Discovering Correlation in Frequent Subgraphs.
PROCEEDINGS OF THE 13TH INTERNATIONAL CONFERENCE ON UBIQUITOUS INFORMATION MANAGEMENT AND COMMUNICATION (IMCOM) 2019(2019)
摘要
In today's networked world, graph data is becoming increasingly more ubiquitous as the complexities, layers and hierarchies of real life data demand to be represented in a structured manner. The goal of graph mining is to extract frequent and interesting subgraph from large graph databases. It is even more significant to perform correlation analysis within these frequent subgraphs, as such relations may provide us with valuable information. However, unfortunately much work has not been done in this field even though its necessity is enormous. In this paper, we propose two measures that help us discover such correlation among frequent subgraphs. Our measures are based on the observation that elements in graphs exhibit the tendency to occur both connected and disconnected. Evaluation results show the effectiveness and practicality of our measures in real life datasets.
更多查看译文
关键词
Data mining,Graph mining,Correlation discovery,Information management,Information processing management
AI 理解论文
溯源树
样例
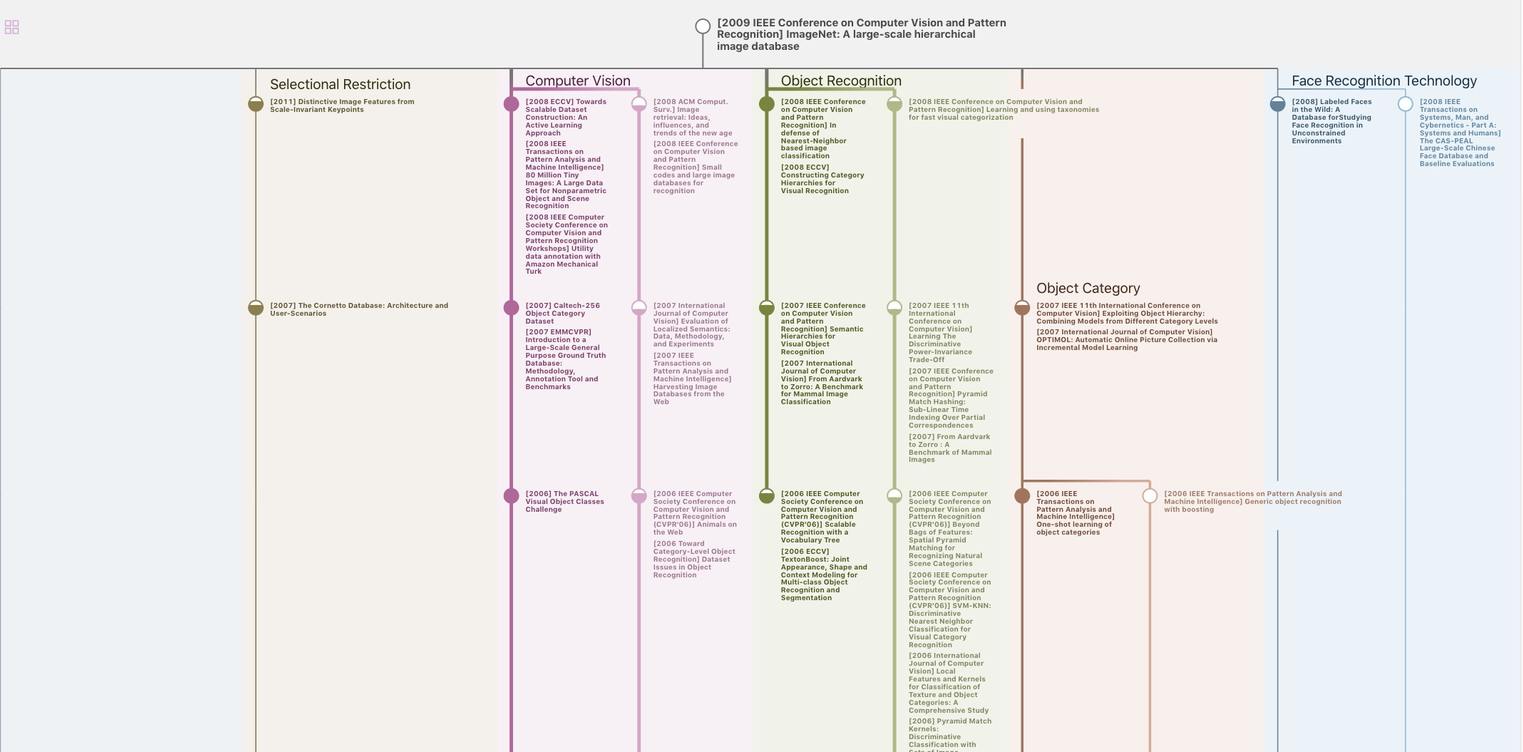
生成溯源树,研究论文发展脉络
Chat Paper
正在生成论文摘要