Balancing exploration and exploitation in multiobjective batch bayesian optimization.
GECCO(2019)
摘要
Many applications such as hyper-parameter tunning in Machine Learning can be casted to multiobjective black-box problems and it is challenging to optimize them. Bayesian Optimization (BO) is an effective method to deal with black-box functions. This paper mainly focuses on balancing exploration and exploitation in multi-objective black-box optimization problems by multiple samplings in BBO. In each iteration, multiple recommendations are generated via two different trade-off strategies respectively the expected improvement (EI) and a multiobjective framework with the mean and variance function of the GP posterior forming two conflict objectives. We compare our algorithm with ParEGO by running on 12 test functions. Hypervolume (HV, also known as S-metric) results show that our algorithm works well in exploration-exploitation trade-off for multiobjective black-box optimization problems.
更多查看译文
关键词
expensive multiobjective optimization, batch Bayesian optimization, Gaussian Process, exploration and exploitation, ParEGO
AI 理解论文
溯源树
样例
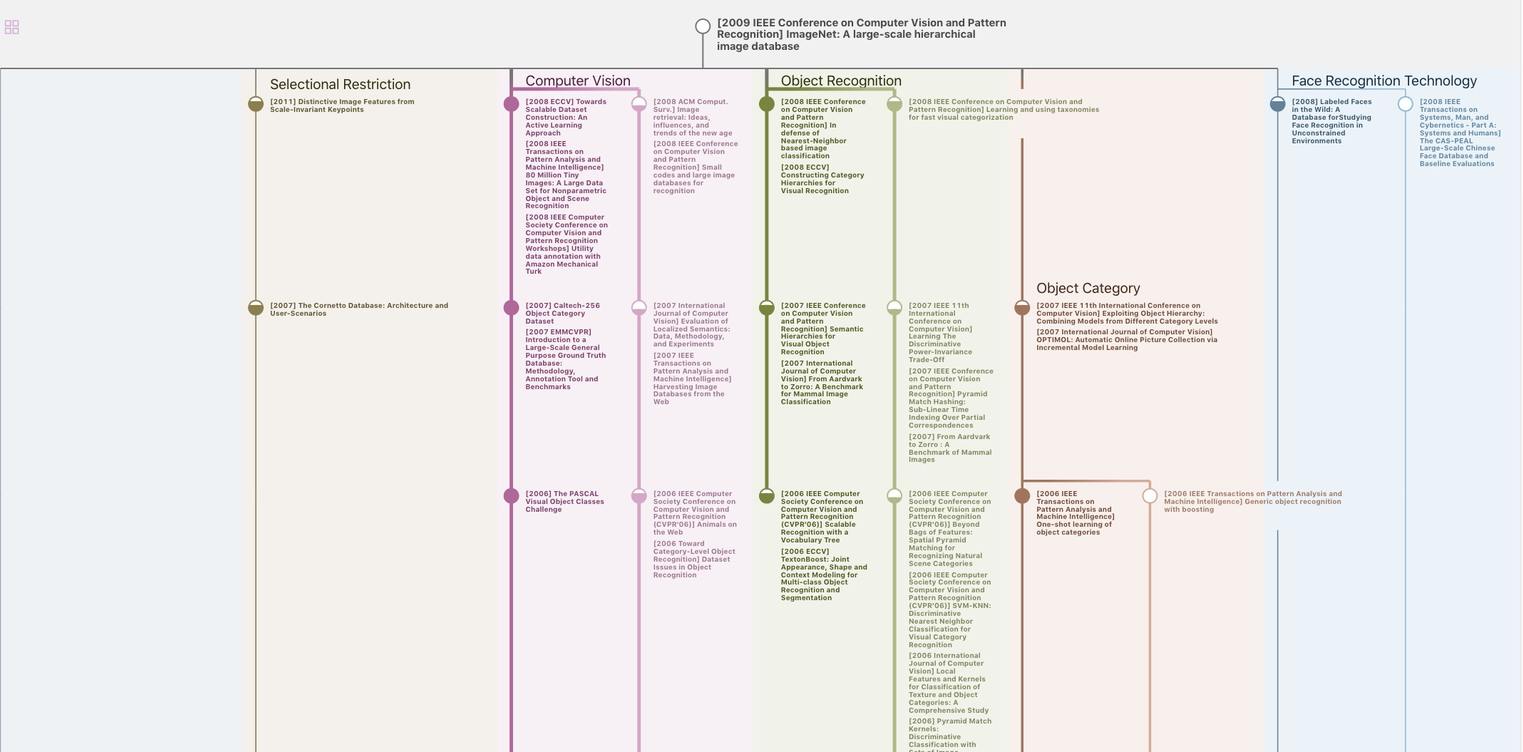
生成溯源树,研究论文发展脉络
Chat Paper
正在生成论文摘要