Feature Selection for Surrogate Model-Based Optimization
Proceedings of the Genetic and Evolutionary Computation Conference Companion(2019)
摘要
We propose a hybridization approach called Regularized-Surrogate-Optimization (RSO) aimed at overcoming difficulties related to high-dimensionality. It combines standard Kriging-based SMBO with regularization techniques. The employed regularization methods use the least absolute shrinkage and selection operator (LASSO). An extensive study is performed on a set of artificial test functions and two real-world applications: the electrostatic precipitator problem and a multilayered composite design problem. Experiments reveal that RSO requires significantly less time than Kriging to obtain comparable results. The pros and cons of the RSO approach are discussed and recommendations for practitioners are presented.
更多查看译文
关键词
Optimization,Surrogates,Modeling,Dimensionality Reduction,Real-World
AI 理解论文
溯源树
样例
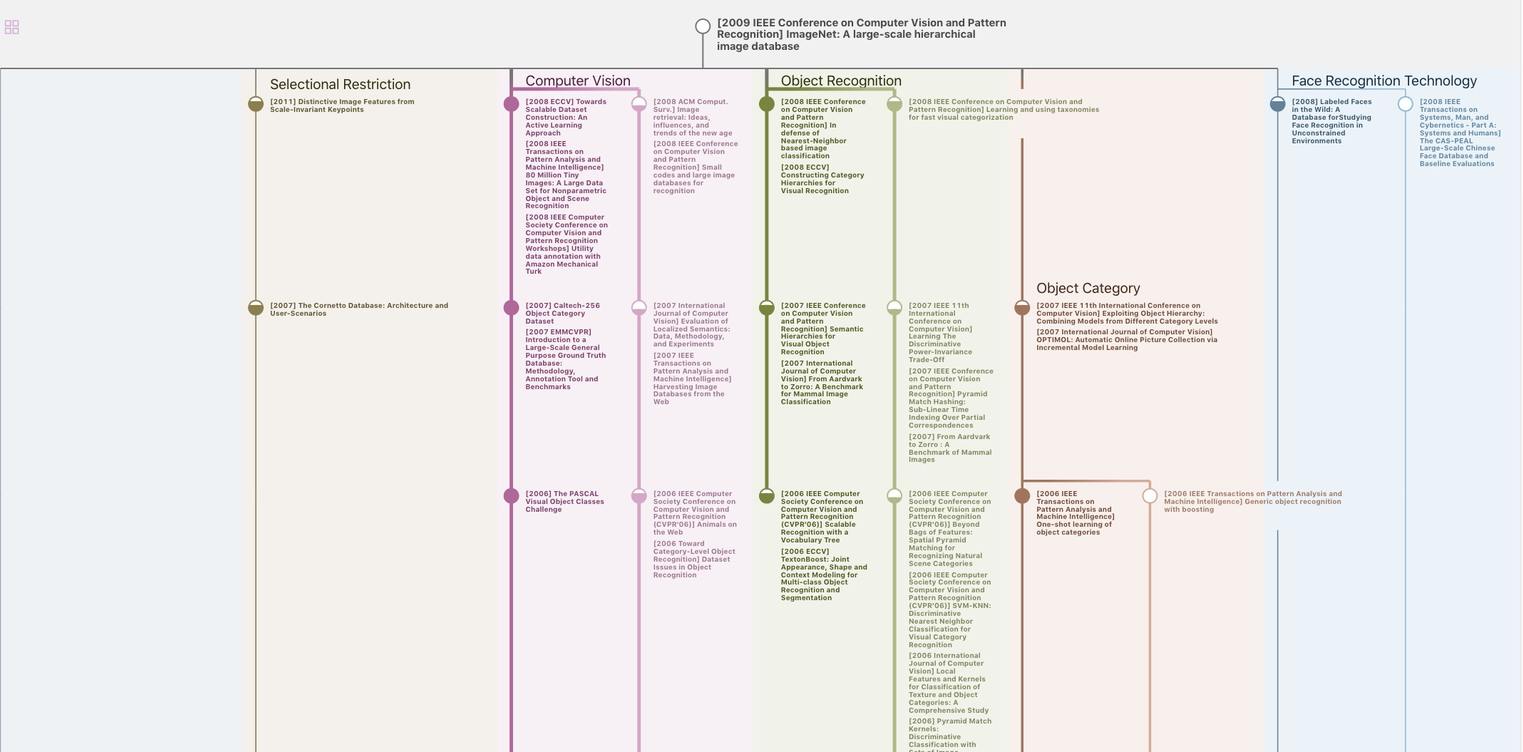
生成溯源树,研究论文发展脉络
Chat Paper
正在生成论文摘要