Improvement of code fragment fitness to guide feature construction in XCS.
GECCO(2019)
摘要
In complex classification problems, constructed features with rich discriminative information can simplify decision boundaries. Code Fragments (CFs) produce GP-tree-like constructed features that can represent decision boundaries effectively in Learning Classifier Systems (LCSs). But the search space for useful CFs is vast due to this richness in boundary creation, which is impractical. Online Feature-generation (OF) improves the search of useful CFs by growing promising CFs from a dynamic list of preferable CFs based on the ability to produce accurate and generalised, i.e. high-fitness, classifiers. However, the previous preference for high-numerosity CFs did not encapsulate information about the applicability of CFs directly. Consequently, learning performances of OF with an accuracy-based LCS (termed XOF) struggled to progress in the final learning phase. The hypothesis is that estimating the CF-fitness of CFs based on classifier fitness will aid the search for useful constructed features. This is anticipated to drive the search of decision boundaries efficiently, and thereby improve learning performances. Experiments on large-scale and hierarchical Boolean problems show that the proposed systems learn faster than traditional LCSs regarding the number of generations and time consumption. Tests on real-world datasets demonstrate its capability to find readable and useful features to solve practical problems.
更多查看译文
关键词
XCS, XCSCFC, LCS, genetic programming, code fragment
AI 理解论文
溯源树
样例
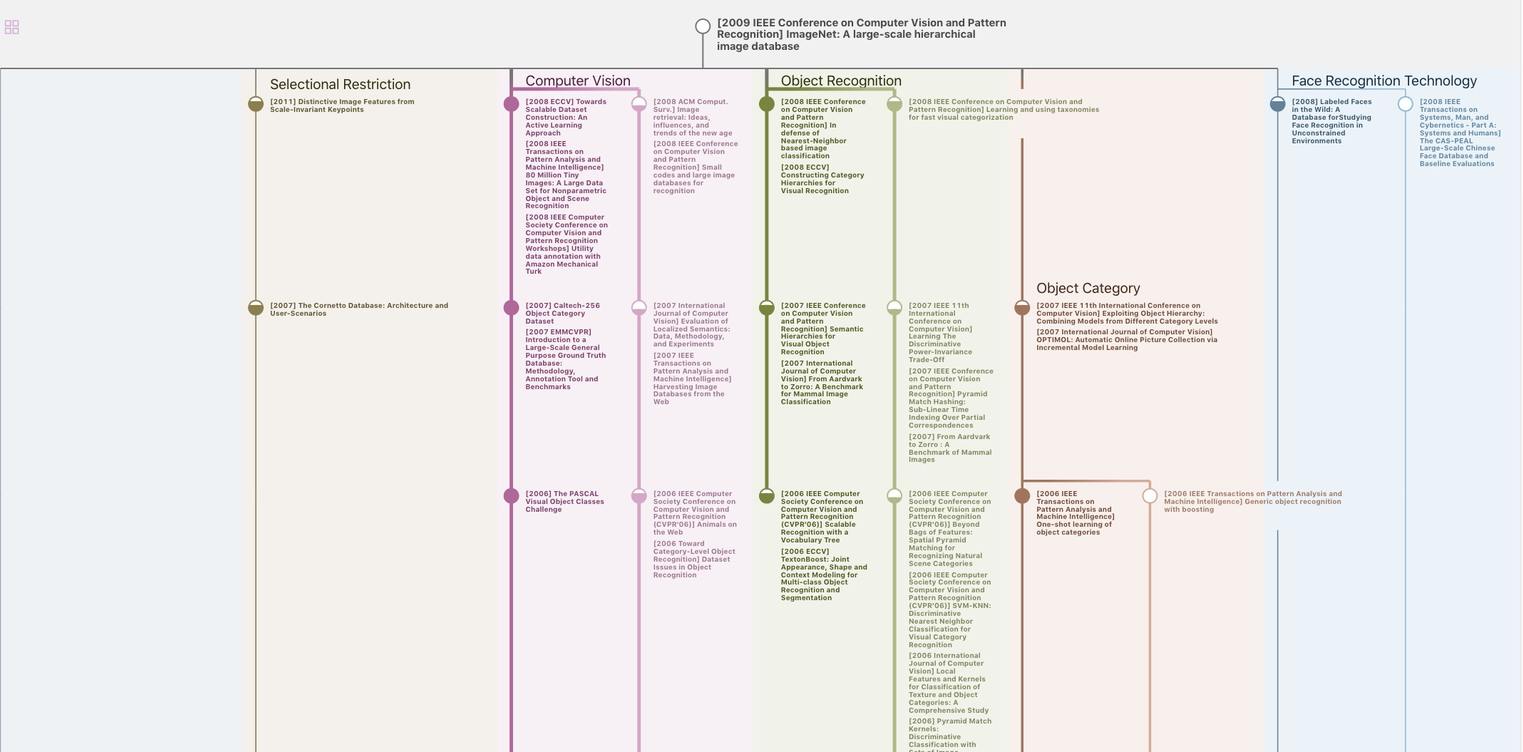
生成溯源树,研究论文发展脉络
Chat Paper
正在生成论文摘要