Bayesian Analysis of Longitudinal Quality of Life Measures with Informative Missing Data Using a Selection Model.
Statistical methods in medical research(2019)
Abstract
Health-related quality of life consists of multi-dimensional measurements of physical and mental health domains. Health-related quality of life is often followed up to evaluate efficacy of treatments in clinical studies. During the follow-up period, a missing data problem inevitably arises. When missing data occur for reasons related to poor health-related quality of life, a complete-case only analysis can lead to invalid inferences. We propose a Bayesian approach to analyze longitudinal moderate to high-dimensional multivariate outcome data in the presence of non-ignorable missing data. To account for non-ignorable missing data, we employ a selection model for the joint likelihood factorization where we apply Bayesian spike and slab variable selection in the missing data mechanism to detect informative factors among multiple outcomes. We model the relationship between multiple outcomes and covariates using linear mixed effects models where multiple outcome correlations are captured by a hierarchical structure. We conduct simulation studies to evaluate the performance of the proposed method compared with the conventional last observation carried forward approach. We use a motivating example that originates from a longitudinal study of quality of life in gastric cancer patients who underwent distal gastrectomy. In this application, we demonstrate that our proposed method can offer efficiency gain in the marginal associations and provide the associations between outcomes and the absence of patients' information.
MoreTranslated text
Key words
Multivariate,health-related quality of life,non-ignorable missingness,longitudinal study,selection model,sensitivity analysis
AI Read Science
Must-Reading Tree
Example
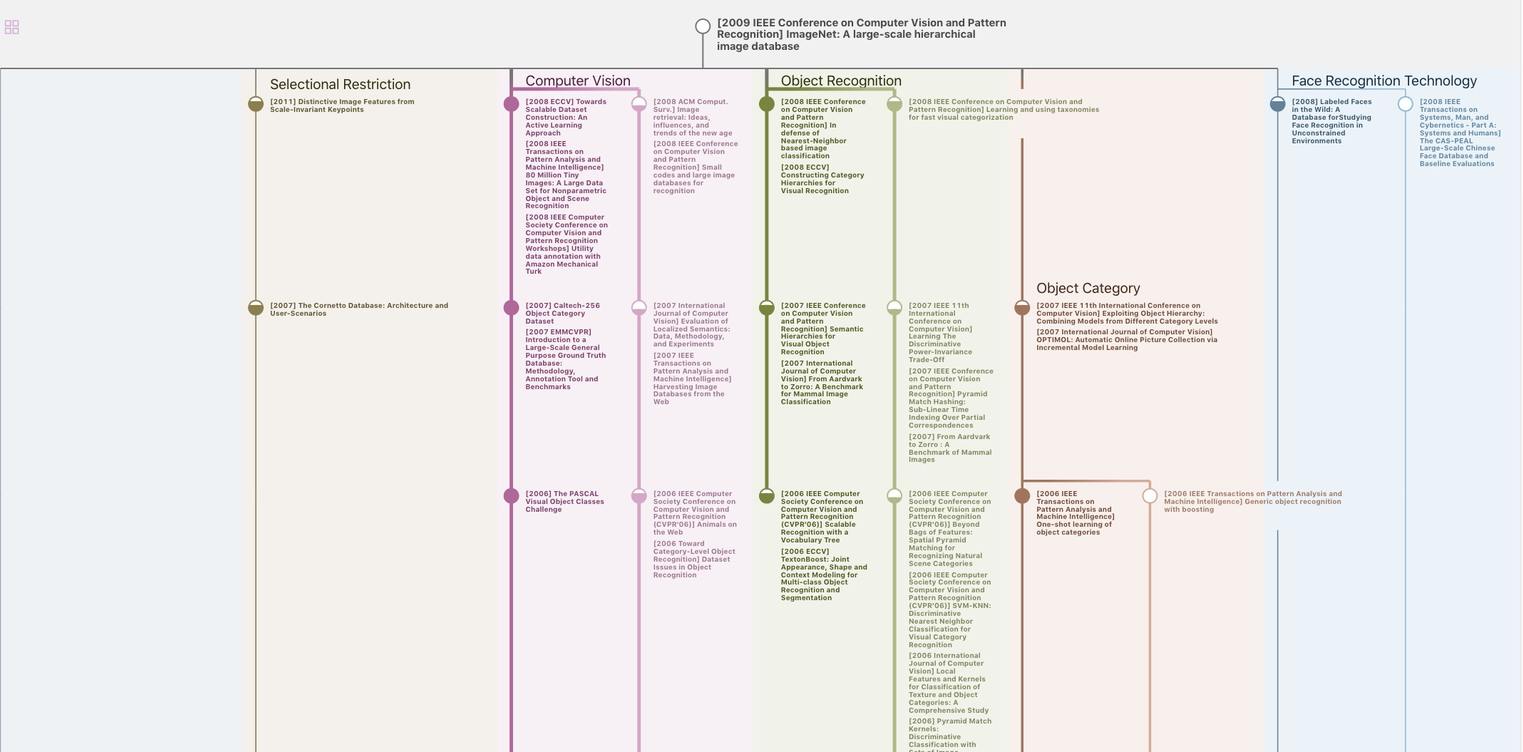
Generate MRT to find the research sequence of this paper
Chat Paper
Summary is being generated by the instructions you defined