Convolutional neural network surrogate-assisted GOMEA
GECCO(2019)
摘要
ABSTRACTWe introduce a novel surrogate-assisted Genetic Algorithm (GA) for expensive optimization of problems with discrete categorical variables. Specifically, we leverage the strengths of the Gene-pool Optimal Mixing Evolutionary Algorithm (GOMEA), a state-of-the-art GA, and, for the first time, propose to use a convolutional neural network (CNN) as a surrogate model. We propose to train the model on pairwise fitness differences to decrease the number of evaluated solutions that is required to achieve adequate surrogate model training. In providing a proof of principle, we consider relatively standard CNNs, and demonstrate that their capacity is already sufficient to accurately learn fitness landscapes of various well-known benchmark functions. The proposed CS-GOMEA is compared with GOMEA and the widely-used Bayesian-optimization-based expensive optimization frameworks SMAC and Hyperopt, in terms of the number of evaluations that is required to achieve the optimum. In our experiments on binary problems with dimensionalities up to400 variables, CS-GOMEA always found the optimum, whereas SMAC and Hyperopt failed for problem sizes over 16 variables. Moreover, the number of evaluated solutions required by CS-GOMEA to find the optimum was found to scale much better than GOMEA.
更多查看译文
关键词
GOMEA, surrogate-assisted GA, convolutional neural network, surrogate model, discrete optimization, expensive optimization
AI 理解论文
溯源树
样例
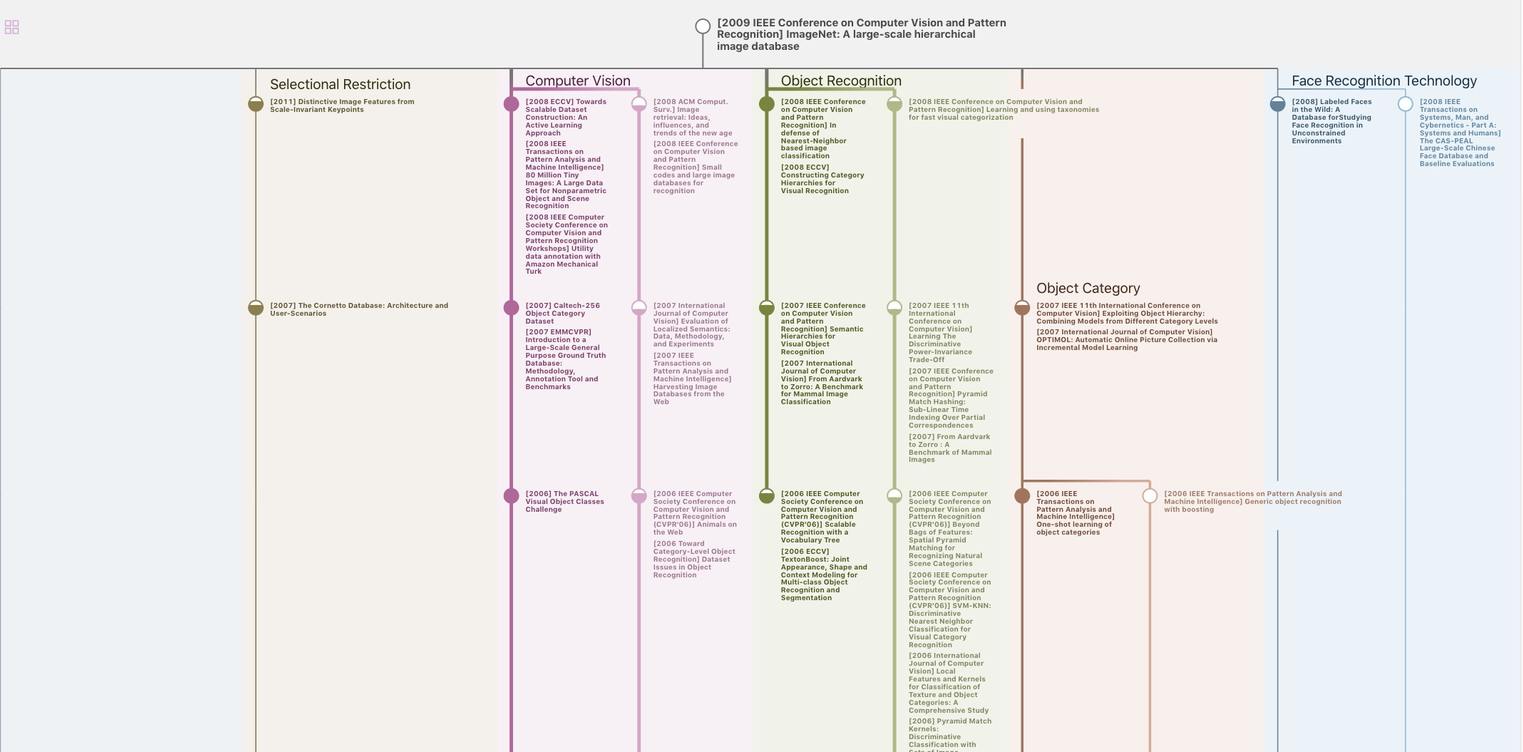
生成溯源树,研究论文发展脉络
Chat Paper
正在生成论文摘要