A Novel Stacked Denoising Autoencoder-Based Reconstruction Framework For Cerenkov Luminescence Tomography
IEEE ACCESS(2019)
摘要
Cerenkov luminescence tomography (CLT) is a promising imaging modality in the field of optical molecular imaging (OMI), which successfully bridges the OMI and tradition nuclear medical imaging and provides the location and quantitative analysis of the distribution of radionuclide probes inside the biological objects. As the CLT is an inherent highly ill-posed inverse problem, it is still a challenge to obtain an accurate reconstruction result. Here, we proposed a novel reconstruction framework based on stacking denoising autoencoders (SDAE), which serve as one famous structure of the artificial neural network (ANN). In our framework, the initial permission region is the whole domain and then a traditional reconstruction algorithm is used to reconstruct each node's energy. Then these nodes are clustered into two regions: permission region and non-permission region, and the permission region is used to start a new reconstruction loop where a new result can be obtained. The procedures above are repeated before the result meets the stop conditions. The numerical simulation experiments, physical phantom experiments and in vivo experiments are all carried out to validate the feasibility and potential of our framework. Results demonstrate that the proposed framework can indeed achieve a good performance in CLT reconstruction.
更多查看译文
关键词
Cerenkov luminescence tomography, optical molecular imaging, reconstruction algorithm, stacking denoising autoencoders
AI 理解论文
溯源树
样例
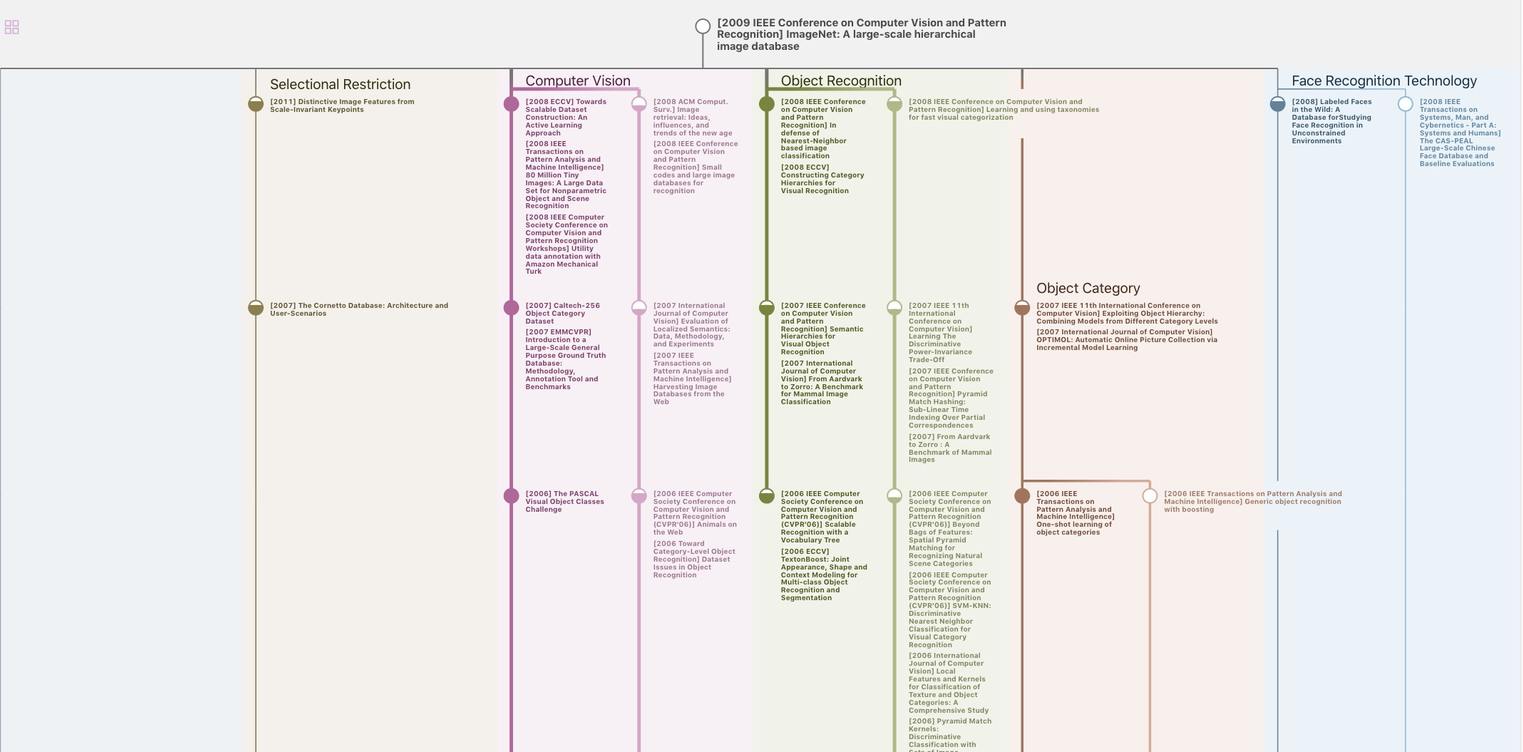
生成溯源树,研究论文发展脉络
Chat Paper
正在生成论文摘要