Guided Bayesian Optimization to AutoTune Memory-Based Analytics
2019 IEEE 35th International Conference on Data Engineering Workshops (ICDEW)(2019)
摘要
There is a lot of interest today in building autonomous (or, self-driving) data processing systems. An emerging school of thought is to leverage the "black box" algorithm of Bayesian Optimization for problems of this flavor both due to its wider applicability and theoretical guarantees on the quality of results produced. The black-box approach, however, could be time and labor-intensive; or otherwise get stuck in a local minima. We study an important problem of auto-tuning the memory allocation for applications running on modern distributed data processing systems. A simple "white-box" model is developed which can quickly separate good configurations from bad ones. To combine the benefits of the two approaches to tuning, we build a framework called Guided Bayesian Optimization (GBO) that uses the white-box model as a guide during the Bayesian Optimization exploration process. An evaluation carried out on Apache Spark using industry-standard benchmark applications shows that GBO consistently provides performance speedups across the application workload with the magnitude of savings being close to 2x.
更多查看译文
关键词
data analytics,auto tuning
AI 理解论文
溯源树
样例
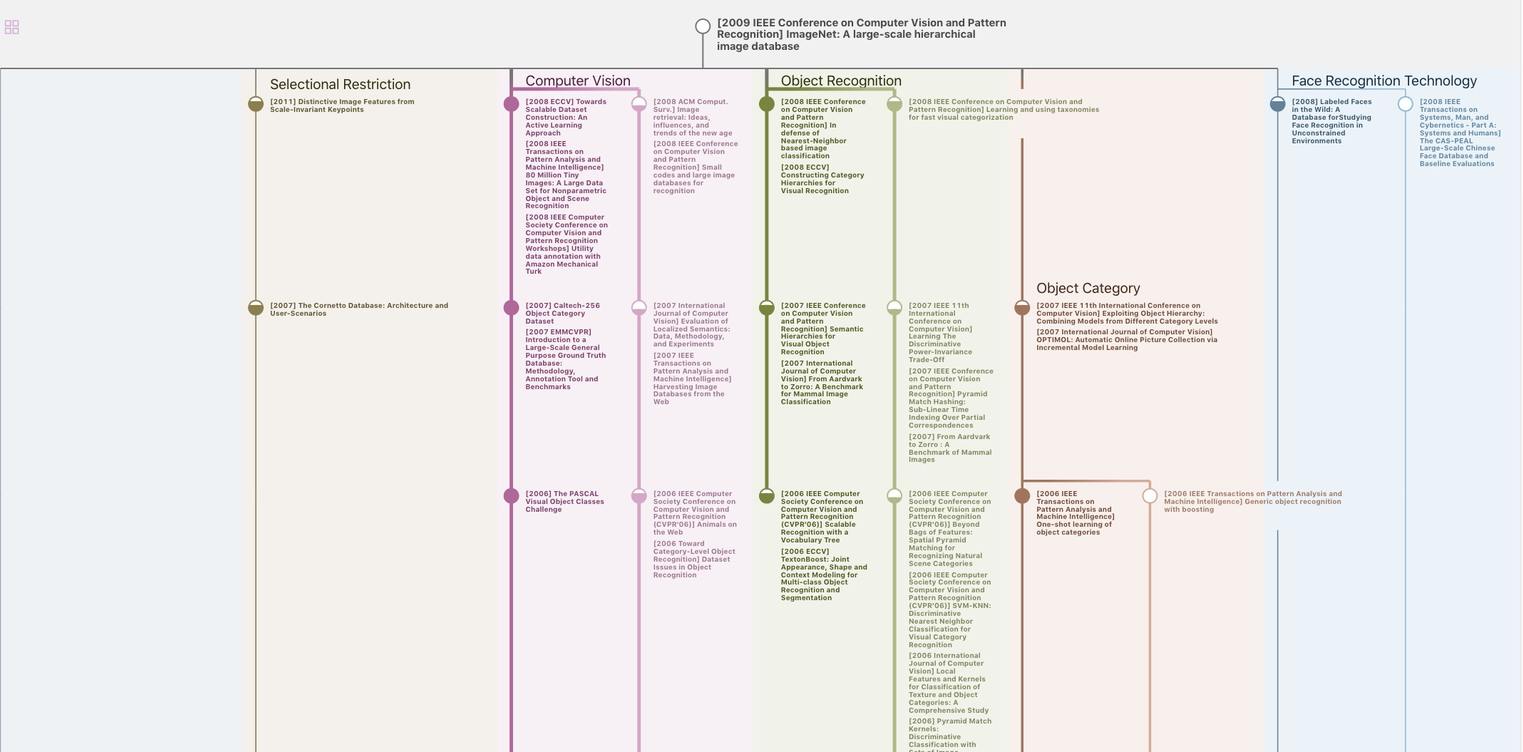
生成溯源树,研究论文发展脉络
Chat Paper
正在生成论文摘要