Searching for Interaction Functions in Collaborative Filtering
arxiv(2019)
摘要
Interaction function (IFC), which captures interactions among items and users, is of great importance in collaborative filtering (CF). The inner product is the most popular IFC due to its success in low-rank matrix factorization. However, interactions in real-world applications can be highly complex. Many other operations (such as plus and concatenation) have also been proposed, and can possibly offer better performance than the inner product. In this paper, motivated by the success of automated machine learning, we propose to search for proper interaction functions (SIF) for CF tasks. We first design an expressive search space for SIF by reviewing and generalizing existing CF approaches. We then propose to represent the search space as a structured multi-layer perceptron, and design a stochastic gradient descent algorithm which can simultaneously update both architectures and learning parameters. Experimental results demonstrate that the proposed method can be much more efficient than popular AutoML approaches, and also obtain much better prediction performance than state-of-the-art CF approaches.
更多查看译文
AI 理解论文
溯源树
样例
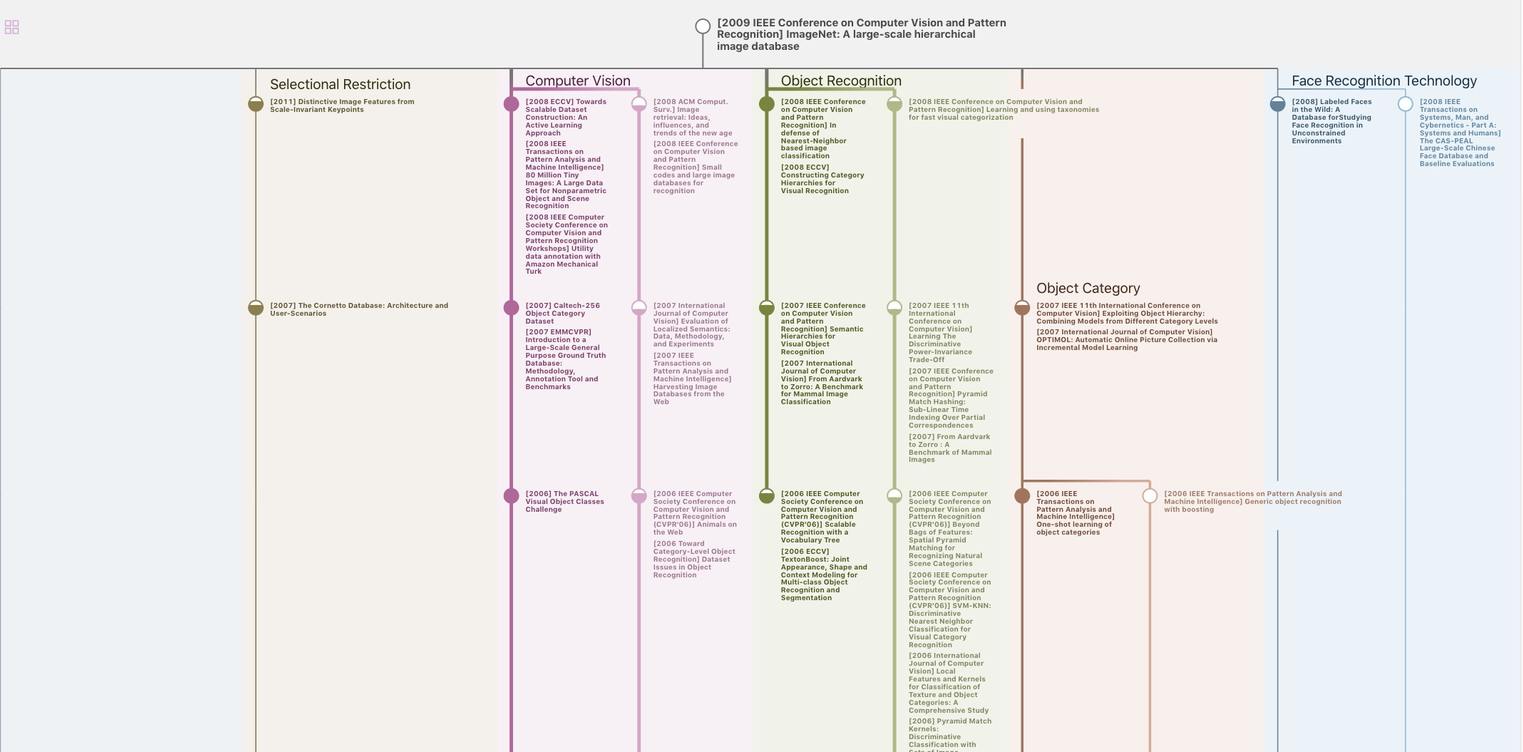
生成溯源树,研究论文发展脉络
Chat Paper
正在生成论文摘要