A Novel Method Based on Entity Relationship for Online Transaction Fraud Detection.
Proceedings of the ACM Turing Celebration Conference - China(2019)
摘要
Fraud detection is the focus of research in Internet financial domain. The methods of both expert rules and machine learning detect fraud by seeking commonness among groups and individual differences between normal and abnormal transactions. However, they don't consider the relationship among attributes of transaction entities that may affect the precision of fraud detection. Different from the grid data, each node in relational network has different numbers of neighbors nodes, and the arrangement among them is disordered. Thus, this paper adds the relationship of transaction entities to machine learning model, which can effectively connect the graph domain and attributes space. First, the relational network between the transaction entity and the attribute entity is extracted from the transaction record. It is essentially a heterogeneous non-connected sparse bipartite graph with characteristics on nodes. However, the heterogeneous information network can not be uniformly characterized, and we should find and utilize the hidden relationship among the transaction entities we focused. Thus, Node Shrinkage Homogenization Algorithm is proposed for homogenization. Based on this homogeneous network, we put forward the method of graph-based Neighborhood Information Aggregation Gradient Boosting Decision Tree (NIAGBDT), so that the transaction features are integrated from its neighbor through the relational network. The experiments show that compared with current state of the art approaches, our algorithm has a significant improvement more than ten percent.
更多查看译文
AI 理解论文
溯源树
样例
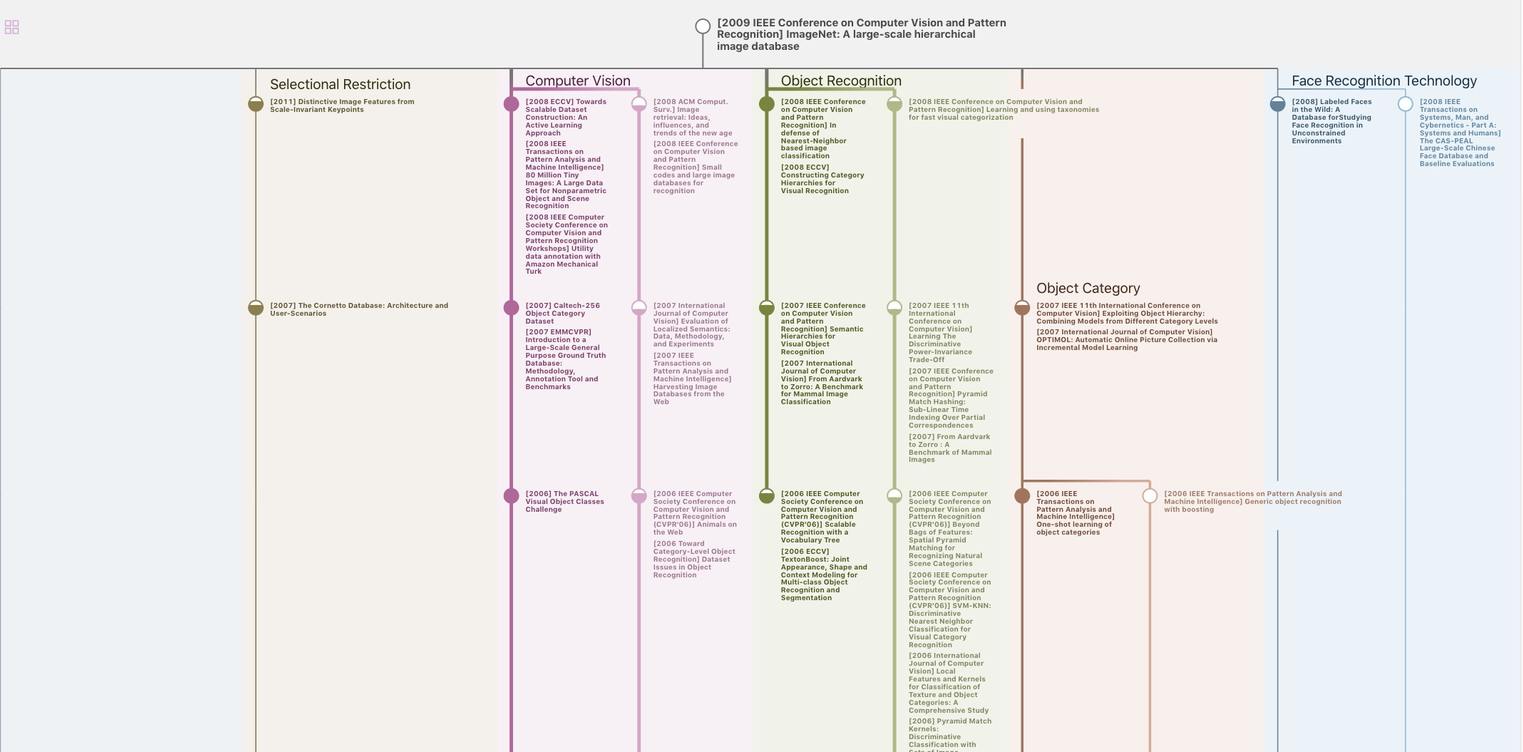
生成溯源树,研究论文发展脉络
Chat Paper
正在生成论文摘要