Transfer Learning for Leisure Centre Energy Consumption Prediction
Lecture Notes in Computer Science Computational Science – ICCS 2019(2019)
摘要
Demand for energy is ever growing. Accurate prediction of energy demand of large buildings becomes essential for property managers to operate these facilitates more efficient and greener. Various temporal modelling provides a reliable yet straightforward paradigm for short term building energy prediction. However, newly constructed buildings and recently renovated buildings, or buildings that have energy monitoring systems newly installed, do not have sufficient data to develop accurate energy demand prediction models. In contrast, established buildings often have vast amounts of data collected which may be lying idle. The model learned from these buildings with huge data can be useful if transferred to buildings with little or no data. An ensemble tree-based machine learning algorithm and datasets from two leisure centres and an office building in Melbourne were used in this transfer learning investigation. The results show that transfer learning is a promising technique in predicting accurately under a new scenario as it can achieve similar or even better performance compared to learning on a full dataset. The results also demonstrated the importance of time series adaptation as a method of improving transfer learning.
更多查看译文
关键词
Transfer learning,Energy use prediction,Leisure centre
AI 理解论文
溯源树
样例
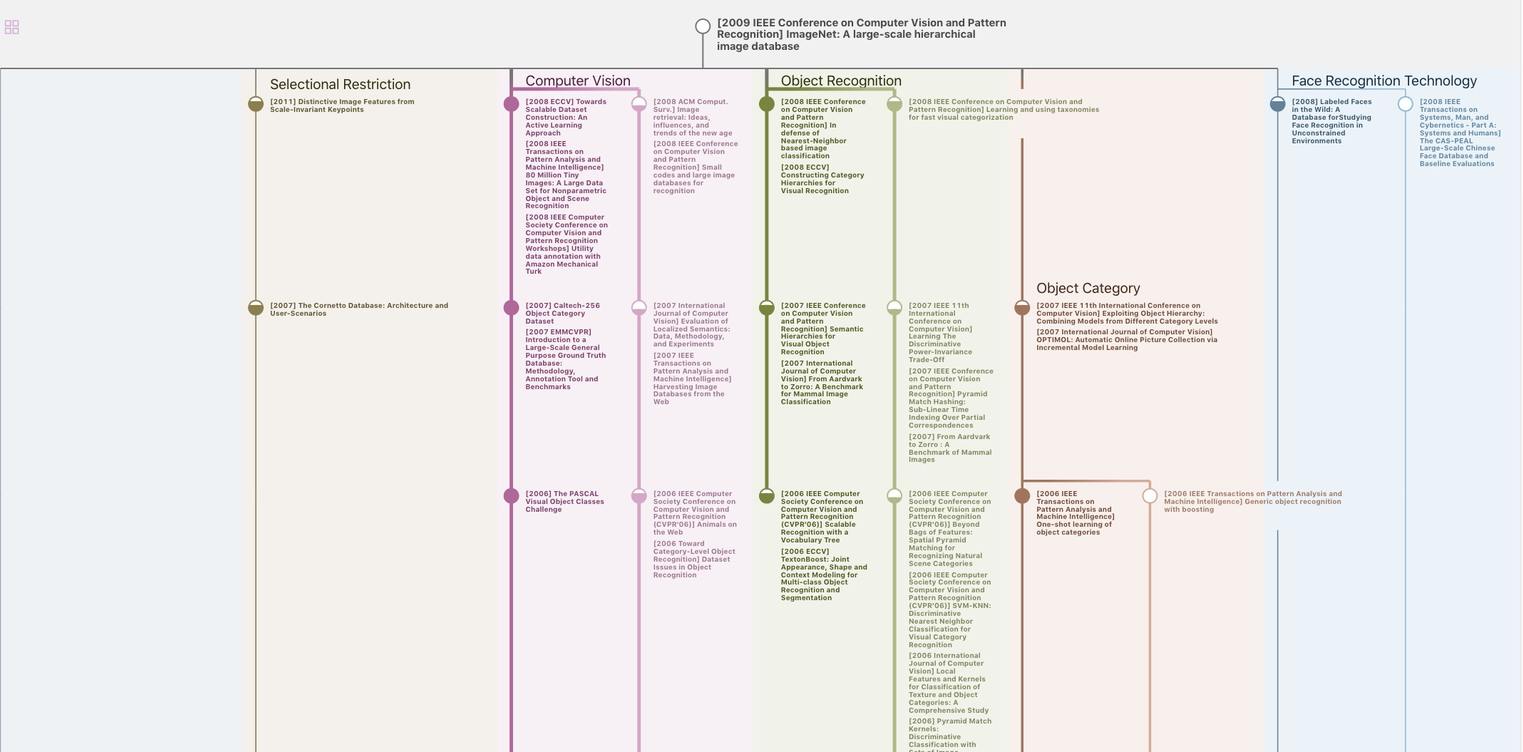
生成溯源树,研究论文发展脉络
Chat Paper
正在生成论文摘要