Bridging Gaps: Predicting User and Task Characteristics from Partial User Information
Proceedings of the 42nd International ACM SIGIR Conference on Research and Development in Information Retrieval(2019)
摘要
Interactive information retrieval (IIR) researchers often conduct laboratory studies to understand the relationship between people seeking information and information retrieval systems. They develop extensive data collection methods and tools create new understanding about the relationship between observable behaviors, searcher context, and underlying cognition, to better support people's information seeking. Yet aside from the problems of data size, realism, and demographics, laboratory studies are limited in the number and nature of phenomena they can study. Hence, data collected in laboratories contains different searcher populations and collects non-overlapping user and task characteristics. While research analyses and collection methods are isolated, how can we further IIR's mission of broad understanding? We approach this as a structure learning problem on incomplete data, determining the extent to which incomplete data can be used to predict user and task characteristics from interactions. In particular, we examine whether combining heterogeneous data sets is more effective than using a single data set alone in prediction. Our results indicate that adding external data significantly improves predictions of searcher characteristics, task characteristics, and behaviors, even when the data does not contain identical information about searchers.
更多查看译文
关键词
bayesian networks, interactive information retrieval, searcher behavior, structure learning, task classification, task prediction, task type
AI 理解论文
溯源树
样例
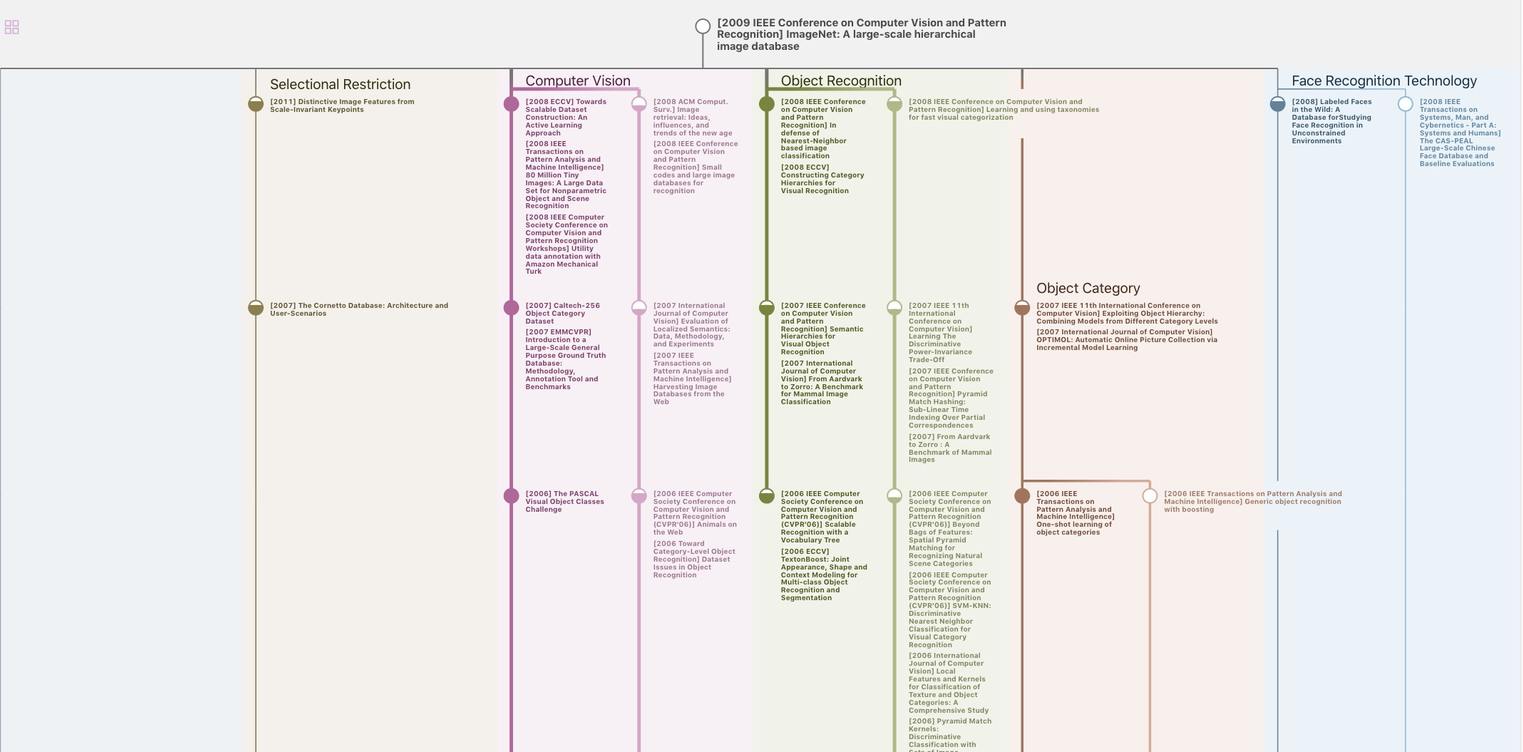
生成溯源树,研究论文发展脉络
Chat Paper
正在生成论文摘要