Prior Activation Distribution (PAD): A Versatile Representation to Utilize DNN Hidden Units
arxiv(2019)
摘要
In this paper, we introduce the concept of Prior Activation Distribution (PAD) as a versatile and general technique to capture the typical activation patterns of hidden layer units of a Deep Neural Network used for classification tasks. We show that the combined neural activations of such a hidden layer have class-specific distributional properties, and then define multiple statistical measures to compute how far a test sample's activations deviate from such distributions. Using a variety of benchmark datasets (including MNIST, CIFAR10, Fashion-MNIST & notMNIST), we show how such PAD-based measures can be used, independent of any training technique, to (a) derive fine-grained uncertainty estimates for inferences; (b) provide inferencing accuracy competitive with alternatives that require execution of the full pipeline, and (c) reliably isolate out-of-distribution test samples.
更多查看译文
AI 理解论文
溯源树
样例
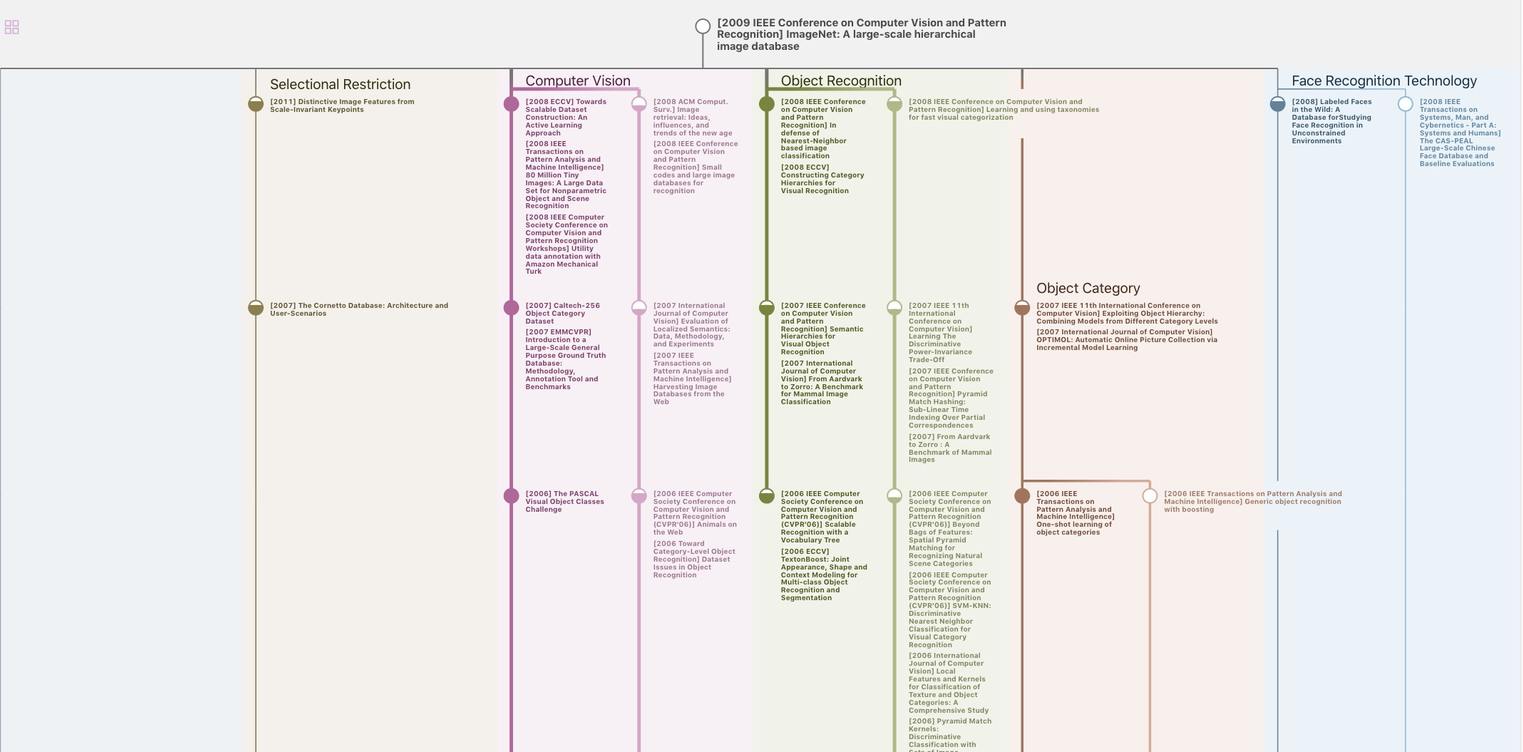
生成溯源树,研究论文发展脉络
Chat Paper
正在生成论文摘要