Fast And Scalable Outlier Detection With Metric Access Methods
COMPUTATIONAL SCIENCE - ICCS 2019, PT II(2019)
摘要
It is well-known that the existing theoretical models for outlier detection make assumptions that may not reflect the true nature of outliers in every real application. With that in mind, this paper describes an empirical study performed on unsupervised outlier detection using 8 algorithms from the state-of-the-art and 8 datasets that refer to a variety of real-world tasks of high impact, like spotting cyberattacks, clinical pathologies and abnormalities in nature. We present the lowdown on the results obtained, pointing out to the strengths and weaknesses of each technique from the application specialist's point of view, which is a shift from the designer-based point of view that is commonly considered. Interestingly, many of the techniques had unfeasibly high runtime requirements or failed to spot what the specialists consider as outliers in their own data. To tackle this issue, we propose MetricABOD: a novel angle-based outlier detection algorithm that makes the analysis up to thousands of times faster, still being in average 26% more accurate than the most accurate related work. This improvement is essential to enable outlier detection in many real-world applications for which the existing methods lead to unexpected results or unfeasible runtime requirements. Finally, we studied two real collections of text data to show that our MetricABOD works also for adimensional, purely metric data.
更多查看译文
关键词
Applied computational sciences, Complex data, Data Mining, Unsupervised outlier detection, Metric Access Methods
AI 理解论文
溯源树
样例
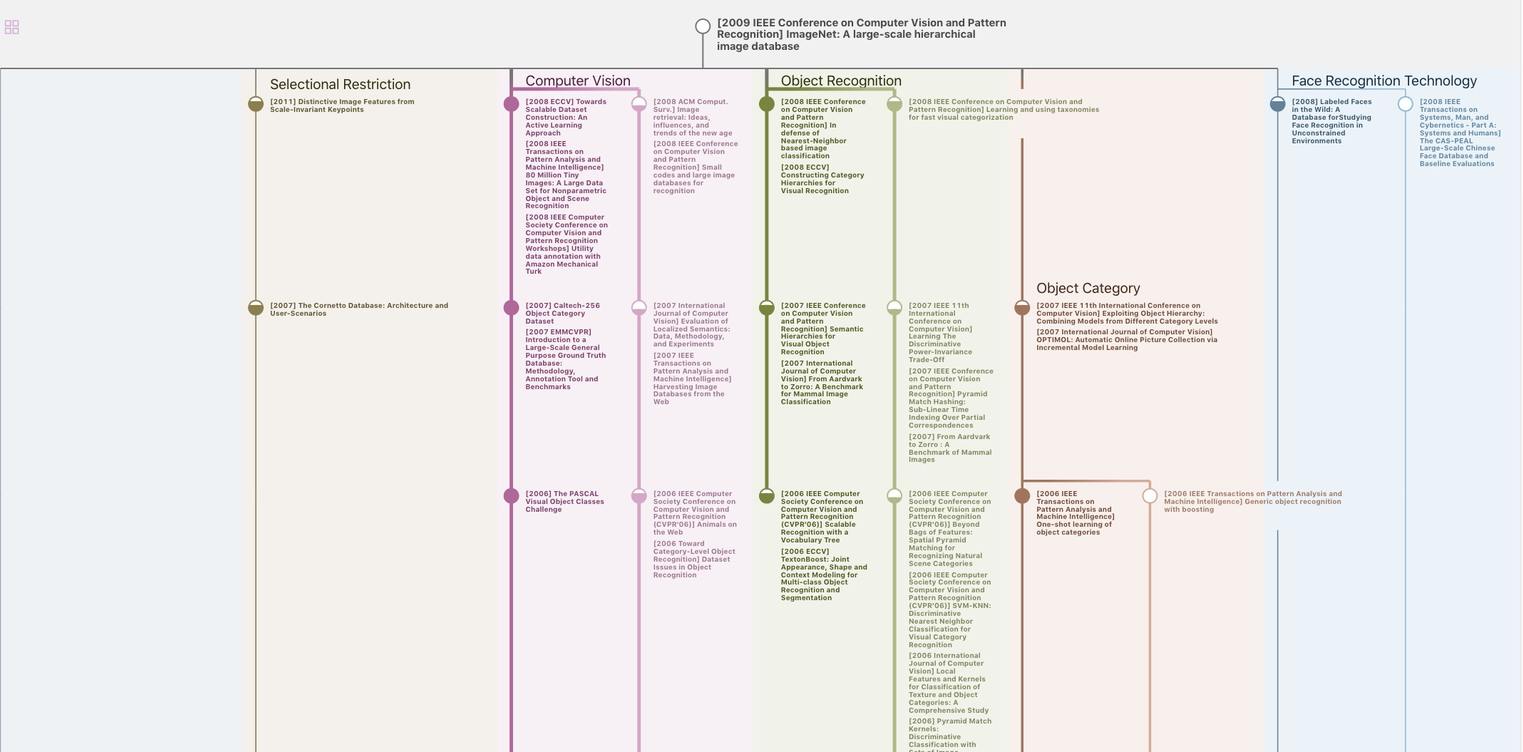
生成溯源树,研究论文发展脉络
Chat Paper
正在生成论文摘要