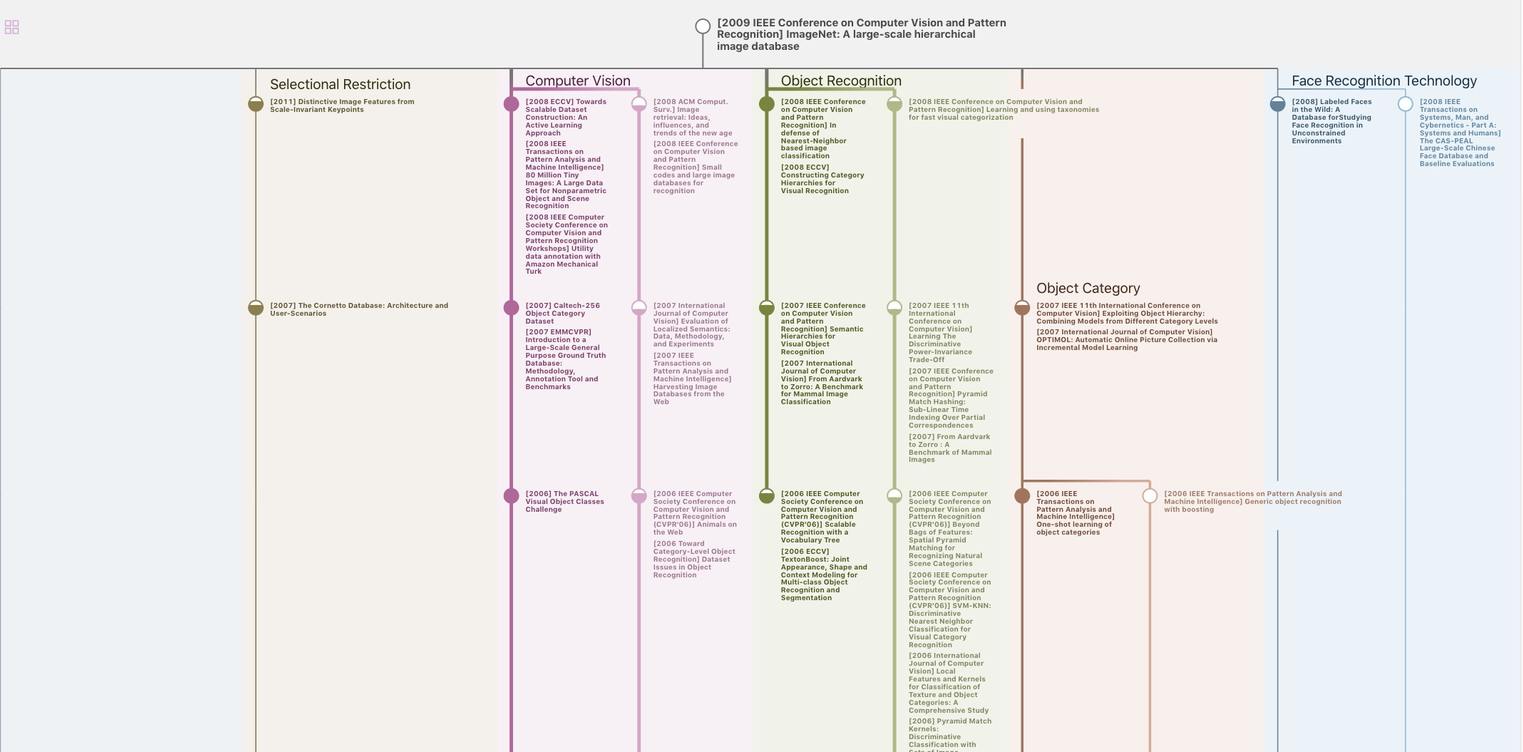
生成溯源树,研究论文发展脉络
Chat Paper
正在生成论文摘要