Noisy multiobjective black-box optimization using bayesian optimization.
GECCO(2019)
摘要
Expensive black-box problems are usually optimized by Bayesian Optimization (BO) since it can reduce evaluation costs via cheaper surrogates. The most popular model used in Bayesian Optimization is the Gaussian process (GP) whose posterior is based on a joint GP prior built by initial observations, so the posterior is also a Gaussian process. Observations are often not noise-free, so in most of these cases, a noisy transformation of the objective space is observed. Many single objective optimization algorithms have succeeded in extending efficient global optimization (EGO) to noisy circumstances, while ParEGO fails to consider noise. In order to deal with noisy expensive black-box problems, we extending ParEGO to noisy optimization according to adding a Gaussian noisy error while approximating the surrogate. We call it noisy-ParEGO and results of S-metric indicate that the algorithm works well on optimizing noisy expensive multiobjective black-box problems.
更多查看译文
关键词
black-box optimization, expensive multiobjective optimization, Gaussian Process, Gaussian noise, ParEGO
AI 理解论文
溯源树
样例
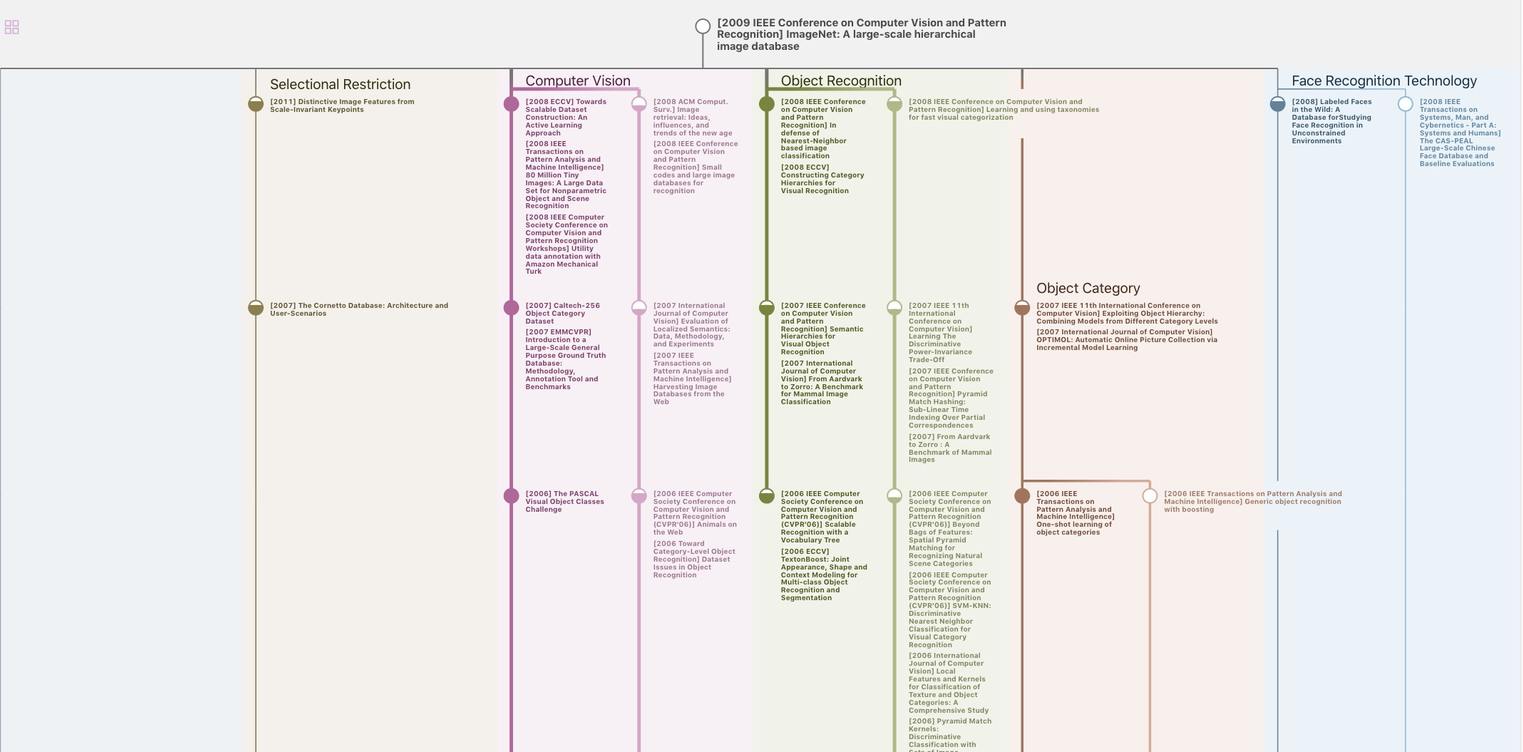
生成溯源树,研究论文发展脉络
Chat Paper
正在生成论文摘要