Automatic Prediction Of Leave Chemical Compositions Based On Nir Spectroscopy With Machine Learning
INTERNATIONAL JOURNAL OF ROBOTICS & AUTOMATION(2019)
摘要
Near-infrared (NIR) spectroscopy is an automatic technique to detect spectra of leaves, and it has been a useful tool to extract the desired chemical information when combined with other multivariate calibration techniques. A least angle regression (LAR) model was proposed to predict automatically the content of chemical compositions based on NIR spectroscopy in the tobacco industry. The other two models, least squares support vector machines (LS-SVM) and partial least squares (PLS), were used for comparison. Results show that the proposed LAR model is much superior to the other two models on prediction accuracy and operational efficiency. In terms of model interpretability, the LAR model has a good ability to reveal the most interrelated wavenumbers of NIR spectra in predicting the content of chemical compositions for its great sparse characteristic, while the PLS model is inferior in such capacity, and the LS-SVM is not able to interpret the correlation due to its nonlinear feature. Therefore, the LAR model is feasible for fast and accurate quantitative analysis for NIR spectra of tobacco leaves.
更多查看译文
关键词
Least angle regression, least squares support vector machines, partial least squares regression, near-infrared spectroscopy, chemical composition
AI 理解论文
溯源树
样例
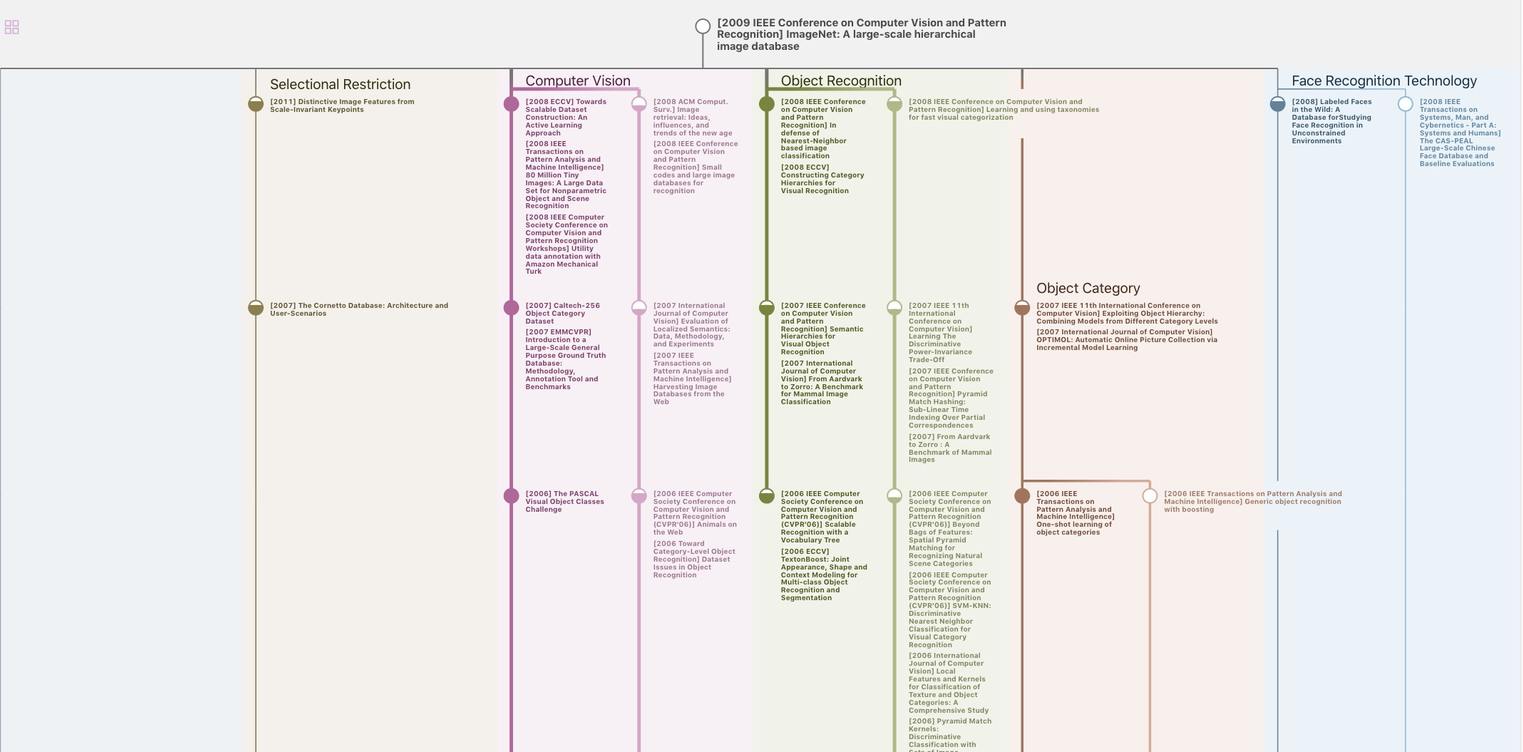
生成溯源树,研究论文发展脉络
Chat Paper
正在生成论文摘要