Deep Network Anatomy Segmentation with Limited Annotations using Auxiliary Labels
2019 IEEE 16th International Symposium on Biomedical Imaging (ISBI 2019)(2019)
摘要
Deep convolutional neural networks (CNNs) have shown impressive performance in anatomy segmentation that are close to the state of the art atlas-based segmentation method. On one hand CNNs have 20x faster predictions than atlas-based segmentation. However, one of the main holdbacks of CNN's advancement is that it's training requires large amount of annotated data. This is a costly hurdle as annotation is time consuming and requires expensive medical expertise. The goal of this work is to reach state of the art segmentation performance using the minimum amount of expensive manual annotations. Recent studies show that auxiliary segmentations can be used together with manual annotations to improve CNN learning. To make this learning scheme more effective, we propose an image selection algorithm that wisely chooses images for manual annotation for producing more accurate auxiliary segmentations and a quality control algorithm that excludes poor quality auxiliary segmentations from CNN training. We perform extensive experiments over chest CT dataset by varying the number of manual annotations used for atlas-based methods and by varying the number of auxiliary segmentations to train the CNN. Our results show that CNN trained with auxiliary segmentations achieve higher dice of 0.76 vs 0.58 when trained with few accurate manual segmentations. Moreover, training with 100 or more auxiliary segmentations, the CNN always outperforms atlas-based method. Finally, when carefully selecting single atlas for producing auxiliary segmentations and controlling the quality of auxiliary segmentations, the trained CNN archives high average dice of 0.72 vs 0.62 when using a randomly selected image for manual annotation with all auxiliary segmentations.
更多查看译文
关键词
Deep network,Segmentation,Auxiliary segmentations,Multi-Atlas
AI 理解论文
溯源树
样例
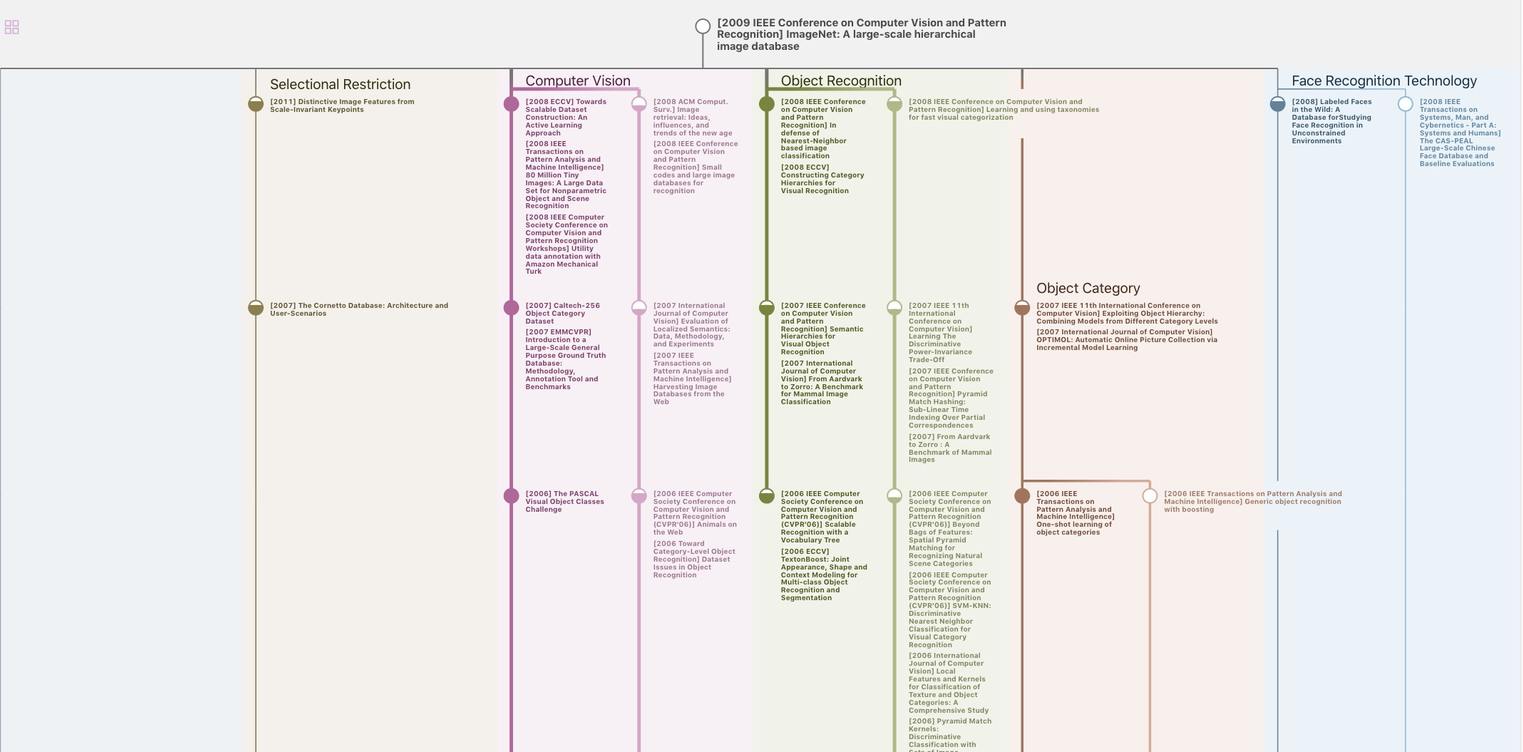
生成溯源树,研究论文发展脉络
Chat Paper
正在生成论文摘要