A Deep Learning Approach for Real-Time 3D Human Action Recognition from Skeletal Data
IMAGE ANALYSIS AND RECOGNITION, ICIAR 2019, PT I(2019)
摘要
We present a new deep learning approach for real-time 3D human action recognition from skeletal data and apply it to develop a vision-based intelligent surveillance system. Given a skeleton sequence, we propose to encode skeleton poses and their motions into a single RGB image. An Adaptive Histogram Equalization (AHE) algorithm is then applied on the color images to enhance their local patterns and generate more discriminative features. For learning and classification tasks, we design Deep Neural Networks based on the Densely Connected Convolutional Architecture (DenseNet) to extract features from enhanced-color images and classify them into classes. Experimental results on two challenging datasets show that the proposed method reaches state-of-the-art accuracy, whilst requiring low computational time for training and inference. This paper also introduces CEMEST, a new RGB-D dataset depicting passenger behaviors in public transport. It consists of 203 untrimmed real-world surveillance videos of realistic normal and anomalous events. We achieve promising results on real conditions of this dataset with the support of data augmentation and transfer learning techniques. This enables the construction of real-world applications based on deep learning for enhancing monitoring and security in public transport.
更多查看译文
关键词
Action recognition,Skeletal data,Enhanced-SPMF,DenseNet
AI 理解论文
溯源树
样例
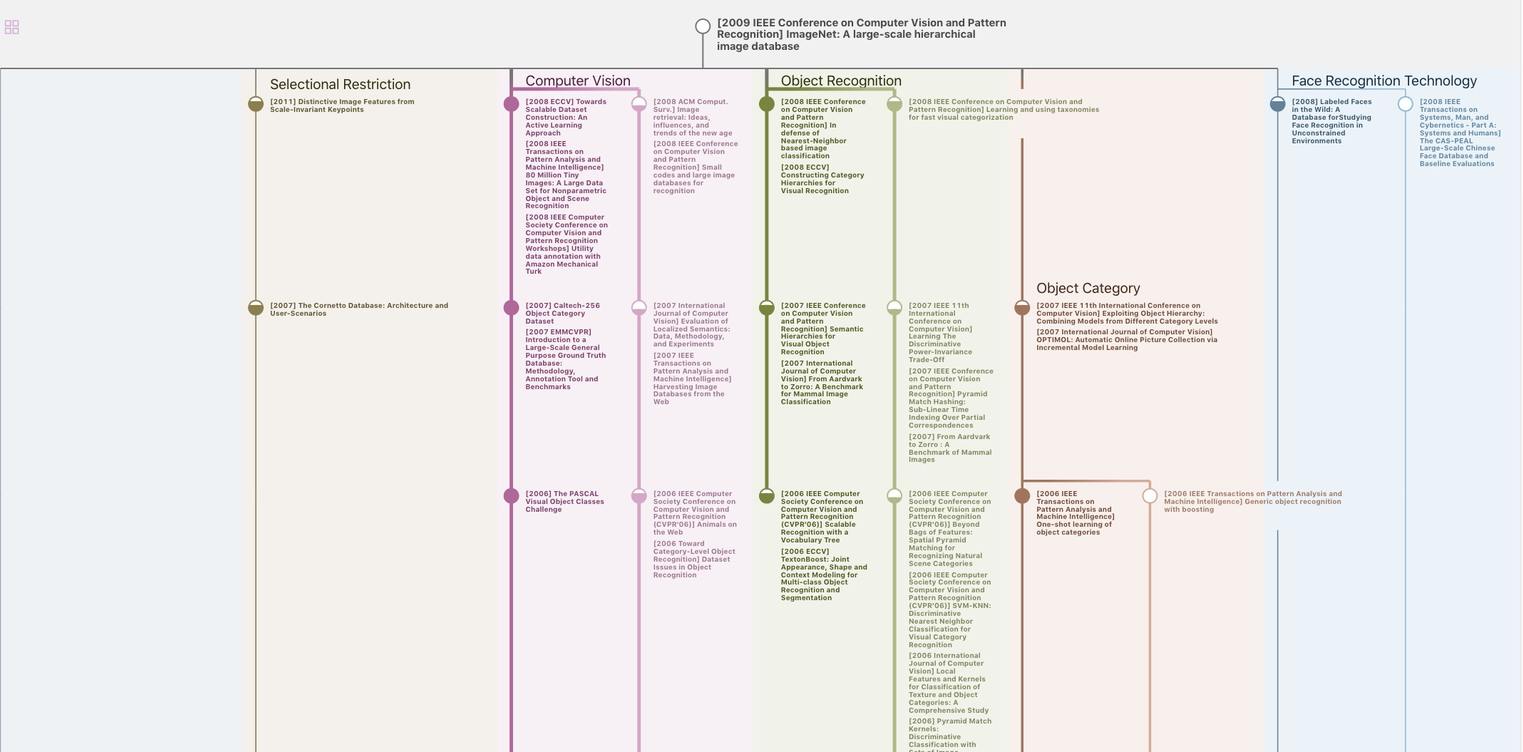
生成溯源树,研究论文发展脉络
Chat Paper
正在生成论文摘要