Fully End-to-End Composite Recurrent Convolution Network for Deformable Facial Tracking in the Wild
FG(2019)
摘要
Human facial tracking is an important task in computer vision, which has recently lost pace compared to other facial analysis tasks. The majority of current available tracker possess two major limitations: their little use of temporal information and the widespread use of handcrafted features, without taking full advantage of the large annotated datasets that have recently become available. In this paper we present a fully end-to-end facial tracking model based on current state of the art deep model architectures that can be effectively trained from the available annotated facial landmark datasets. We build our model from the recently introduced general object tracker Re 3 , which allows modeling the short and long temporal dependency between frames by means of its internal Long Short Term Memory (LSTM) layers. Facial tracking experiments on the challenging 300-VW dataset show that our model can produce state of the art accuracy and far lower failure rates than competing approaches. We specifically compare the performance of our approach modified to work in tracking-by-detection mode and showed that, as such, it can produce results that are comparable to state of the art trackers. However, upon activation of our tracking mechanism, the results improve significantly, confirming the advantage of taking into account temporal dependencies.
更多查看译文
关键词
tracking-by-detection mode,computer vision,end-to-end facial tracking model,deep model architectures,facial landmark datasets,human deformable facial tracking,internal long short term memory layers,fully end-to-end composite recurrent convolution network,training,LSTM layers,object tracker Re3
AI 理解论文
溯源树
样例
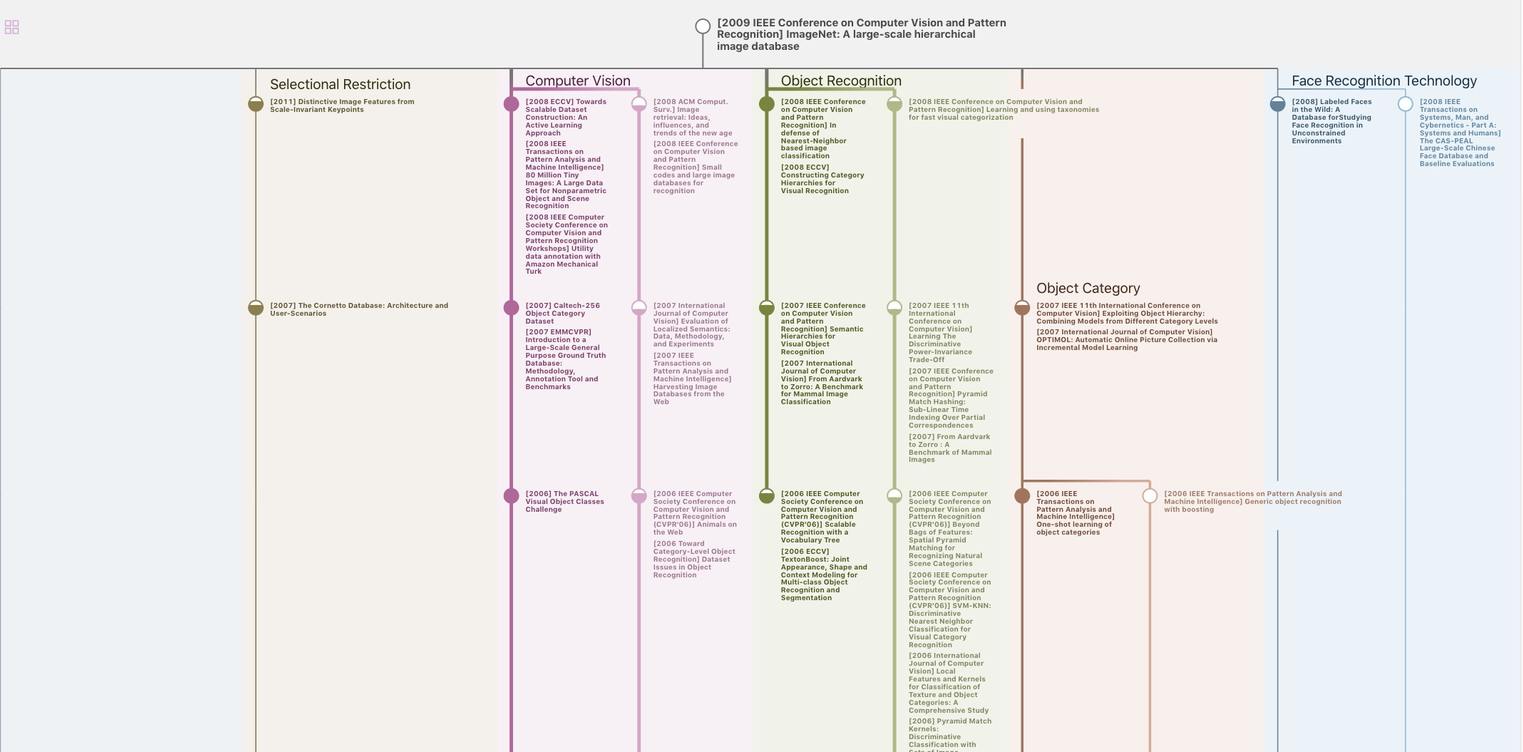
生成溯源树,研究论文发展脉络
Chat Paper
正在生成论文摘要