Revisiting Metric Learning for Few-Shot Image Classification
Neurocomputing(2020)
摘要
The goal of few-shot learning is to recognize new visual concepts with just a few amount of labeled samples in each class. Recent effective metric-based few-shot approaches employ neural networks to learn a feature similarity comparison between query and support examples. However, the importance of feature embedding, i.e., exploring the relationship among training samples, is neglected. In this work, we present a simple yet powerful baseline for few-shot classification by emphasizing the importance of feature embedding. Specifically, we revisit the classical triplet network from deep metric learning, and extend it into a deep K-tuplet network for few-shot learning, utilizing the relationship among the input samples to learn a general representation learning via episode-training. Once trained, our network is able to extract discriminative features for unseen novel categories and can be seamlessly incorporated with a non-linear distance metric function to facilitate the few-shot classification. Our result on the miniImageNet benchmark outperforms other metric-based few-shot classification methods. More importantly, when evaluated on completely different datasets (Caltech-101, CUB-200, Stanford Dogs and Cars) using the model trained with miniImageNet, our method significantly outperforms prior methods, demonstrating its superior capability to generalize to unseen classes.
更多查看译文
关键词
Few-shot learning,Metric learning,Feature representation,Deep learning,
AI 理解论文
溯源树
样例
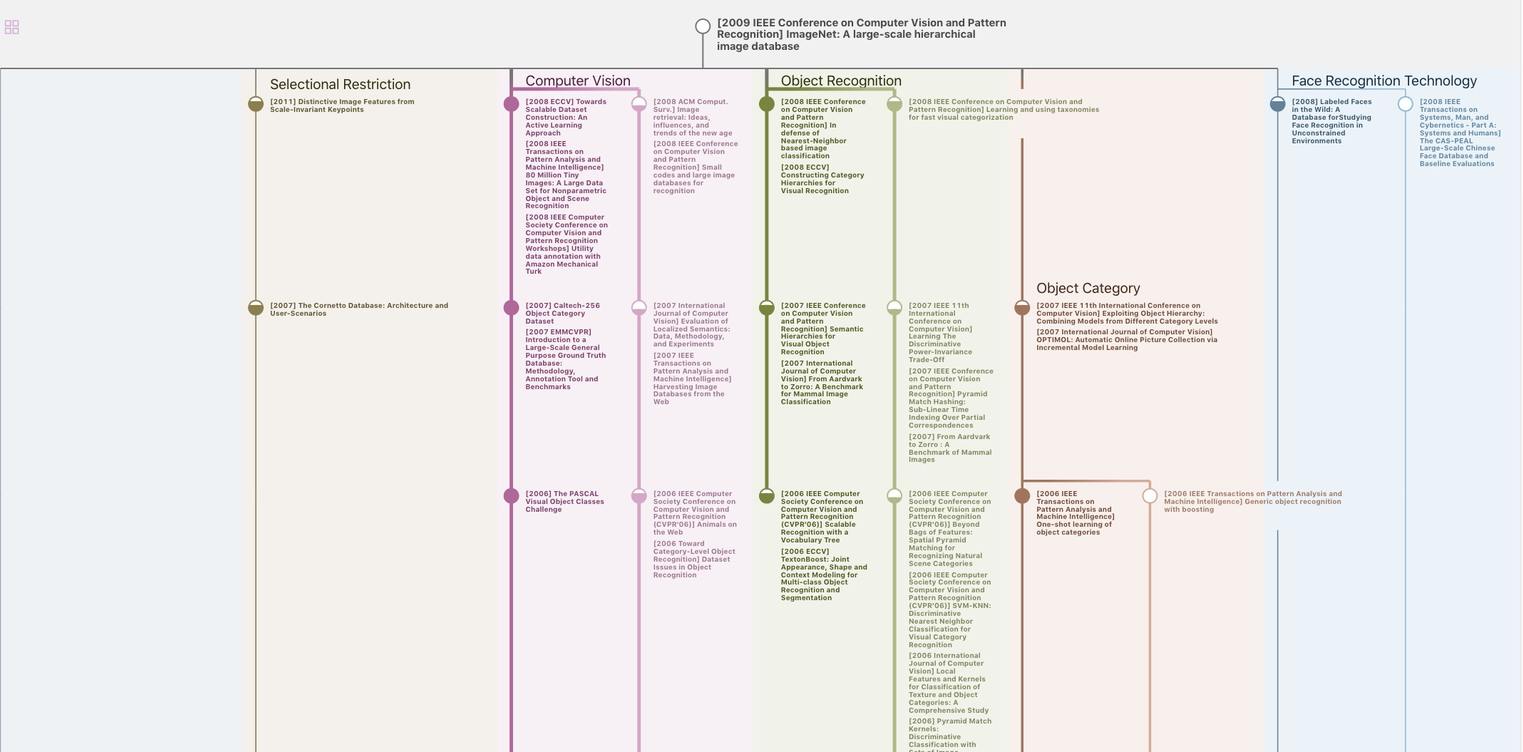
生成溯源树,研究论文发展脉络
Chat Paper
正在生成论文摘要