Deep mutual learning for visual tracking
Proceedings of the ACM Turing Celebration Conference - China(2019)
摘要
In this work, we propose a novel deep learning method to improve the accuracy and the speed of particle filter based object trackers. The main contributions include two aspects. First, to enhance the discrimination of feature representations, we propose two identical CNNs, each of which shares information from the other by introducing the Kullback-Leibler (KL) loss. Second, in order to boost the speed of trackers, we introduce the region of interest (ROI) align, and optimize the structure-identical networks with knowledge distillation and deep mutual learning. It makes the forward propagation only calculate once instead of hundreds of iterations. Experimental results on the OTB2015 and VOT2015 benchmarks demonstrate that our method performs better than several state-of-the-art algorithms.
更多查看译文
关键词
deep mutual learning, knowledge distillation, particle filter object tracking
AI 理解论文
溯源树
样例
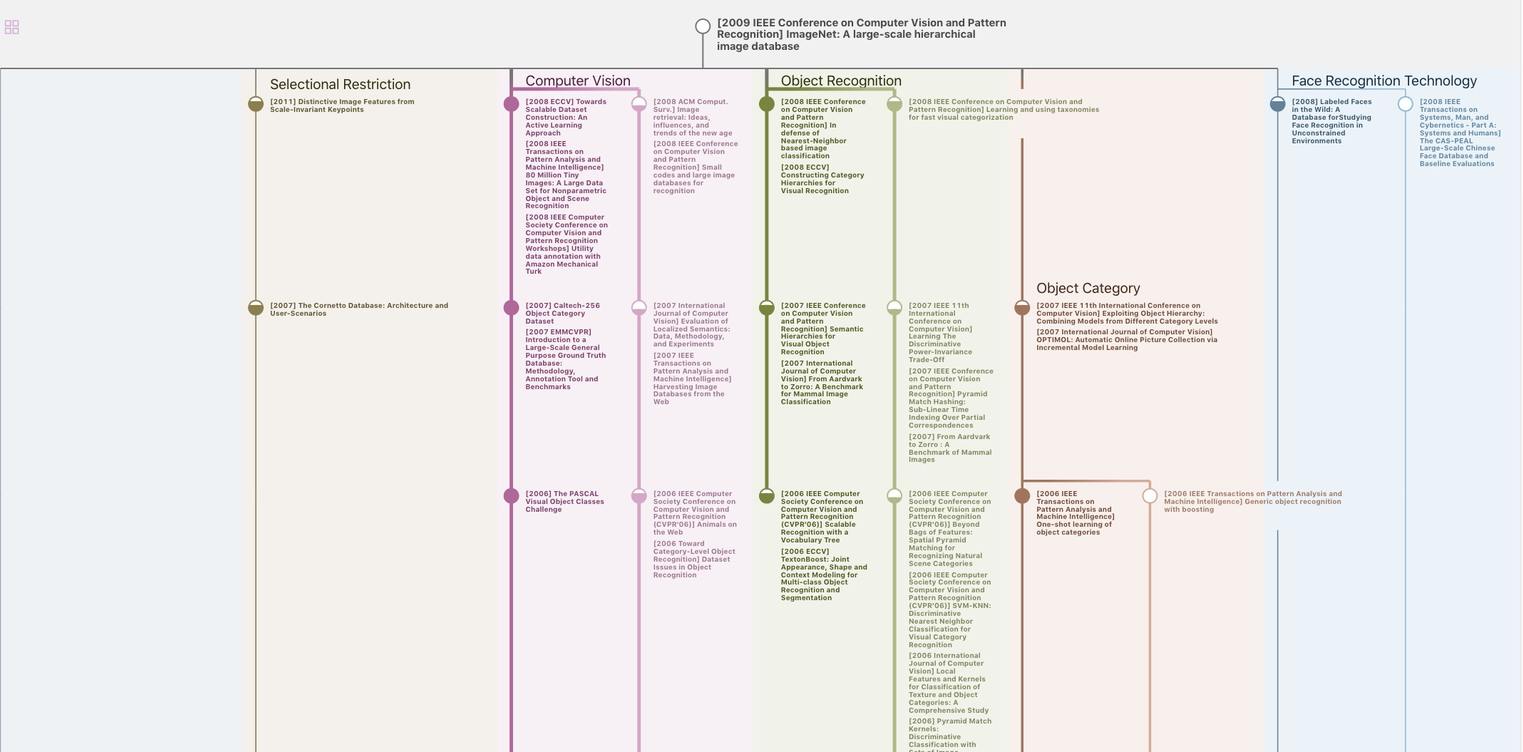
生成溯源树,研究论文发展脉络
Chat Paper
正在生成论文摘要