Learning to Segment the Lung Volume from CT Scans Based on Semi-Automatic Ground-Truth
2019 IEEE 16TH INTERNATIONAL SYMPOSIUM ON BIOMEDICAL IMAGING (ISBI 2019)(2019)
摘要
Lung volume segmentation is a key step in the design of Computer-Aided Diagnosis systems for automated lung pathology analysis. However, isolating the lung from CT volumes can be a challenging process due to considerable deformations and the potential presence of pathologies. Convolutional Neural Networks (CNN) are effective tools for modeling the spatial relationship between lung voxels. Unfortunately, they typically require large quantities of annotated data, and manually delineating the lung from volumetric CT scans can be a cumbersome process. We propose to train a 3D CNN to solve this task based on semi-automatically generated annotations. For this, we introduce an extension of the well-known V-Net architecture that can handle higher-dimensional input data. Even if the training set labels are noisy and contain errors, our experiments show that it is possible to learn to accurately segment the lung relying on them. Numerical comparisons on an external test set containing lung segmentations provided by a medical expert demonstrate that the proposed model generalizes well to new data, reaching an average 98.7% Dice coefficient. The proposed approach results in a superior performance with respect to the standard V-Net model, particularly on the lung boundary.
更多查看译文
关键词
Lung Volume Segmentation, CT scans
AI 理解论文
溯源树
样例
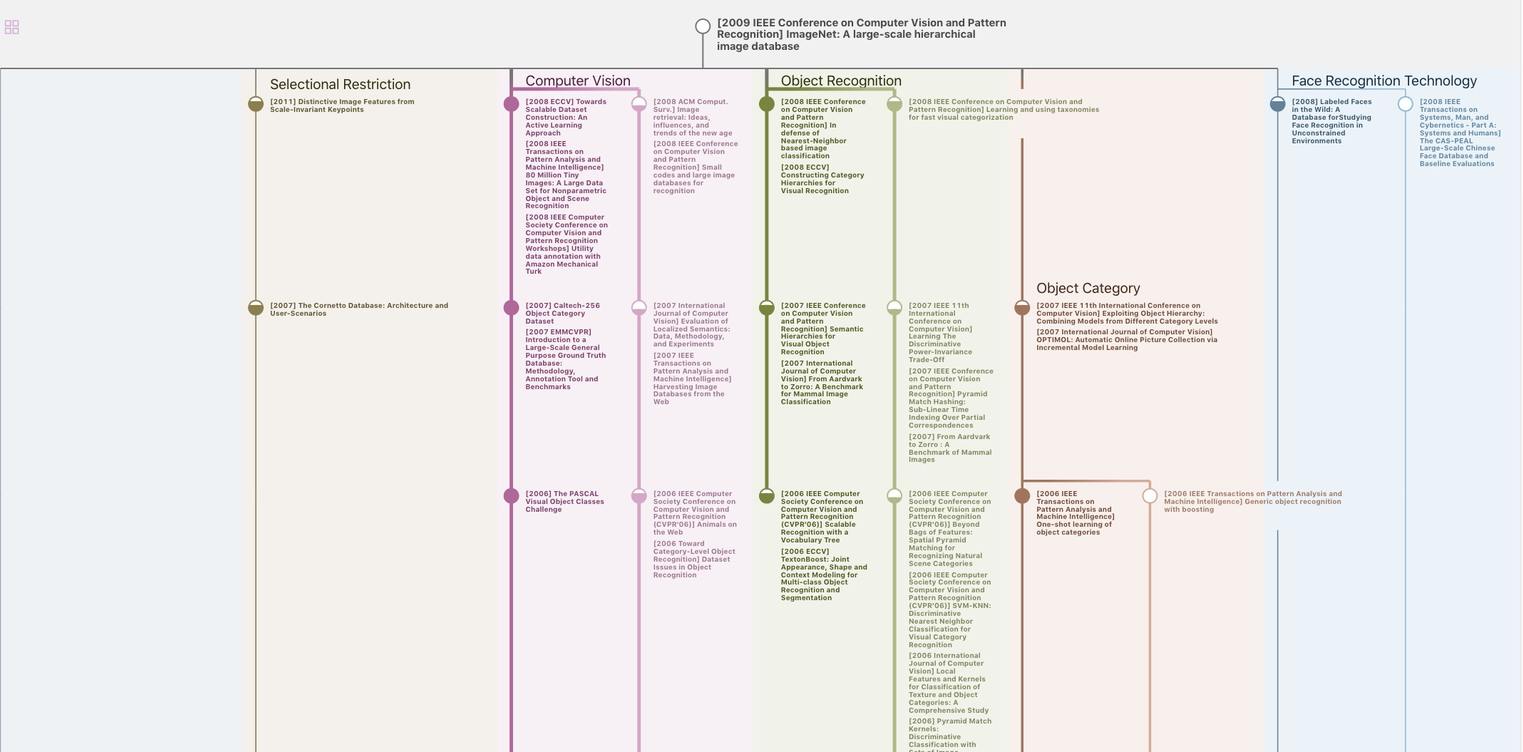
生成溯源树,研究论文发展脉络
Chat Paper
正在生成论文摘要