Improved Two-Dimensional Quaternion Principal Component Analysis
IEEE ACCESS(2019)
摘要
The two-dimensional quaternion principal component analysis (2D-QPCA) is first improved into abstracting the features of quaternion matrix samples in both row and column directions, being the generalization ability, and with the components weighted by corresponding eigenvalues. The main components of the 2D-QPCA in row and column directions are defined as the solution of one optimal problem with orthogonality constraints on two variables. In advance, 2D-QPCA is innovatively armed with the generalization ability by applying the label information (if known) to generate the weight of each class of training set. With such generalization ability, 2D-QPCA may enlarge the variance of all projections of known and unknown samples. Different from the well-known PCA-like methods, the improved 2D-QPCA prefers to the components that have larger variances of projected samples and weight them with larger factors. The improved versions of 2D-QPCA are applied to the color face recognition and image reconstruction. The numerical results based on the real face data sets validate that the newly proposed method performs better than the state-of-the-art ones.
更多查看译文
关键词
2D-QPCA, quaternion matrix, generalization ability, color face recognition, image reconstruction, eigenvalue problem
AI 理解论文
溯源树
样例
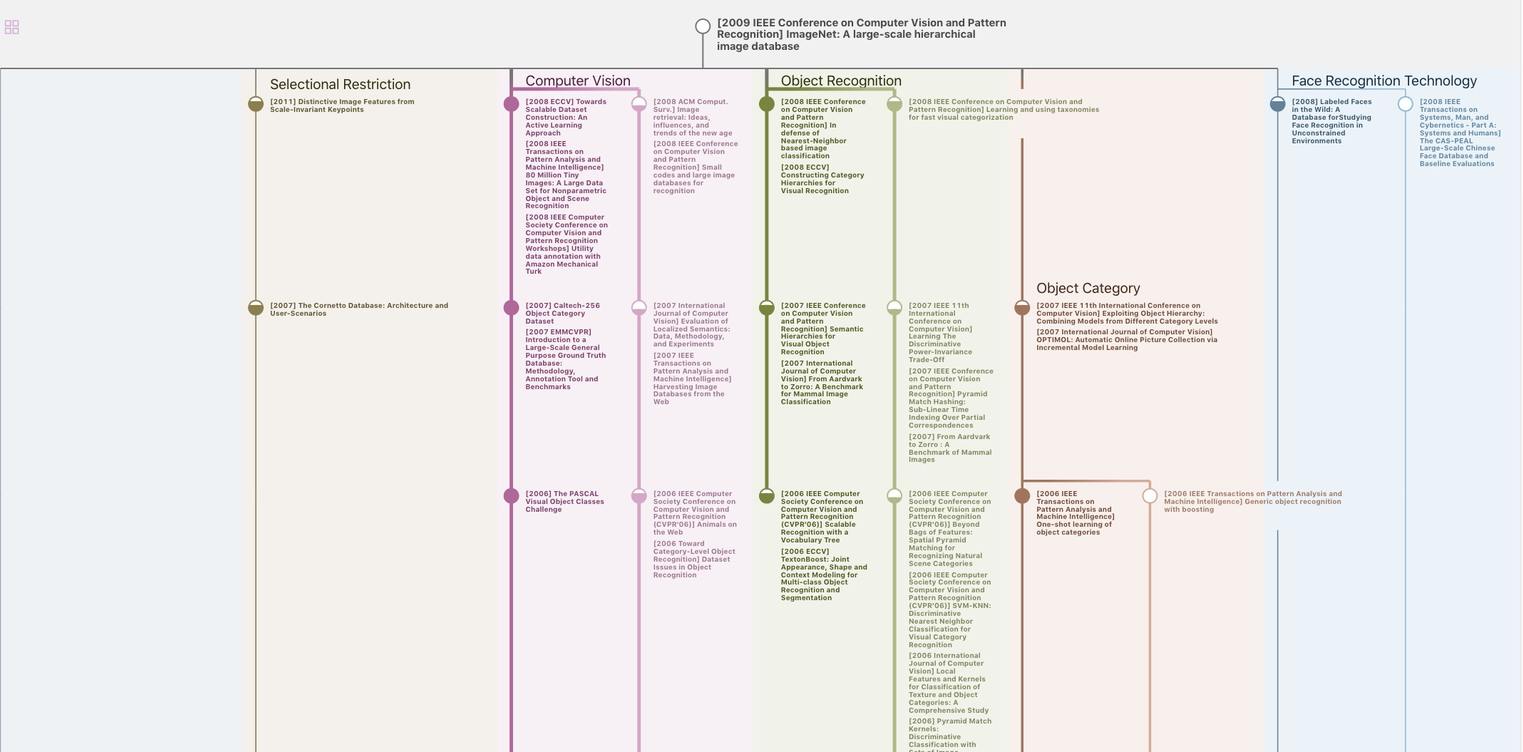
生成溯源树,研究论文发展脉络
Chat Paper
正在生成论文摘要