Tracking the Evolution of Financial Time Series Clusters
Proceedings of the 5th Workshop on Data Science for Macro-modeling with Financial and Economic Datasets(2019)
摘要
Nowadays, a huge amount of applications exist that natively adopt a data-streaming model to represent highly dynamic phenomena. A challenging application is constituted by data from the stock market, where the stock prices are naturally modeled as data streams that fluctuate very much and remain meaningful only for short amounts of time. In this paper we present a technique to track evolving clusters of financial time series, with the aim of constructing reliable models for this highly dynamic application. In our technique the clustering over a set of time series is iterated over time through sliding windows and, at each iteration, the differences between the current clustering and the previous one are studied to determine those changes that are "significant" with respect to the application. For example, in the financial domain, if a company that has belonged to the same cluster for a certain amount of time moves to another cluster, this may be a signal of a significant change in its economical or financial situation.
更多查看译文
关键词
Financial Time Series, Tracking Data-Stream Clusters
AI 理解论文
溯源树
样例
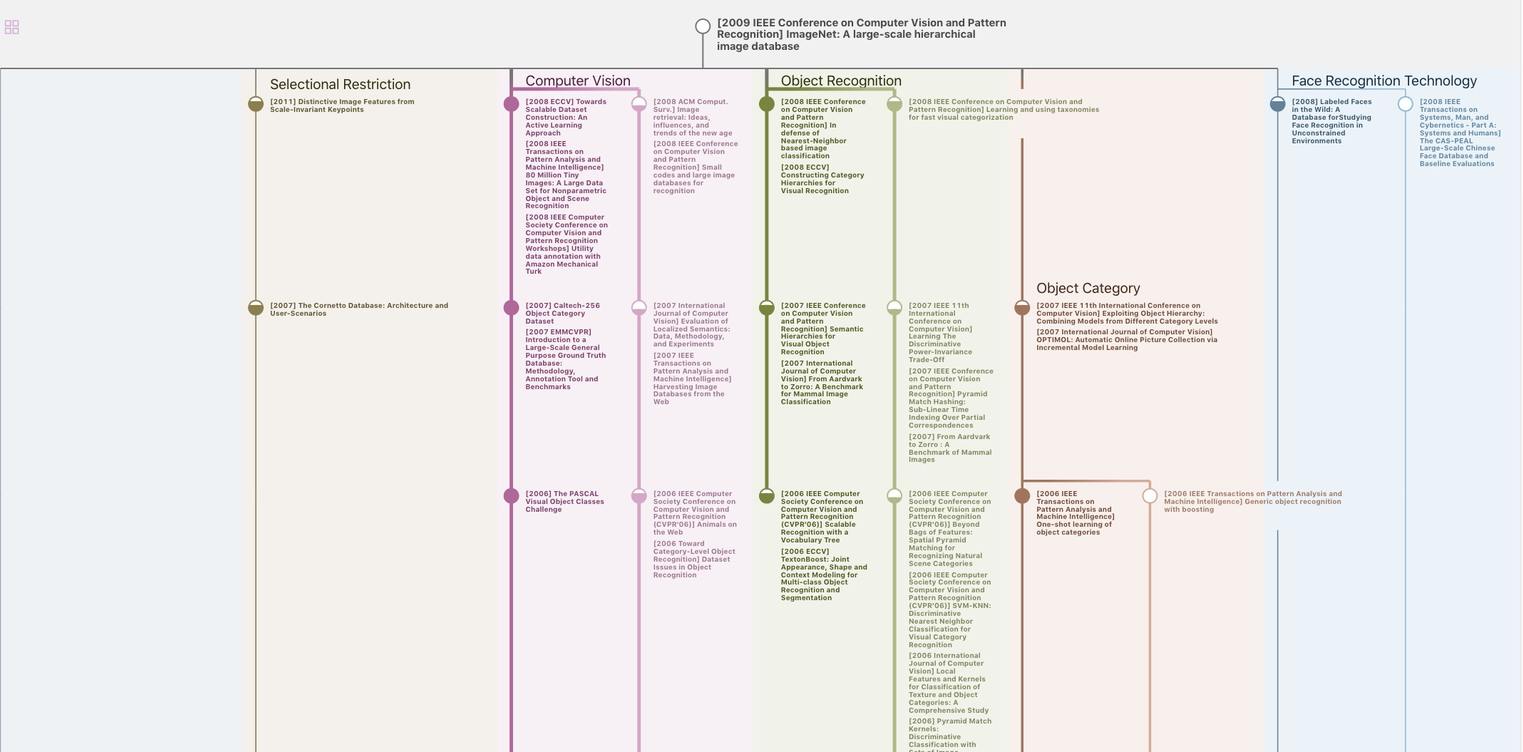
生成溯源树,研究论文发展脉络
Chat Paper
正在生成论文摘要