Restarting Frank–Wolfe: Faster Rates under Hölderian Error Bounds
22ND INTERNATIONAL CONFERENCE ON ARTIFICIAL INTELLIGENCE AND STATISTICS, VOL 89(2022)
摘要
Conditional gradient algorithms (aka Frank–Wolfe algorithms) form a classical set of methods for constrained smooth convex minimization due to their simplicity, the absence of projection steps, and competitive numerical performance. While the vanilla Frank–Wolfe algorithm only ensures a worst-case rate of 𝒪(1/ϵ ) , various recent results have shown that for strongly convex functions on polytopes, the method can be slightly modified to achieve linear convergence. However, this still leaves a huge gap between sublinear 𝒪(1/ϵ ) convergence and linear 𝒪(log 1/ϵ ) convergence to reach an ϵ -approximate solution. Here, we present a new variant of conditional gradient algorithms that can dynamically adapt to the function’s geometric properties using restarts and smoothly interpolates between the sublinear and linear regimes. These interpolated convergence rates are obtained when the optimization problem satisfies a new type of error bounds, which we call strong Wolfe primal bounds . They combine geometric information on the constraint set with Hölderian error bounds on the objective function.
更多查看译文
关键词
frank-wolfe
AI 理解论文
溯源树
样例
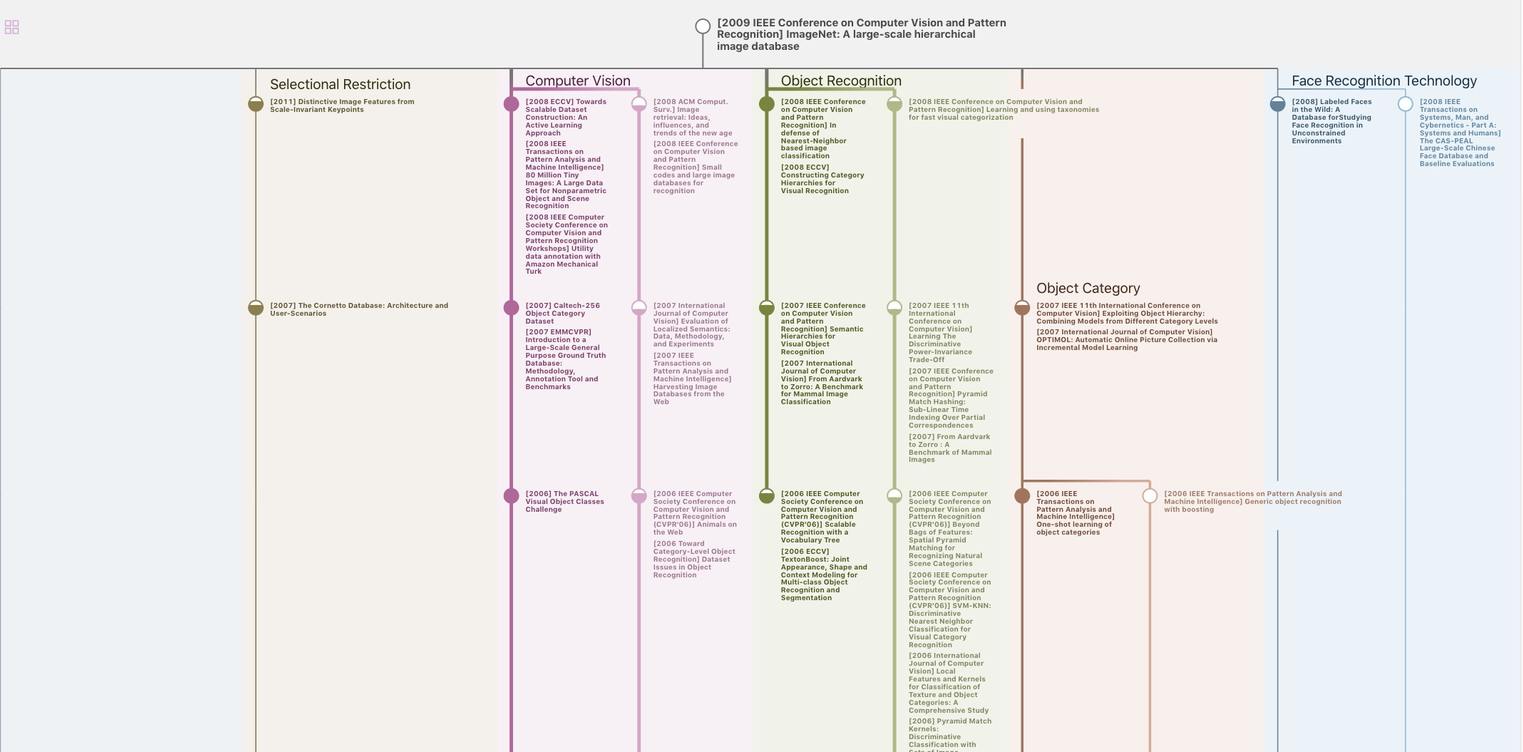
生成溯源树,研究论文发展脉络
Chat Paper
正在生成论文摘要