Object Counting Based on Density Using Perspective Transformation
2019 IEEE-RIVF International Conference on Computing and Communication Technologies (RIVF)(2019)
摘要
This paper presents a framework to count objects in images with perspective issues. The counting framework based on density estimation of the two authors Victor Lempitsky and Andrew Zisserman [1] produces excellent results in counting objects that are severely overlapped. However, the framework does not address the perspective issues, which frequently happen in many situations. It assumes that the subjected objects have the same size in every location of the images. Therefore, we propose a perspective model and integrate it into the existing density estimation framework to reduce the error caused by perspective distortions. In order to generate the perspective model, the heights of some objects are collected in addition to their locations to learn a perspective map. Using the map, we estimate the heights of the objects based on their positions in the images. The framework is adapted to exploit the height information of objects to customize the size of the normalized 2D Gaussian kernel, which is used to generate the ground truth density of images, and the size of the Gaussian window when computing the Scale- Invariant Feature Transform (SIFT) features. The adapted framework is then applied to count the number of motorbikes in Hanoi streets. The results are later compared with the ones produced by the original framework to see the effectiveness of our proposed solution. Our proposed framework performs better in high-density images while maintaining competitive accuracy in low-density images.
更多查看译文
关键词
Object counting,density estimation,perspective issues
AI 理解论文
溯源树
样例
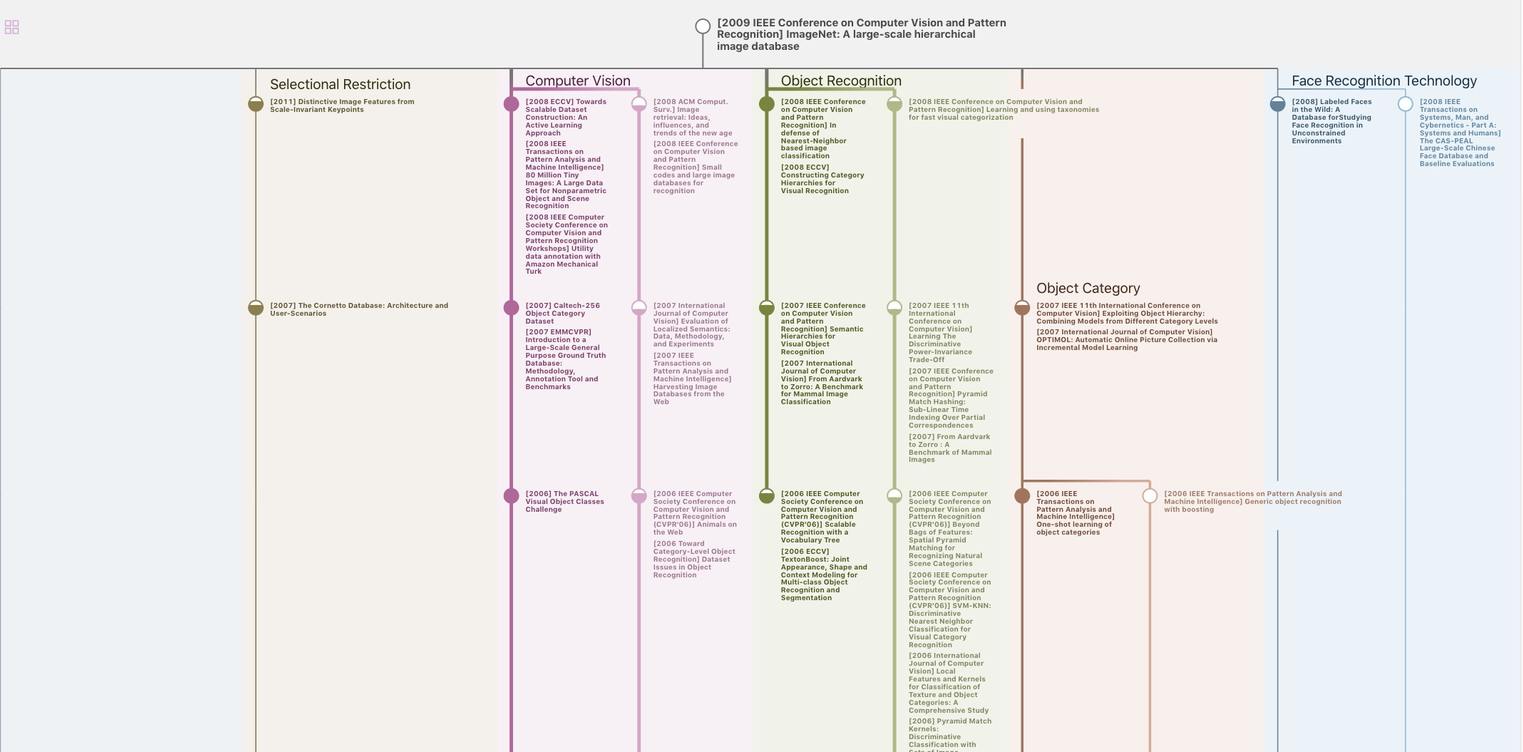
生成溯源树,研究论文发展脉络
Chat Paper
正在生成论文摘要