Towards Robust Monocular Depth Estimation: Mixing Datasets for Zero-Shot Cross-Dataset Transfer
IEEE Transactions on Pattern Analysis and Machine Intelligence(2022)
摘要
The success of monocular depth estimation relies on large and diverse training sets. Due to the challenges associated with acquiring dense ground-truth depth across different environments at scale, a number of datasets with distinct characteristics and biases have emerged. We develop tools that enable mixing multiple datasets during training, even if their annotations are incompatible. In particular, we propose a robust training objective that is invariant to changes in depth range and scale, advocate the use of principled multi-objective learning to combine data from different sources, and highlight the importance of pretraining encoders on auxiliary tasks. Armed with these tools, we experiment with five diverse training datasets, including a new, massive data source: 3D films. To demonstrate the generalization power of our approach we use
zero-shot cross-dataset transfer
, i.e. we evaluate on datasets that were not seen during training. The experiments confirm that mixing data from complementary sources greatly improves monocular depth estimation. Our approach clearly outperforms competing methods across diverse datasets, setting a new state of the art for monocular depth estimation.
更多查看译文
关键词
Monocular depth estimation,single-image depth prediction,zero-shot cross-dataset transfer,multi-dataset training
AI 理解论文
溯源树
样例
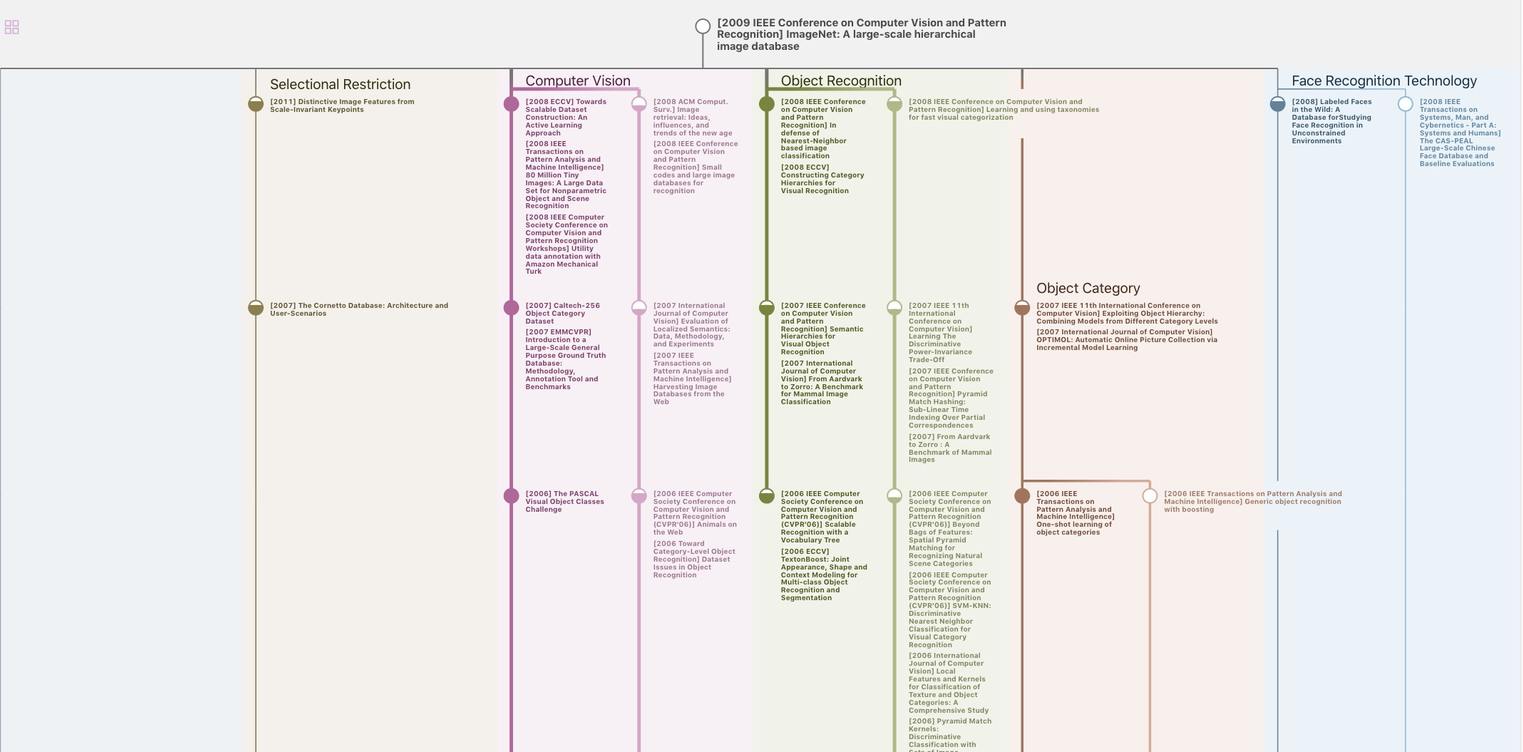
生成溯源树,研究论文发展脉络
Chat Paper
正在生成论文摘要