Training Streaming Factorization Machines with Alternating Least Squares
Proceedings of the 42nd International ACM SIGIR Conference on Research and Development in Information Retrieval(2019)
摘要
Factorization Machines (FM) have been widely applied in industrial applications for recommendations. Traditionally FM models are trained in batch mode, which entails training the model with large datasets every few hours or days. Such training procedure cannot capture the trends evolving in real time with large volume of streaming data. In this paper, we propose an online training scheme for FM with the alternating least squares (ALS) technique, which has comparable performance with existing batch training algorithms. We incorporate an online update mechanism to the model parameters at the cost of storing a small cache. The mechanism also stabilizes the training error more than a traditional online training technique like stochastic gradient descent (SGD) as data points come in, which is crucial for real-time applications. Experiments on large scale datasets validate the efficiency and robustness of our method.
更多查看译文
关键词
alternating least squares, factorization machines, online learning
AI 理解论文
溯源树
样例
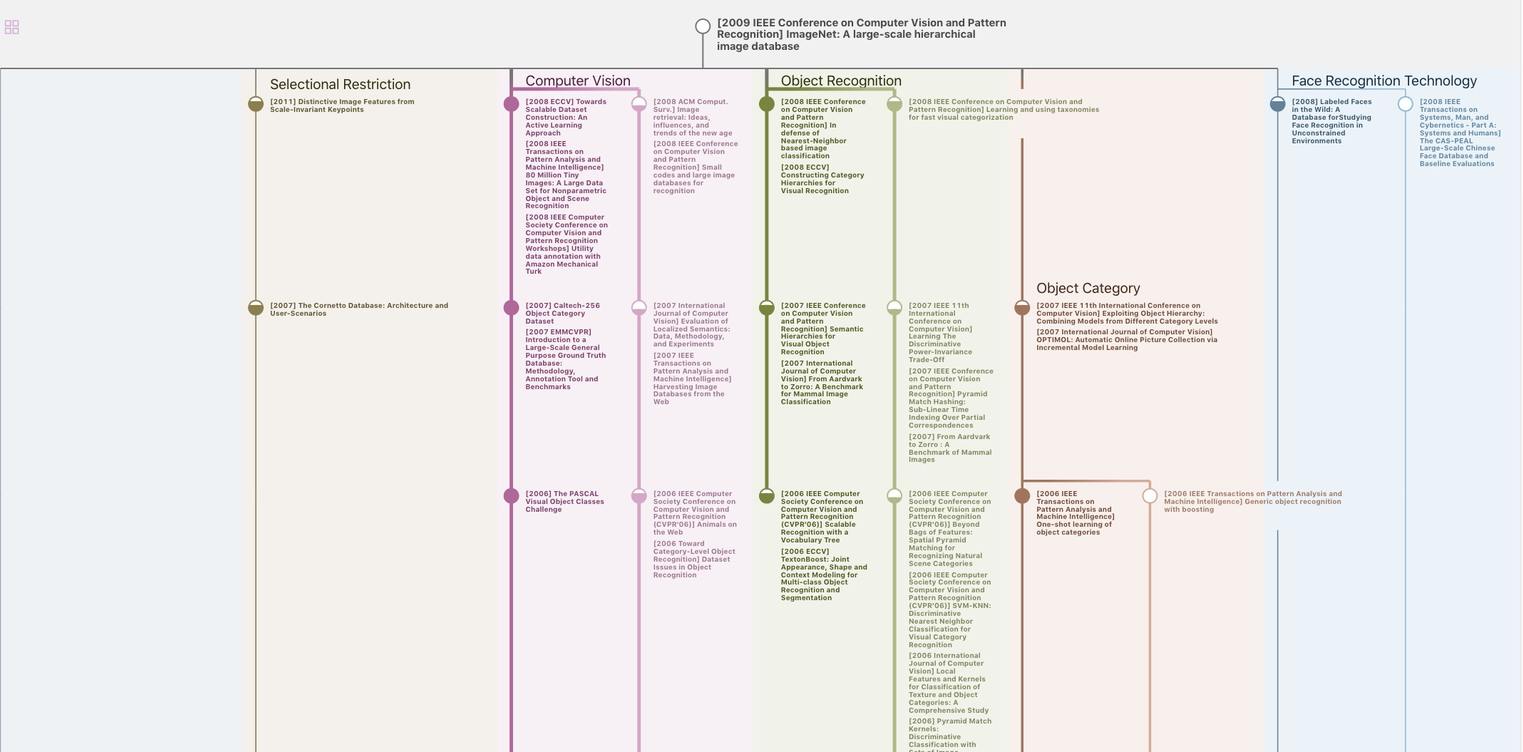
生成溯源树,研究论文发展脉络
Chat Paper
正在生成论文摘要