A Hierarchical And Scalable Strategy For Protein Structural Classification
BIOINFORMATICS AND BIOMEDICAL ENGINEERING, IWBBIO 2019, PT I(2019)
摘要
Protein function prediction is a relevant but challenging task as protein structural data is a large and complex information. With the increase of biological data available there is a demand for computational methods to annotate and help us make sense of this data deluge. Here we propose a model and a data mining based strategy to perform protein structural classification. We are particularly interested in hierarchical classification schemes. To evaluate the proposed strategy, we conduct three experiments using as input protein structural data from biological databases (CATH, SCOPe and BRENDA). Each dataset is associated with a well known hierarchical classification scheme (CATH, SCOP, EC number). We show that our model accuracy ranges from 86% to 95% when predicting CATH, SCOP and EC Number levels respectively. To the best of our knowledge, ours is the first work to reach such high accuracy when dealing with very large data sets.
更多查看译文
关键词
Protein hierarchical classification, CATH, EC number, SCOP
AI 理解论文
溯源树
样例
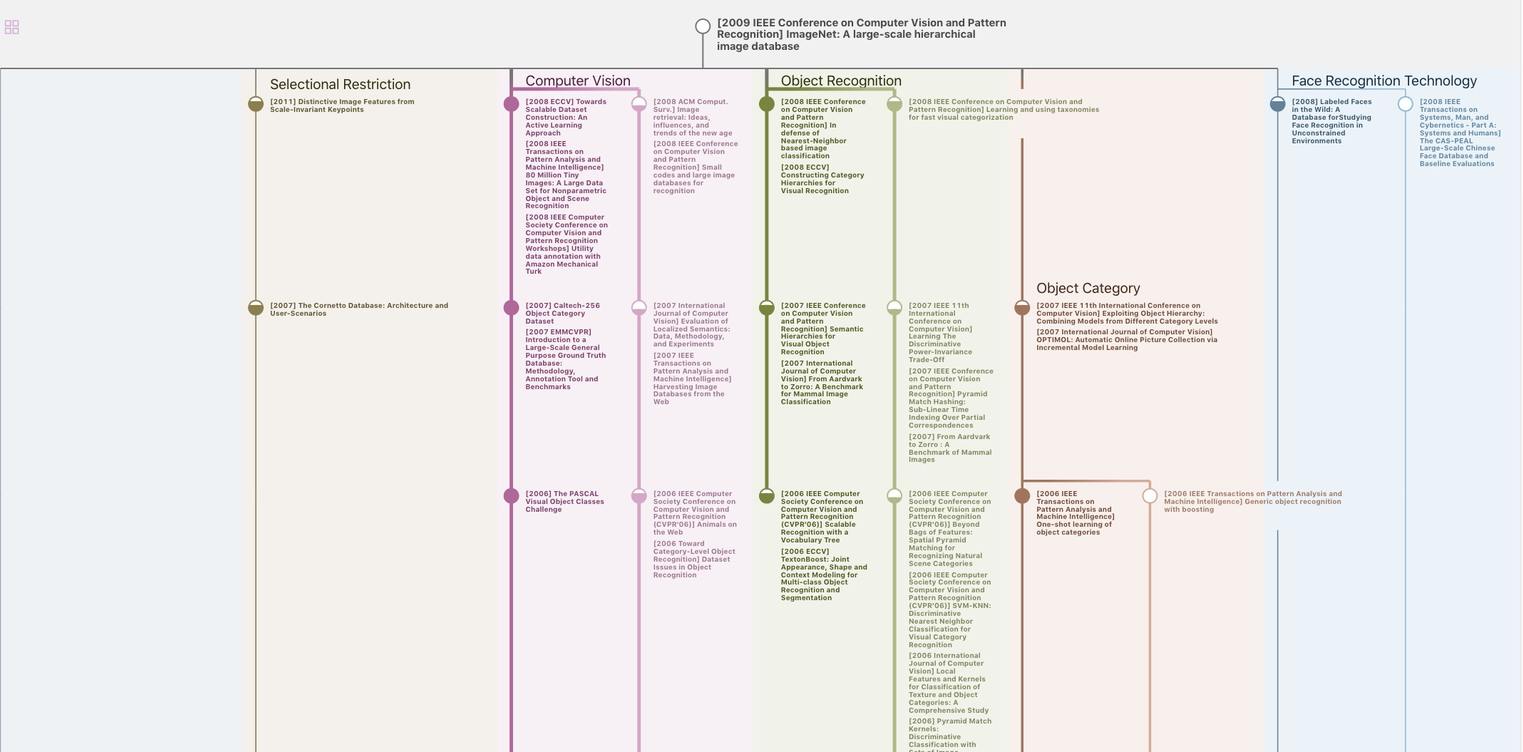
生成溯源树,研究论文发展脉络
Chat Paper
正在生成论文摘要