Quantifying Sex Bias in Clinical Studies at Scale With Automated Data Extraction.
JAMA NETWORK OPEN(2019)
摘要
IMPORTANCE Analyses of female representation in clinical studies have been limited in scope and scale. OBJECTIVE To perform a large-scale analysis of global enrollment sex bias in clinical studies. DESIGN, SETTING, AND PARTICIPANTS In this cross-sectional study, clinical studies from published articles from PubMed from 19 66 to 2018 and records from Aggregate Analysis of ClinicalTrials.gov from 19 99 to 2018 were identified. Global disease prevalence was determined for male and female patients in 11 disease categories from the Global Burden of Disease database: cardiovascular, diabetes, digestive, hepatitis (types A, B, C, and E), HIV/AIDS, kidney (chronic), mental, musculoskeletal, neoplasms, neurological, and respiratory (chronic). Machine reading algorithms were developed that extracted sex data from tables in articles and records on December 31, 2018, at an artificial intelligence research institute. Male and female participants in 43 135 articles (792 004 915 participants) and 13 165 records (12 977103 participants) were included. MAIN OUTCOMES AND MEASURES Sex bias was defined as the difference between the fraction of female participants in study participants minus prevalence fraction of female participants for each disease category. A total of 1000 bootstrap estimates of sex bias were computed by resampling individual studies with replacement. Sex bias was reported as mean and 95% bootstrap confidence intervals from articles and records in each disease category over time (before or during 19 93 to 2018), with studies or participants as the measurement unit. RESULTS There were 792 004 915 participants, including 390 470 834 female participants (49%), in articles and 12 977 103 participants, including 6 351 619 female participants (49%), in records. With studies as measurement unit, substantial female underrepresentation (sex bias <= -0.05) was observed in 7 of 11 disease categories, especially HIV/AIDS (mean for articles, -0.17 [95% CI, -018 to -0.16]), chronic kidney diseases (mean,-0.17 [95% CI,-0.17 to -0.16]), and cardiovascular diseases (mean, -0.14 [95% CI, -014 to -0.13]). Sex bias in articles for all categories combined was unchanged over time with studies as measurement unit (range, -0.15 [95% CI, -016 to -0.13] to -0.10 [95% CI, -0.14 to -0.06]), but improved from before or during 1993 (mean, -0.11[95% CI, -016 to -0.05]) to 2014 to 2018 (mean, -0.05 [95% CI, -0.09 to -0.02]) with participants as the measurement unit. Larger study size was associated with greater female representation. CONCLUSIONS AND RELEVANCE Automated extraction of the number of participants in clinical reports provides an effective alternative to manual analysis of demographic bias. Despite legal and policy initiatives to increase female representation, sex bias against female participants in clinical studies persists. Studies with more participants have greater female representation. Differences between sex bias estimates with studies vs participants as measurement unit, and between articles vs records, suggest that sex bias with both measures and data sources should be reported.
更多查看译文
AI 理解论文
溯源树
样例
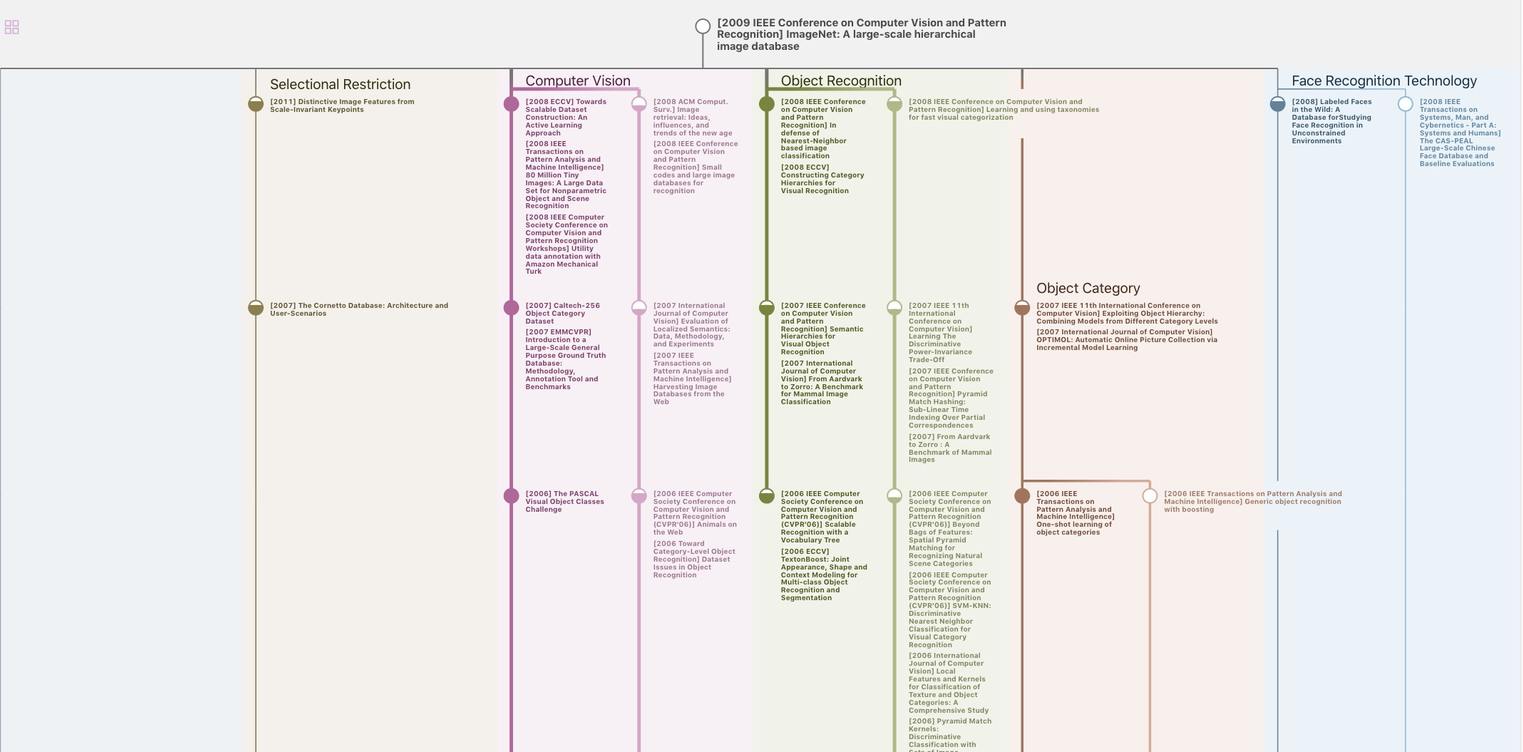
生成溯源树,研究论文发展脉络
Chat Paper
正在生成论文摘要