Efficient Parameter Estimation of Sampled Random Fields
arxiv(2019)
摘要
We provide a computationally and statistically efficient method for estimating the parameters of a stochastic Gaussian model observed on a spatial grid, which need not be rectangular. Standard methods are plagued by computational intractability, where designing methods that can be implemented for realistically sized problems has been an issue for a long time. This has motivated the use of the Fourier Transform and the Whittle likelihood approximation. The challenge of frequency-domain methods is to determine and account for observational boundary effects, missing data, and the shape of the observed spatial grid. In this paper we address these effects explicitly by proposing a new quasi-likelihood estimator. We prove consistency and asymptotic normality of our estimator in settings that include irregularly shaped grids. Our simulations show that the proposed method solves boundary issues with Whittle estimation for random fields, yielding parameter estimates with significantly reduced bias and error. We demonstrate the effectiveness of our method for incomplete lattices, in comparison to other recent methods. Finally, we apply our method to estimate the parameters of a Mat\'ern process used to model data from Venus' topography.
更多查看译文
关键词
aliasing, irregular boundaries, missing data, random fields, Whittle likelihood
AI 理解论文
溯源树
样例
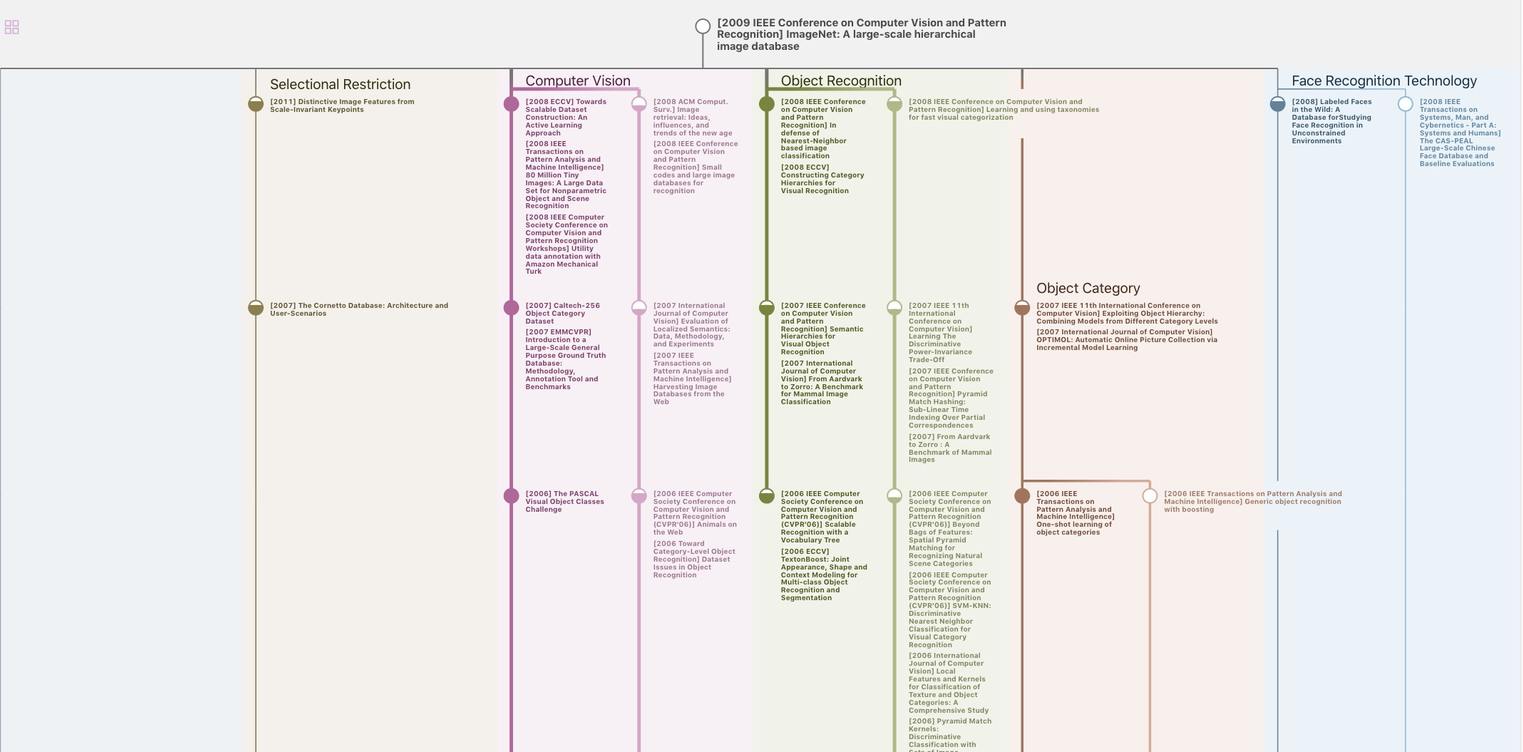
生成溯源树,研究论文发展脉络
Chat Paper
正在生成论文摘要