Confrustion In Learning From Erroneous Examples: Does Type Of Prompted Self-Explanation Make A Difference?
ARTIFICIAL INTELLIGENCE IN EDUCATION (AIED 2019), PT I(2019)
摘要
Confrustion, a mix of confusion and frustration sometimes experienced while grappling with instructional materials, is not necessarily detrimental to learning. Prior research has shown that studying erroneous examples can increase students' experiences of confrustion, while at the same time helping them learn and overcome their misconceptions. In the study reported in this paper, we examined students' knowledge and misconceptions about decimal numbers before and after they interacted with an intelligent tutoring system presenting either erroneous examples targeting misconceptions (erroneous example condition) or practice problems targeting the same misconceptions (problem-solving condition). While students in both conditions significantly improved their performance from pretest to posttest, students in the problem-solving condition improved significantly more and experienced significantly less confrustion. When controlling for confrustion levels, there were no differences in performance. This study is interesting in that, unlike prior studies, the higher confrustion that resulted from studying erroneous examples was not associated with better learning outcomes; instead, it was associated with poorer learning. We propose several possible explanations for this different outcome and hypothesize that revisions to the explanation prompts to make them more expert-like may have also made them - and the erroneous examples that they targeted - less understandable and less effective. Whether prompted self-explanation options should be modeled after the shorter, less precise language students tend to use or the longer, more precise language of experts is an open question, and an important one both for understanding the mechanisms of self-explanation and for designing self-explanation options deployed in instructional materials.
更多查看译文
关键词
Erroneous examples, Affect, Confusion, Frustration, Affect detection, Learning outcomes
AI 理解论文
溯源树
样例
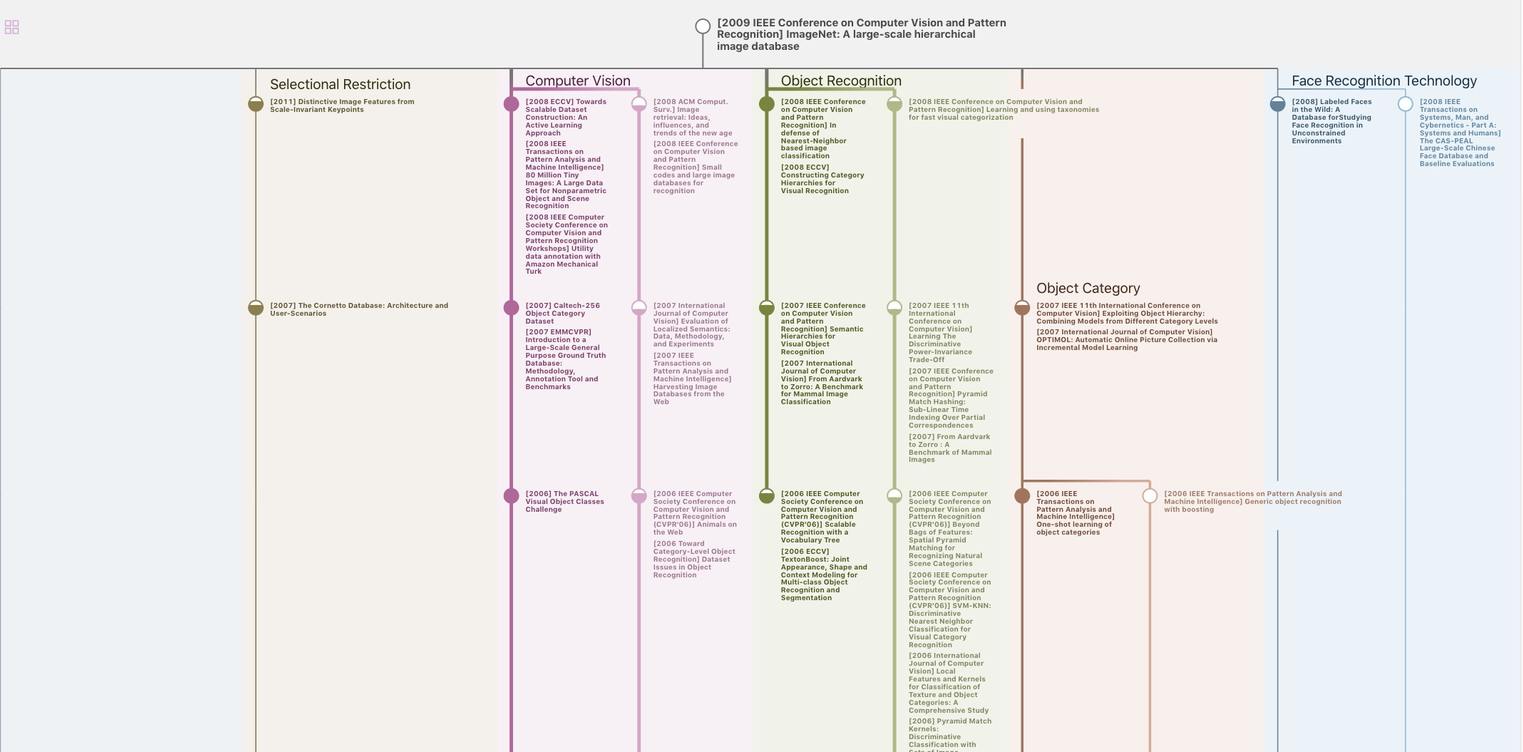
生成溯源树,研究论文发展脉络
Chat Paper
正在生成论文摘要