Online Topology Inference from Streaming Stationary Graph Signals
2019 IEEE Data Science Workshop (DSW)(2019)
摘要
We address the problem of online topology inference from streaming nodal observations of graph signals generated by linear diffusion dynamics on the sought graph. To that end, we leverage the stationarity of the signals and use the so-called graph-shift operator (GSO) as a matrix representation of the graph. Under this model, estimated covariance eigenvectors obtained from streaming independent graph signals diffused on the sought network are a valid estimator of the GSO's spectral templates. We develop an ADMM algorithm to find a sparse and structurally admissible GSO given the eigenvectors estimate. Then, we propose an online scheme that upon sensing new diffused observations, efficiently updates eigenvectors (thus makes more accurate on expectation) and performs only one or a few iterations of the mentioned ADMM until the new data is observed. Numerical tests illustrate the effectiveness of the proposed topology inference approach in recovering large scale graphs, adapting to streaming information, and accommodating changes in the sought network.
更多查看译文
关键词
Network topology inference,graph signal processing,diffusion process,online algorithm.
AI 理解论文
溯源树
样例
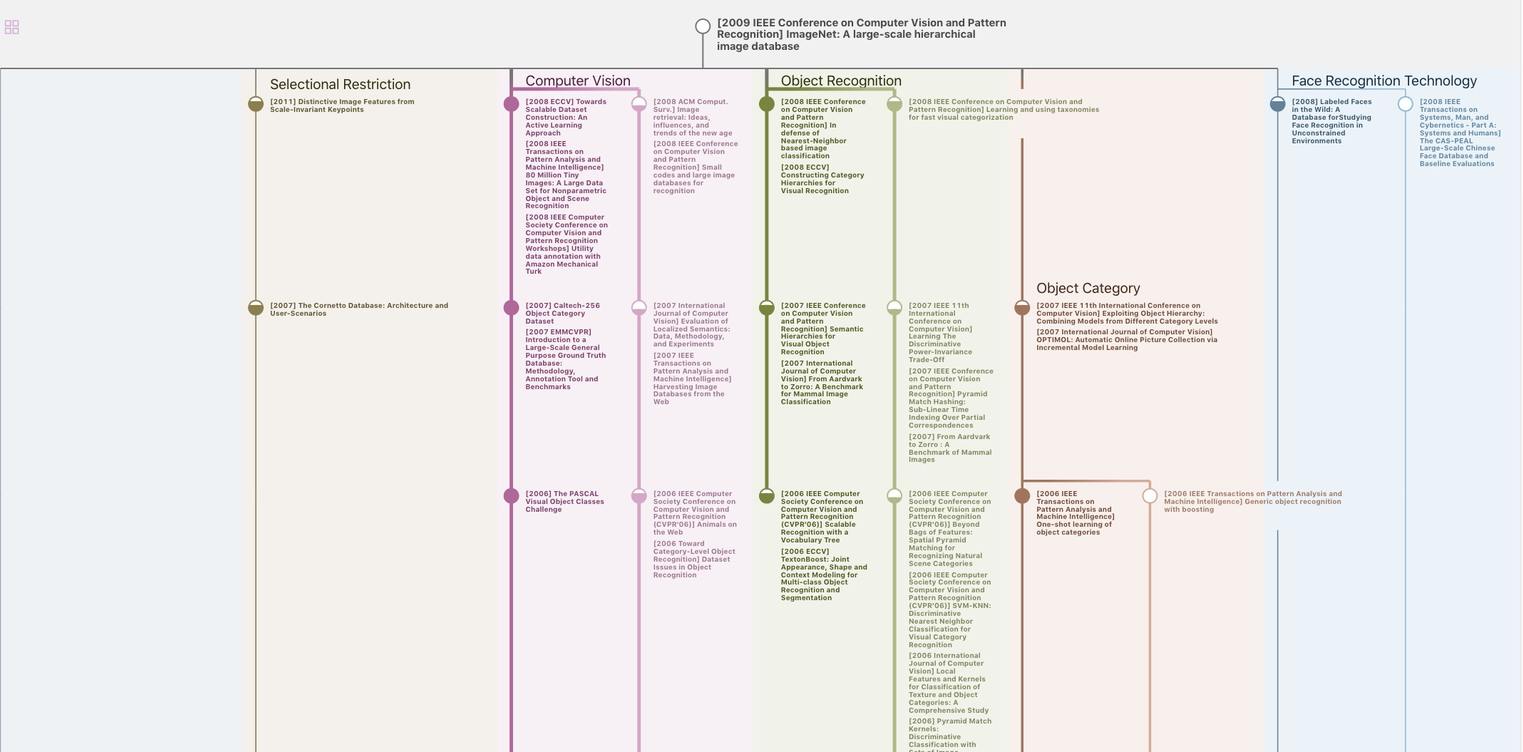
生成溯源树,研究论文发展脉络
Chat Paper
正在生成论文摘要